SynTable: A Synthetic Data Generation Pipeline for Unseen Object Amodal Instance Segmentation of Cluttered Tabletop Scenes
CoRR(2023)
摘要
In this work, we present SynTable, a unified and flexible Python-based
dataset generator built using NVIDIA's Isaac Sim Replicator Composer for
generating high-quality synthetic datasets for unseen object amodal instance
segmentation of cluttered tabletop scenes. Our dataset generation tool can
render a complex 3D scene containing object meshes, materials, textures,
lighting, and backgrounds. Metadata, such as modal and amodal instance
segmentation masks, occlusion masks, depth maps, bounding boxes, and material
properties, can be generated to automatically annotate the scene according to
the users' requirements. Our tool eliminates the need for manual labeling in
the dataset generation process while ensuring the quality and accuracy of the
dataset. In this work, we discuss our design goals, framework architecture, and
the performance of our tool. We demonstrate the use of a sample dataset
generated using SynTable by ray tracing for training a state-of-the-art model,
UOAIS-Net. The results show significantly improved performance in Sim-to-Real
transfer when evaluated on the OSD-Amodal dataset. We offer this tool as an
open-source, easy-to-use, photorealistic dataset generator for advancing
research in deep learning and synthetic data generation.
更多查看译文
关键词
instance segmentation,unseen object amodal
AI 理解论文
溯源树
样例
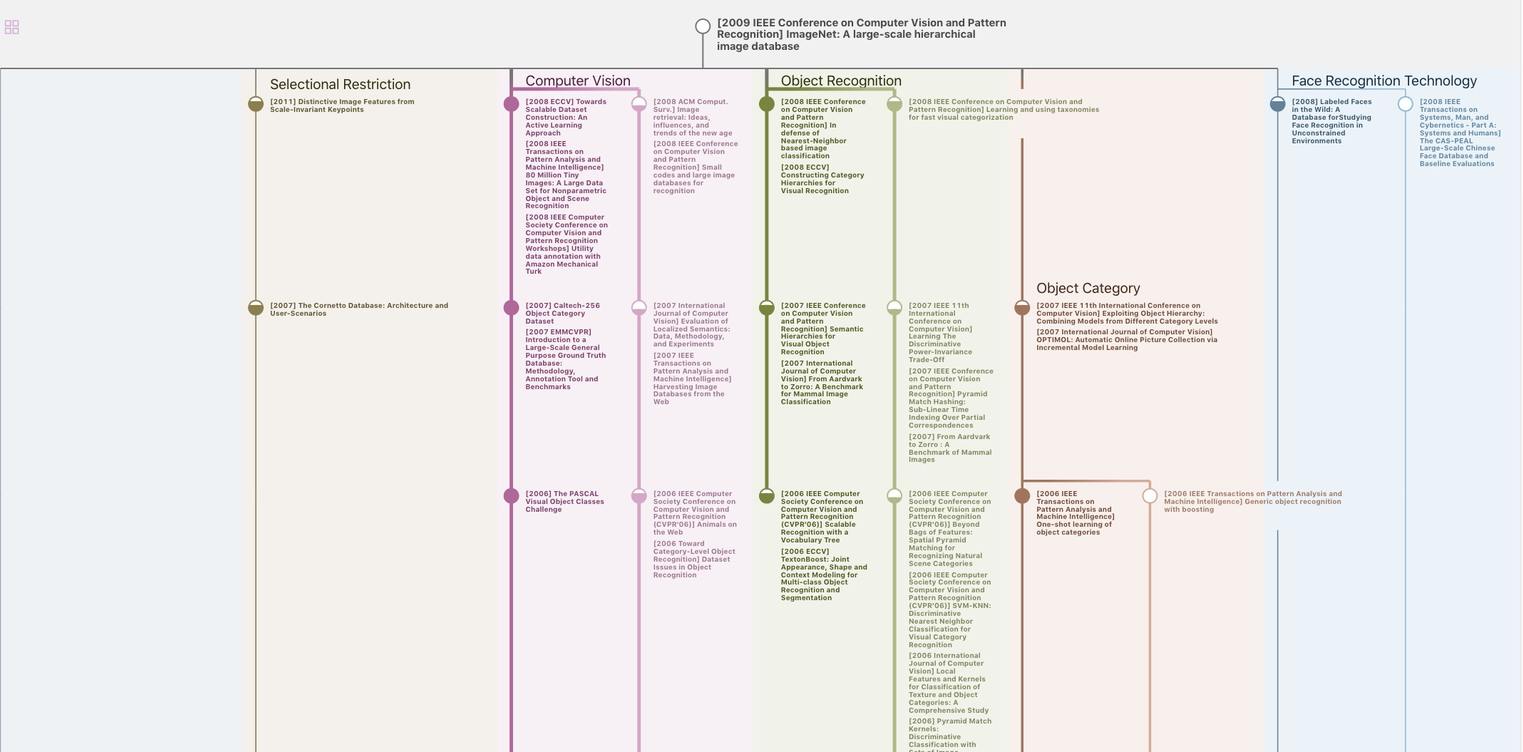
生成溯源树,研究论文发展脉络
Chat Paper
正在生成论文摘要