DISPEL: Domain Generalization via Domain-Specific Liberating
CoRR(2023)
摘要
Domain generalization aims to learn a generalization model that can perform well on unseen test domains by only training on limited source domains. However, existing domain generalization approaches often bring in prediction-irrelevant noise or require the collection of domain labels. To address these challenges, we consider the domain generalization problem from a different perspective by categorizing underlying feature groups into domain-shared and domain-specific features. Nevertheless, the domain-specific features are difficult to be identified and distinguished from the input data. In this work, we propose DomaIn-SPEcific Liberating (DISPEL), a post-processing fine-grained masking approach that can filter out undefined and indistinguishable domain-specific features in the embedding space. Specifically, DISPEL utilizes a mask generator that produces a unique mask for each input data to filter domain-specific features. The DISPEL framework is highly flexible to be applied to any fine-tuned models. We derive a generalization error bound to guarantee the generalization performance by optimizing a designed objective loss. The experimental results on five benchmarks demonstrate DISPEL outperforms existing methods and can further generalize various algorithms.
更多查看译文
关键词
domain generalization,domain-specific
AI 理解论文
溯源树
样例
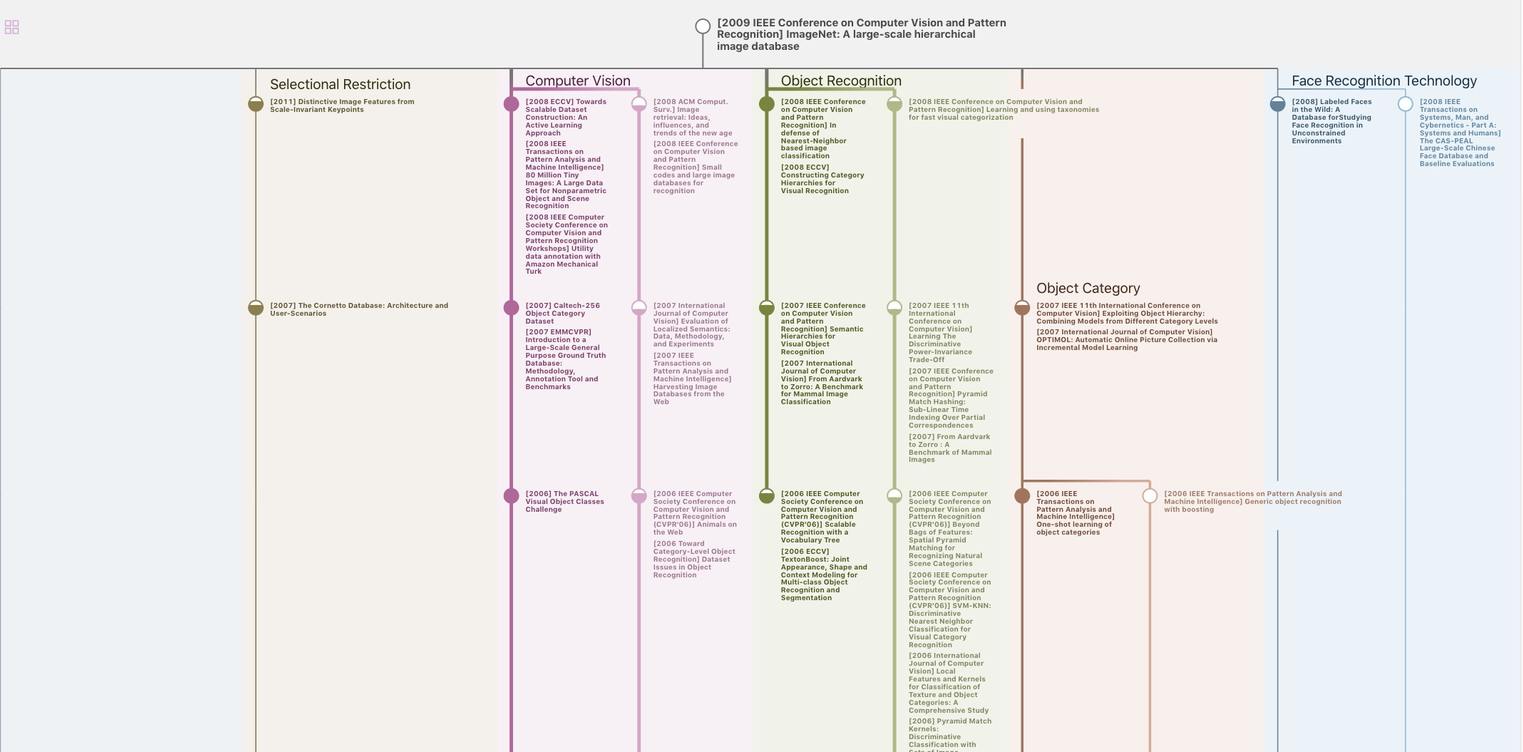
生成溯源树,研究论文发展脉络
Chat Paper
正在生成论文摘要