Evaluations of statistical methods for outlier detection when benchmarking in clinical registries: a systematic review
BMJ open(2023)
摘要
Objectives Benchmarking is common in clinical registries to support the improvement of health outcomes by identifying underperforming clinician or health service providers. Despite the rise in clinical registries and interest in publicly reporting benchmarking results, appropriate methods for benchmarking and outlier detection within clinical registries are not well established, and the current application of methods is inconsistent. The aim of this review was to determine the current statistical methods of outlier detection that have been evaluated in the context of clinical registry benchmarking. Design A systematic search for studies evaluating the performance of methods to detect outliers when benchmarking in clinical registries was conducted in five databases: EMBASE, ProQuest, Scopus, Web of Science and Google Scholar. A modified healthcare modelling evaluation tool was used to assess quality; data extracted from each study were summarised and presented in a narrative synthesis. Results Nineteen studies evaluating a variety of statistical methods in 20 clinical registries were included. The majority of studies conducted application studies comparing outliers without statistical performance assessment (79%), while only few studies used simulations to conduct more rigorous evaluations (21%). A common comparison was between random effects and fixed effects regression, which provided mixed results. Registry population coverage, provider case volume minimum and missing data handling were all poorly reported. Conclusions The optimal methods for detecting outliers when benchmarking clinical registry data remains unclear, and the use of different models may provide vastly different results. Further research is needed to address the unresolved methodological considerations and evaluate methods across a range of registry conditions. PROSPERO registration number CRD42022296520.
更多查看译文
关键词
outlier detection,clinical registries,statistical methods,systematic review
AI 理解论文
溯源树
样例
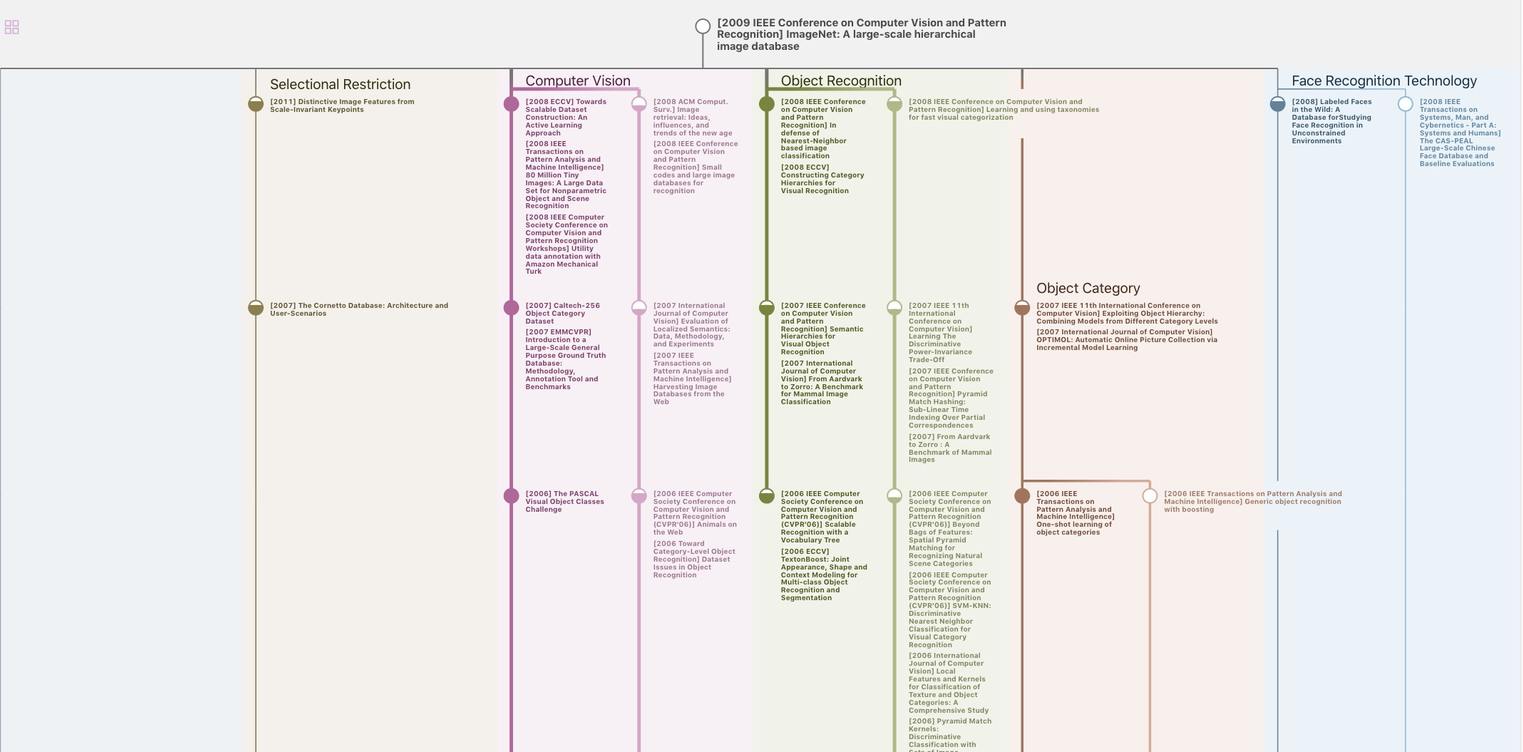
生成溯源树,研究论文发展脉络
Chat Paper
正在生成论文摘要