Bootstrapping Vision-Language Learning with Decoupled Language Pre-training
NeurIPS(2023)
摘要
We present a novel methodology aimed at optimizing the application of frozen large language models (LLMs) for resource-intensive vision-language (VL) pre-training. The current paradigm uses visual features as prompts to guide language models, with a focus on determining the most relevant visual features for corresponding text. Our approach diverges by concentrating on the language component, specifically identifying the optimal prompts to align with visual features. We introduce the Prompt-Transformer (P-Former), a model that predicts these ideal prompts, which is trained exclusively on linguistic data, bypassing the need for image-text pairings. This strategy subtly bifurcates the end-to-end VL training process into an additional, separate stage. Our experiments reveal that our framework significantly enhances the performance of a robust image-to-text baseline (BLIP-2), and effectively narrows the performance gap between models trained with either 4M or 129M image-text pairs. Importantly, our framework is modality-agnostic and flexible in terms of architectural design, as validated by its successful application in a video learning task using varied base modules. The code is available at https://github.com/yiren-jian/BLIText
更多查看译文
关键词
decoupled vision-language,learning,pre-training
AI 理解论文
溯源树
样例
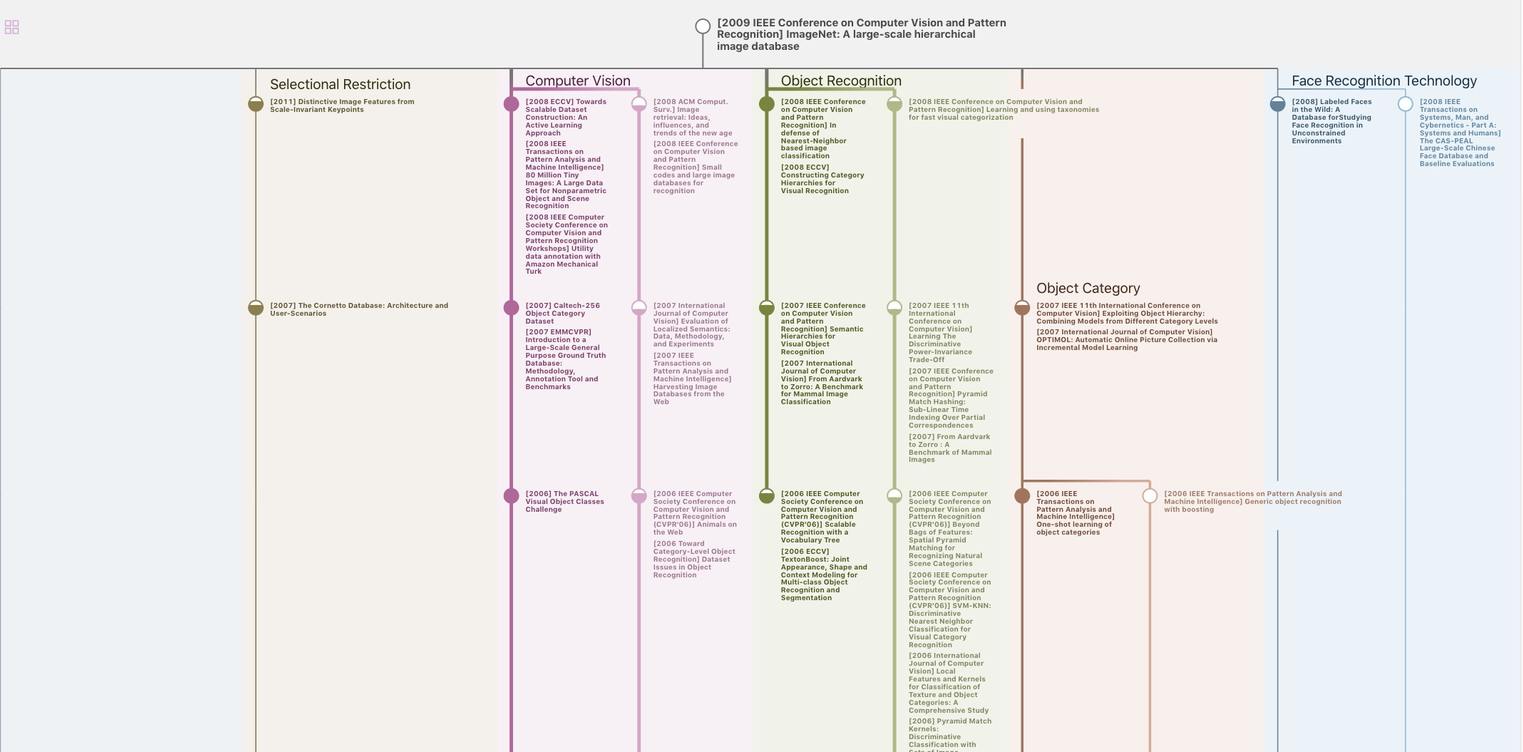
生成溯源树,研究论文发展脉络
Chat Paper
正在生成论文摘要