Deep learning augmented detection of flat lesions on white light cystoscopy
The Journal of Urology(2023)
摘要
You have accessJournal of UrologyCME1 Apr 2023PD01-12 DEEP LEARNING AUGMENTED DETECTION OF FLAT LESIONS ON WHITE LIGHT CYSTOSCOPY Eugene Shkolyar, Xiao Jia, Okyaz Eminaga, Mark Laurie, Zixia Zhou, Tauhidul Islam, Lei Xing, and Joseph Liao Eugene ShkolyarEugene Shkolyar More articles by this author , Xiao JiaXiao Jia More articles by this author , Okyaz EminagaOkyaz Eminaga More articles by this author , Mark LaurieMark Laurie More articles by this author , Zixia ZhouZixia Zhou More articles by this author , Tauhidul IslamTauhidul Islam More articles by this author , Lei XingLei Xing More articles by this author , and Joseph LiaoJoseph Liao More articles by this author View All Author Informationhttps://doi.org/10.1097/JU.0000000000003218.12AboutPDF ToolsAdd to favoritesDownload CitationsTrack CitationsPermissionsReprints ShareFacebookLinked InTwitterEmail Abstract INTRODUCTION AND OBJECTIVE: While-light cystoscopy (WLC) has inadequate performance for detection of flat lesions such as carcinoma in situ. Blue-light cystoscopy (BLC) has improved flat-lesion detection, however adoption is modest due to equipment cost, drug instillation, and user experience. Here we develop a non-invasive, deep learning-based algorithm to augment WLC flat lesion detection by using blue-light informed transfer learning. METHODS: CystoFlatNet was developed based on CystoNet, a deep learning platform for WLC bladder cancer detection. CystoNet was enhanced to augment flat lesion detection in two stages. First, a WLC image was input into a BLC domain using domain adaption of CycleGAN. Second, a region of interest (ROI) detector was used to segment the regions within the transferred images occupied by a flat lesion. Transfer learning was applied to embed features learned from both WLC and BLC into the model weights. Algorithm outputs included an outline deployed in WLC and a “mock” BLC output. For algorithm development, images of WLC and BLC matches from 50 subjects with flat lesions were selected and annotated. A training set consisting of 40 subjects and a test set of 10 subjects were used. Training was performed on WLC and BLC images. WLC was the only input used for validation. Per-lesion sensitivity was determined and performance compared to CystoNet. RESULTS: CystoFlatNet detected flat lesions on WLC with a 90% per-lesion sensitivity (9/10). In comparison, the previously trained CystoNet had a 30% per-lesion sensitivity (3/10). Large accuracy gains were obtained in ROI overlap and visual guidance in the blue-light domain (Figure 1). CONCLUSIONS: We have developed a deep-learning algorithm that shows promise in WLC flat lesion detection. CystoFlatNet may aid in diagnostic decision-making and could serve as a non-invasive, accessible alternative to available adjunct technologies. Validation in a large prospective cohort is needed to further evaluate CystoFlatNet performance. Source of Funding: Department of Veterans Affairs Merit Review Award I01 BX005598, Urology Care Foundation Research Scholar Award © 2023 by American Urological Association Education and Research, Inc.FiguresReferencesRelatedDetails Volume 209Issue Supplement 4April 2023Page: e68 Advertisement Copyright & Permissions© 2023 by American Urological Association Education and Research, Inc.MetricsAuthor Information Eugene Shkolyar More articles by this author Xiao Jia More articles by this author Okyaz Eminaga More articles by this author Mark Laurie More articles by this author Zixia Zhou More articles by this author Tauhidul Islam More articles by this author Lei Xing More articles by this author Joseph Liao More articles by this author Expand All Advertisement PDF downloadLoading ...
更多查看译文
关键词
deep learning augmented detection,flat lesions,deep learning
AI 理解论文
溯源树
样例
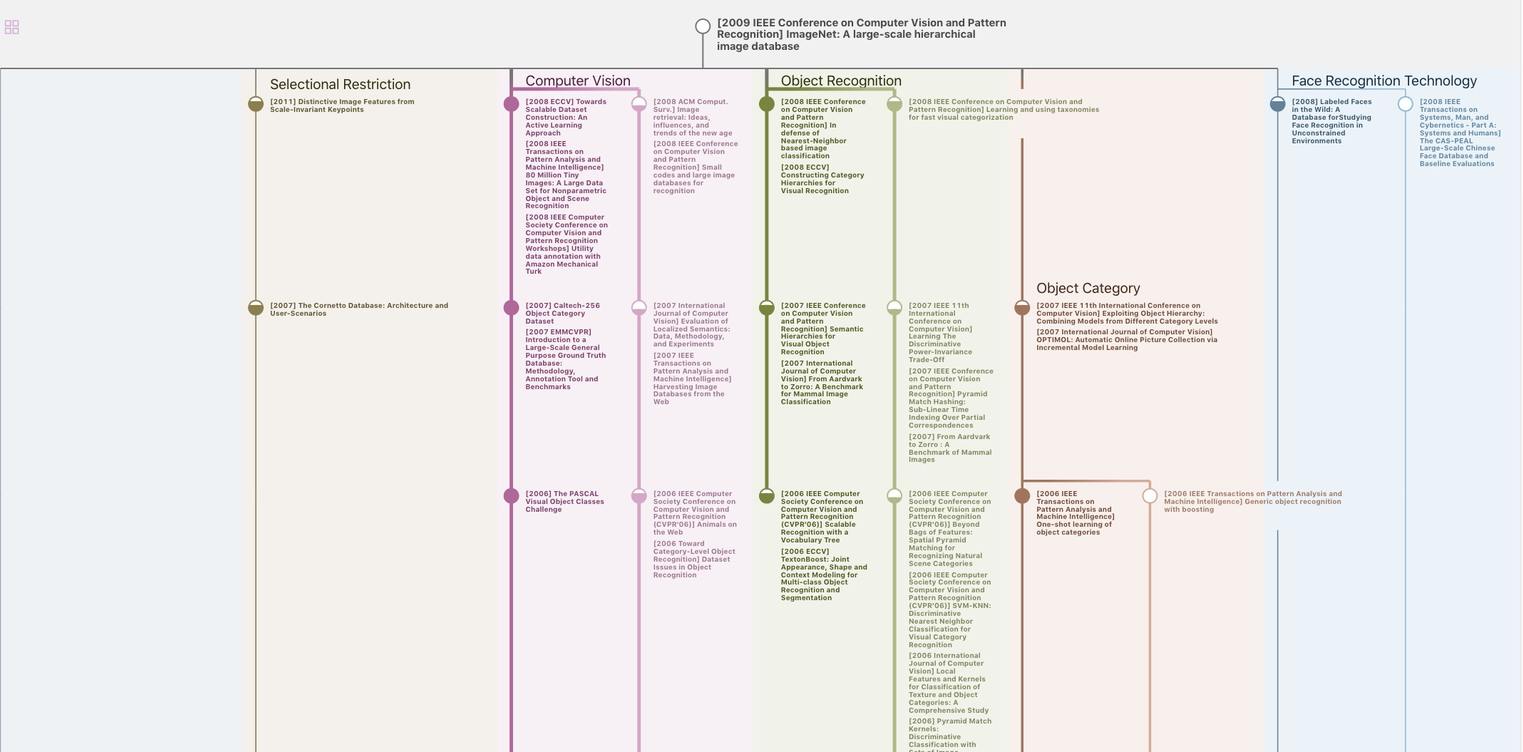
生成溯源树,研究论文发展脉络
Chat Paper
正在生成论文摘要