Particle-flow based tau identification at future $\textrm{e}^{+}\textrm{e}^{-}$ colliders
arXiv (Cornell University)(2023)
摘要
Different algorithms for identifying hadronic $\tau$ decays ($\tau_{\textrm{h}}$) at future high-energy $\textrm{e}^{+}\textrm{e}^{-}$ colliders are studied and compared in terms of their performance. The algorithms studied include the "hadrons-plus-strips" and DeepTau algorithms developed by the CMS collaboration for $\textrm{p}\textrm{p}$ collisions at the LHC and two novel neural network based algorithms, LorentzNet and ParticleTransformer, which have originally been developed for jet flavor tagging and are repurposed for the task of $\tau_{\textrm{h}}$ identification. The algorithms use particles reconstructed by the particle-flow method as input. The best performing algorithm achieves an average misidentification rate for quark and gluon jets of $4.0\cdot10^{-5}$ for an average hadronic $\tau$ identification efficiency of $60\%$. This performance provides very promising prospects for physics analyses involving hadronic $\tau$ decays at future high-energy $\textrm{e}^{+}\textrm{e}^{-}$ colliders.
更多查看译文
关键词
tau identification,particle-flow
AI 理解论文
溯源树
样例
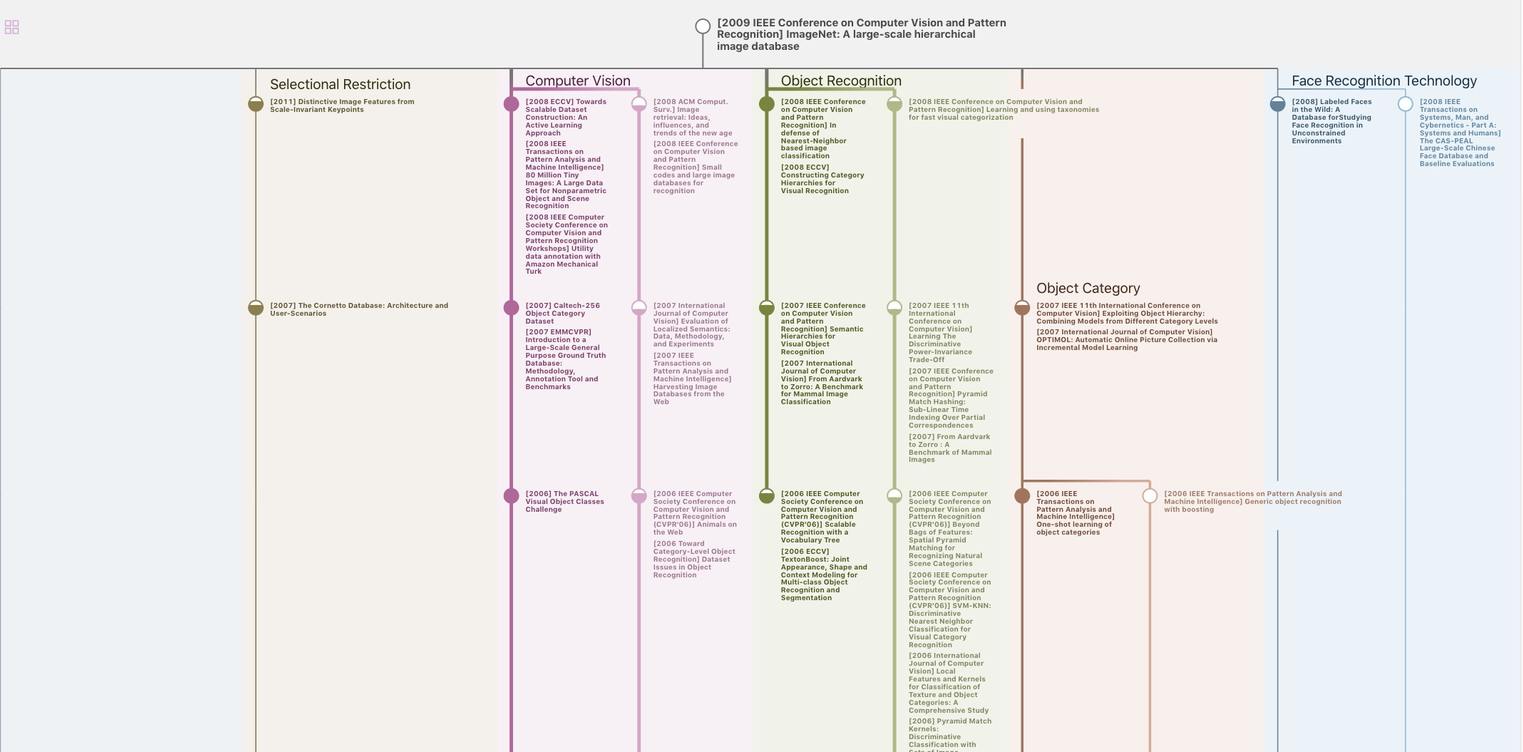
生成溯源树,研究论文发展脉络
Chat Paper
正在生成论文摘要