Model-Free Adaptive Iterative Learning From Communicable Agents for Nonlinear Networks Consensus
IEEE Transactions on Signal and Information Processing over Networks(2023)
摘要
This work reconsiders the consensus tracking issue from a new viewpoint of learning from communicable agents in a strongly connected nonlinear nonaffine multiagent system (MAS). First, we present a communicable-agent-based linear data model (CA-LDM) for describing the input-output (I/O) dynamics between an agent and its neighbors. Meanwhile, an iterative adaptive method is designed to update the CA-LDM by employing I/O data. Then, a communicable-agent-based model-free adaptive iterative learning consensus (CA-MFAILC) scheme is developed by learning the spatial behaviour of MAS and the behaviour of the agent itself. The proposed algorithm does not require the same initial conditions, such that it is easy to be applied to the real applications. Besides, the presented CA-MFAILC does not use the model information, but being a data-driven method. The rigorous analysis along with the simulation study illustrates the effectiveness of the CA-MFAILC.
更多查看译文
关键词
communicable agents,networks,consensus,learning,model-free
AI 理解论文
溯源树
样例
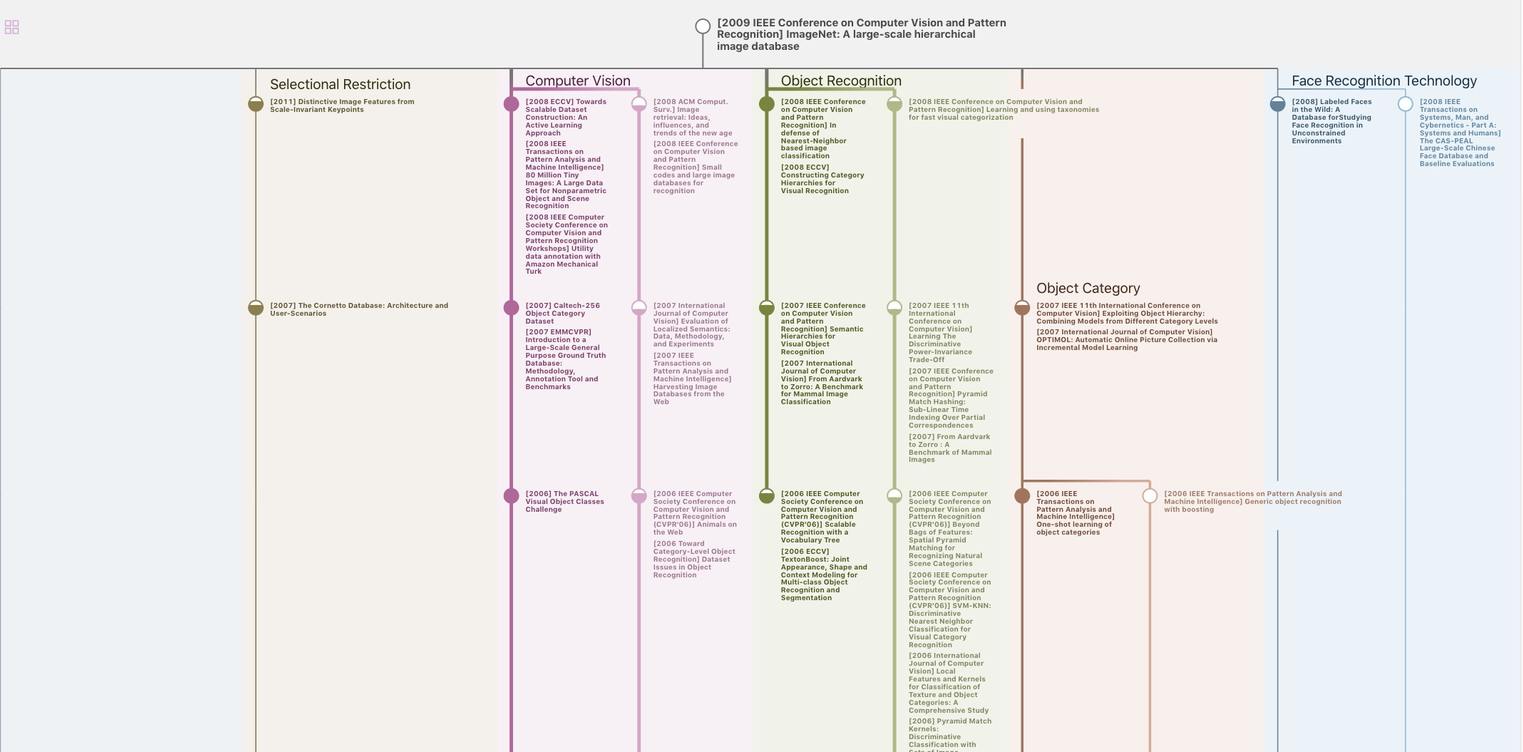
生成溯源树,研究论文发展脉络
Chat Paper
正在生成论文摘要