Pd01-01 enhanced kinematics and multi-modality learning to improve automation of suturing skills assessment
The Journal of Urology(2023)
摘要
You have accessJournal of UrologyCME1 Apr 2023PD01-01 ENHANCED KINEMATICS AND MULTI-MODALITY LEARNING TO IMPROVE AUTOMATION OF SUTURING SKILLS ASSESSMENT Loc Trinh, Timothy N. Chu, Ahmed Ghazi, John W. Davis, Brian J. Miles, Clayton Lau, Anand Malpani, Yan Liu, and Andrew J. Hung Loc TrinhLoc Trinh More articles by this author , Timothy N. ChuTimothy N. Chu More articles by this author , Ahmed GhaziAhmed Ghazi More articles by this author , John W. DavisJohn W. Davis More articles by this author , Brian J. MilesBrian J. Miles More articles by this author , Clayton LauClayton Lau More articles by this author , Anand MalpaniAnand Malpani More articles by this author , Yan LiuYan Liu More articles by this author , and Andrew J. HungAndrew J. Hung More articles by this author View All Author Informationhttps://doi.org/10.1097/JU.0000000000003218.01AboutPDF ToolsAdd to favoritesDownload CitationsTrack CitationsPermissionsReprints ShareFacebookLinked InTwitterEmail Abstract INTRODUCTION AND OBJECTIVE: Previous work have shown that surgeons’ technical skill scores are strong predictors of urinary continence recovery following robot-assisted radical prostatectomy (RARP). Computer vision techniques can help automate the evaluation of suturing skills. In this study, we capture enhanced kinematics (granular motion tracking) of surgical instruments and leverage complementary information between videos and instrument kinematics to improve the performance of automated skill assessments. METHODS: 43 surgeons across 5 institutions completed 5 VR exercises on the Surgical Science™ Flex VR simulator. Instrument kinematic data were captured at 30 Hz, including up to 70 features (XYZ + rotations of each instrument, needle, target, and camera). Videos were segmented into three sub-stitch phases (Needle Handling, Driving, and Withdrawal) and labeled by 3 human raters after standardized training with a validated suturing assessment method. The learning framework extracted features from RGB color and optical flow frames (video) using a pre-trained convolutional network (Alexnet). Visual features are concatenated to kinematic features and encoded using a Transformer encoder. The self-attention mechanism within the Transformer correlated information across both modality (video + kinematic) for all timesteps (Figure 1a). RESULTS: This study included 35 expert surgeons (median caseload 362 [IQR 250-1250]) and 8 training surgeon 64 [ 25-75]) with a total 3448 virtual sutures captured and labelled. Enhanced kinematics models outperformed basic kinematics from previous studies across all domains (+1-12% improvement in AUC; Figure 1b). Video models outperformed enhanced kinematics model on two sub-skills (Needle Hold Angle and Driving Smoothness), demonstrating the usefulness of videos. Multi-modal learning with video and kinematic data in a Transformer encoder yields the best performance for the key sub-skills of Needle driving and Needle withdrawal domains (+1-5.5% improvement in AUC; Figure 1b). CONCLUSIONS: This study demonstrated the best performance of automated assessment of suturing technical skills to date. Enhanced kinematics information and advanced multi-modal learning techniques can improve automated assessment and provide accurate feedback to training surgeons. Source of Funding: Research reported in this publication was supported by the National Cancer Institute of the National Institutes of Health under Award Number R01CA251579. The content is solely the responsibility of the authors and does not necessarily represent the official views of the National Institutes of Health © 2023 by American Urological Association Education and Research, Inc.FiguresReferencesRelatedDetails Volume 209Issue Supplement 4April 2023Page: e62 Advertisement Copyright & Permissions© 2023 by American Urological Association Education and Research, Inc.MetricsAuthor Information Loc Trinh More articles by this author Timothy N. Chu More articles by this author Ahmed Ghazi More articles by this author John W. Davis More articles by this author Brian J. Miles More articles by this author Clayton Lau More articles by this author Anand Malpani More articles by this author Yan Liu More articles by this author Andrew J. Hung More articles by this author Expand All Advertisement PDF downloadLoading ...
更多查看译文
关键词
enhanced kinematics,skills assessment,multi-modality
AI 理解论文
溯源树
样例
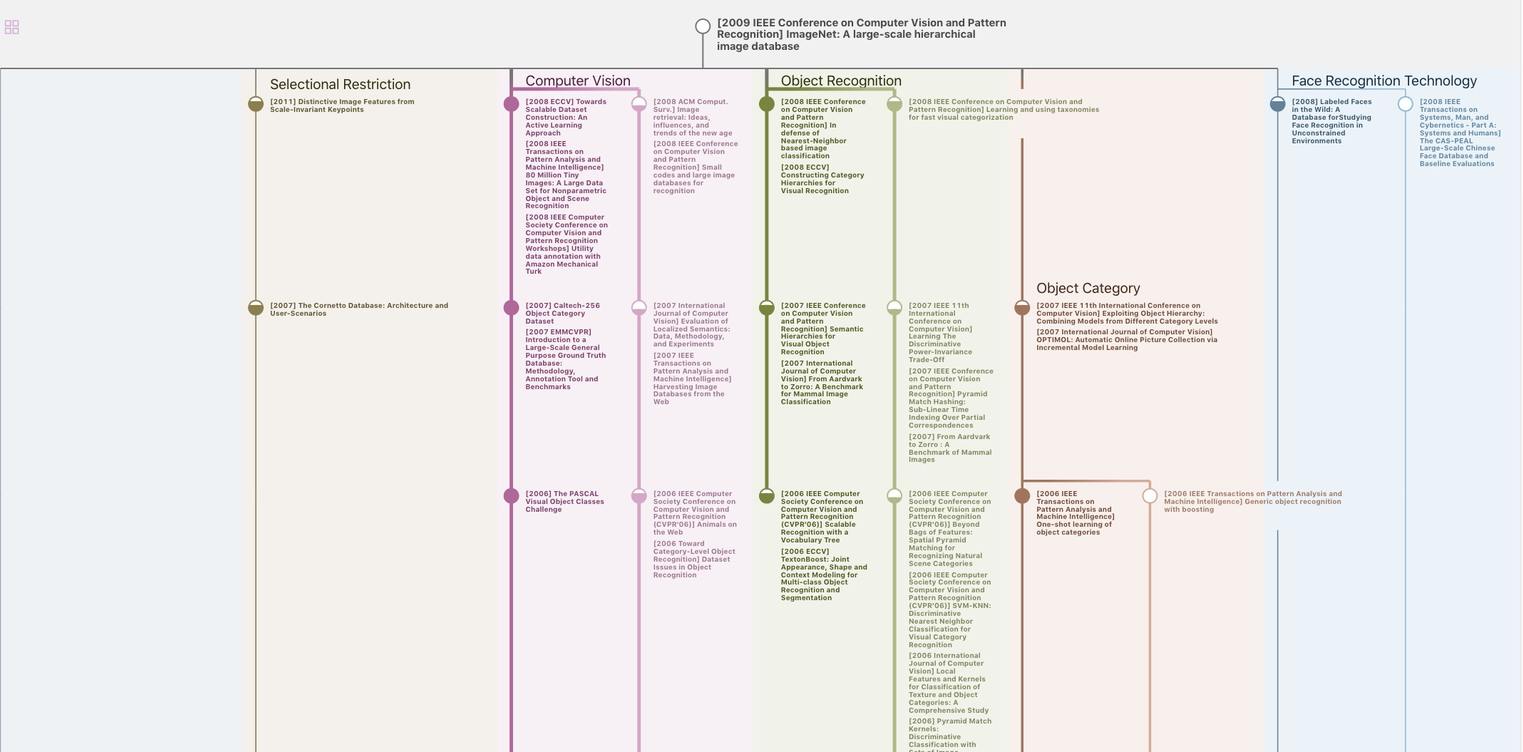
生成溯源树,研究论文发展脉络
Chat Paper
正在生成论文摘要