Predictive Visual Analytics for Machine Learning Model in House Price Prediction: A Case Study
Open International Journal of Informatics(2021)
摘要
As an individual, buying a house is a nerve-racking process. It requires a huge amount of money, time-consuming and relentless worry whether it is a good deal or not. The uncertainty in the housing market and the motivation to own a house have raised questions among homeowners and buyers regarding how accurate the house prices can be predicted, and what attributes or factors influenced the house prices. There were studies conducted in Malaysia that applied machine learning in predicting house prices. However, most of the studies using the Valuation and Property Service Department (VPSD) dataset were conducted in different states, namely Selangor, Kuala Lumpur, and Johor. Thus, there is an opportunity to extend the study to predict the house price in Penang state, Malaysia due to the increase in house prices in Penang is the highest among all the states in Malaysia. Therefore, this study aims to produce a machine learning predictive model using 2,666 terrace houses actual property transactions in Penang from VPSD from January 2018 until December 2019. The dataset is split into a train-test (estimation-validation) set with 80% train set and 20% test set (80:20) proportion and separated by two groups of different feature selection dataset which is all feature and selected features. Hence, to capture the different performances from both groups. The predictive model development using Multiple Linear Regression, Random Forest, and K-Nearest Neighbors algorithms with different parameters. The predictive model's performance was evaluated based on error measurement metrics such as Mean Absolute Error (MAE), Root Mean Square Error (RMSE), and Mean Absolute Percentage Error (MAPE). Its reveals that Random Forest of 250 trees using all feature has been chosen as the best model among others which produces 23,786.856 for Root Mean Square Error (RMSE), 13,769.965 for Mean Absolute Error (MAE), and 4.674% Mean Absolute Percentage Error (MAPE) from the train set.
更多查看译文
关键词
predictive visual analytics,house price prediction,machine learning,machine learning model
AI 理解论文
溯源树
样例
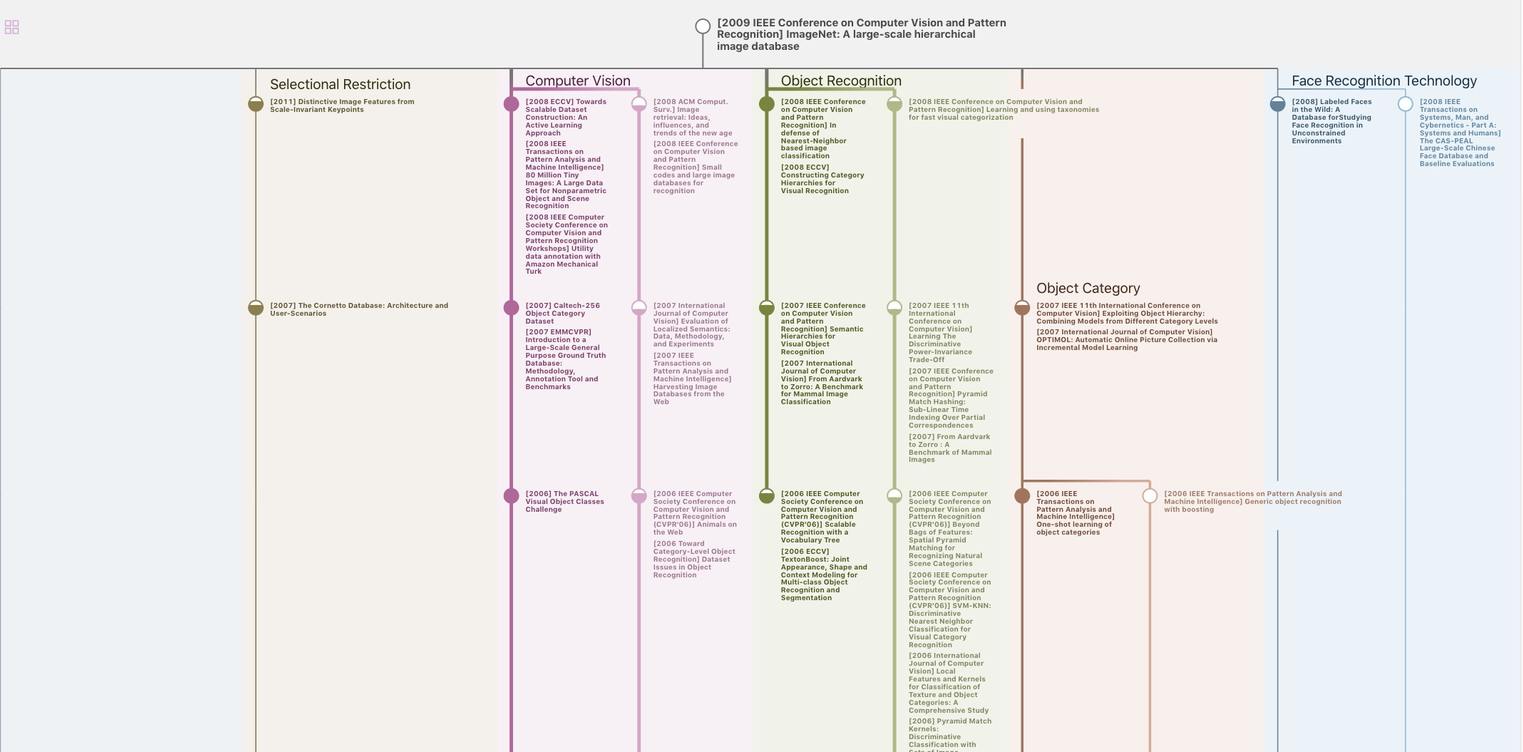
生成溯源树,研究论文发展脉络
Chat Paper
正在生成论文摘要