A novel prediction model for possibility of sperm retrieval in micro tese for non-obstructive azoospermia patients using automated artificial intelligence
The Journal of Urology(2023)
摘要
You have accessJournal of UrologyCME1 Apr 2023MP30-02 A NOVEL PREDICTION MODEL FOR POSSIBILITY OF SPERM RETRIEVAL IN MICRO TESE FOR NON-OBSTRUCTIVE AZOOSPERMIA PATIENTS USING AUTOMATED ARTIFICIAL INTELLIGENCE Hideyuki Kobayashi, Masato Uetani, Koichi Nakajima, and Koichi Nagao Hideyuki KobayashiHideyuki Kobayashi More articles by this author , Masato UetaniMasato Uetani More articles by this author , Koichi NakajimaKoichi Nakajima More articles by this author , and Koichi NagaoKoichi Nagao More articles by this author View All Author Informationhttps://doi.org/10.1097/JU.0000000000003258.02AboutPDF ToolsAdd to favoritesDownload CitationsTrack CitationsPermissionsReprints ShareFacebookLinked InTwitterEmail Abstract INTRODUCTION AND OBJECTIVE: Azoospermia is a severe problem that prevents couples from having their own children through natural pregnancy. In non-obstructive azoospermia (NOA), a particularly severe type of male infertility, microdissection testicular sperm extraction (micro TESE) is required to collect sperm and, at 40-60%, the sperm retrieval success rate is not very high.Previous studies identified no single clinical finding or investigation that could accurately predict the outcome of sperm retrieval. In addition, although clinical and hormone parameters have been previously investigated as potential predictors of sperm retrieval, the evidence for them has been conflicting and a specific biochemical marker predicting the possibility of sperm retrieval has yet to be established. It would be very valuable to know the possibility of sperm retrieval for patients with NOA before performing micro TESE. Therefore, our objective was to make a model for predicting the possibility of sperm retrieval in patients with NOA before performing micro TESE, using machine learning. METHODS: We retrospectively obtained data from the medical records of 430 patients who underwent micro TESE from 2011 to 2020. Parameters extracted were age, height, body weight, body mass index, LH, FSH, FSH, PRL, total testosterone, E2, T/E2, sperm retrieval, G-band, AZF, medical history, Rt testis, and Lt testis. Prediction One, which does not require coding, was used to create an AI prediction model for sperm retrieval. Prediction One makes the best prediction model using an artificial neural network (ANN) with internal cross validation. RESULTS: The mean age of the 430 male patients who underwent micro TESE in this study was 36.78±7.58 years. Sperm was successfully retrieved in 151 (35.1%) patients.Statistically significant differences between successful and unsuccessful sperm retrieval were observed for age, LH, FSH, total testosterone, Rt testis, Lt testis and medical history. Medical history included none, Lt varicocele, cryptorchidism, inguinal hernia, torsion of spermatic cord, orchitis, cancer treatment, spinal injury, spina bifida, and anti-sperm antibody (+). The AUC for the AI model was 72.46%, which is acceptable. The values for Accuracy, Precision and Recall were 72.09%, 64.76% and 45.03%, respectively, when the F-value was 53.13%. CONCLUSIONS: We created an AI model for predicting sperm retrieval in patients with NOA before undergoing micro TESE. Source of Funding: none © 2023 by American Urological Association Education and Research, Inc.FiguresReferencesRelatedDetails Volume 209Issue Supplement 4April 2023Page: e391 Advertisement Copyright & Permissions© 2023 by American Urological Association Education and Research, Inc.MetricsAuthor Information Hideyuki Kobayashi More articles by this author Masato Uetani More articles by this author Koichi Nakajima More articles by this author Koichi Nagao More articles by this author Expand All Advertisement PDF downloadLoading ...
更多查看译文
关键词
sperm retrieval,micro tese,artificial intelligence,automated artificial intelligence,non-obstructive
AI 理解论文
溯源树
样例
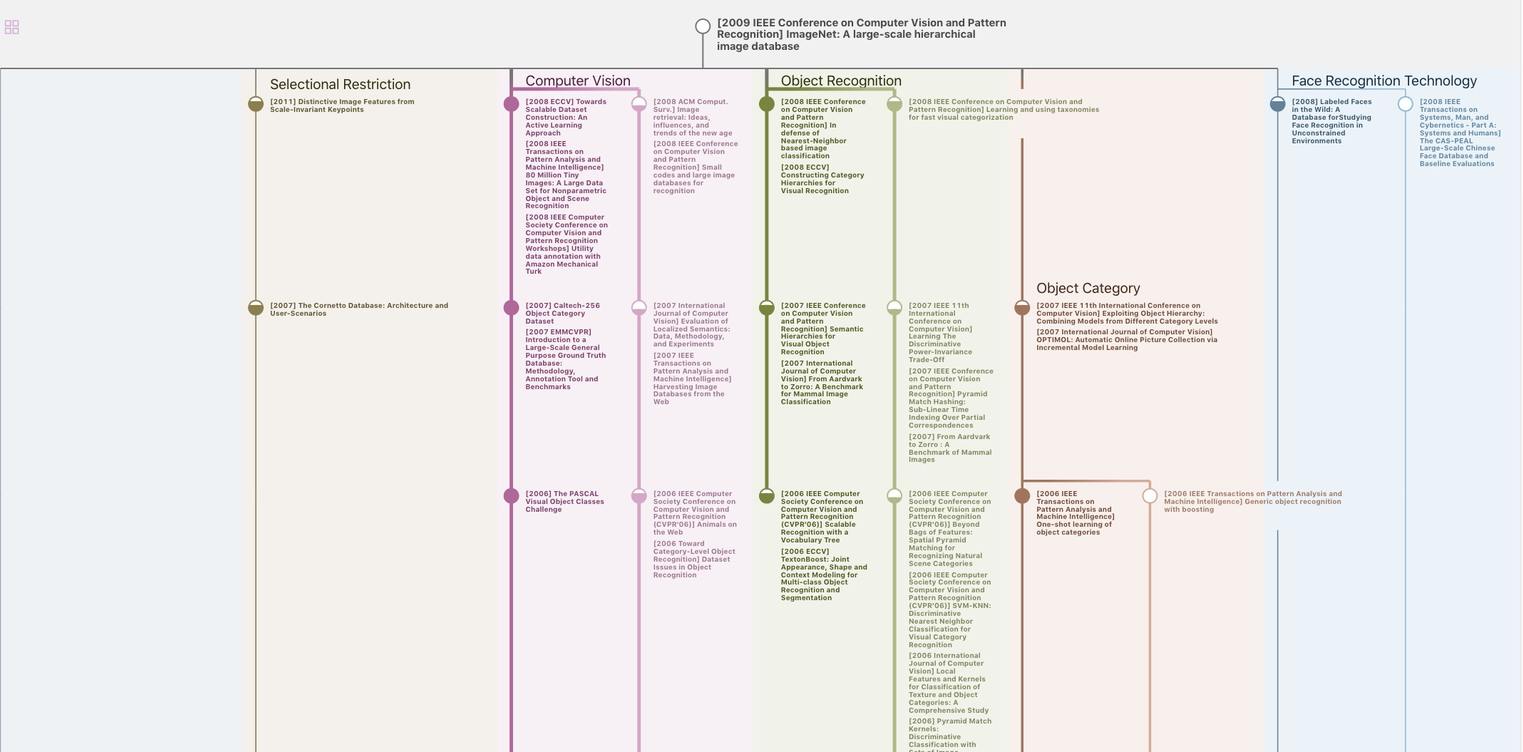
生成溯源树,研究论文发展脉络
Chat Paper
正在生成论文摘要