Speaking truth about power: Are underpowered trials undercutting evaluation of new care models?
JOURNAL OF EVALUATION IN CLINICAL PRACTICE(2024)
摘要
Leading voices have called for expanded use of randomised controlled trials (RCTs) within learning health systems to rigorously evaluate innovative models of health care delivery,1 such as intensive case management interventions. However, intervention trials—especially involving complex health care model interventions—are unlike trials studying drugs and devices because the interventions are often multifaceted and complex. As a result, even well-designed trials can encounter implementation problems such as under recruitment, incomplete patient engagement, or loss to follow up, which reduce statistical power. When implementation issues have disrupted a trial, interpretation of null results is not straightforward: was the intervention truly ineffective, did implementation of the intervention fall short, or did were evaluation analyses simply underpowered? Evaluators favor randomised study designs and intent-to-treat (ITT) analyses because these methods reduce the chance of incorrectly concluding that an ineffective intervention works. The dangers of false-positive results are salient to today's decision makers: propagating ineffective—and costly—interventions would be a failure. However, underpowered trials can produce false-negative results, suggesting an intervention is not effective (when it actually is). False-negative results can lead evaluators to shelve the intervention and not report results,2 which in turn means sponsors' investments yield few lessons learned, implementers become disillusioned about rigorous evaluation, and patients lose access to an effective care model. In the remainder of this commentary, we summarise the concept of statistical power and discuss the features of care model trials that make them especially prone to being underpowered. We then present five strategies that innovators and evaluators can use to avoid power problems or respond when they occur. When most people think about statistical power they think in terms of sample size, but statistical power is more nuanced than that.3 Power depends on five factors: (1) study design (2) effect size, the average effect of the intervention on outcome(s) within the target population; (3) sample size, the number of study participants; (4) variance of the outcome measure; and, (5) the decision rule (or α-level), the threshold for determining statistical significance (p = 0.05 is the traditional choice). Statistical power increases as sample size and effect size increase, as variance decreases, and as the α-level is increased.3 Overreliance on p-values has been criticised4 but decision-making frameworks that account for the relative costs of the different errors5 are rarely adopted in standard evaluation practice. Strategies used to increase statistical power in laboratory and pharma trials are often infeasible or impractical to apply in care-model RCTs. These might include tactics like limiting trials to narrow populations with high anticipated effect size, carrying out recruitment before randomisation, delivering treatment immediately after randomisation, and using simple treatment protocols like ‘pill a day’ interventions. Furthermore, sample sizes in trials of health care innovations are often constrained by practical realities like limited budgets or available clinicians or staff. This makes a randomised design arguably the fairest way to allocate a limited resource, but it can also lead innovators to randomise an insufficient number of participants. This can be compounded when power estimates are inflated by innovators' optimism about the expected effectiveness of the intervention (e.g., overlooking heterogeneity of model effectiveness and mistakenly attributing the anticipated effect size for the optimal participant to the whole sample) or when eligibility drifts are permitted as a means to increase sample size (without recognising that the average effect size in a broadened target population may decrease as a result).6 An additional source of power loss that is particular to RCTs of new care models occurs when trial protocols fail to optimise participation such that a substantial portion of the randomised intervention group does not receive enough (or any) of the intervention (Figure 1). One way this can manifest is if some intervention arm patients cannot be reached or decline trial enrolment. Care model trials, which often leverage secondary data and assign usual care as the control condition, frequently randomise patients before recruiting and enroling the intervention group. This strategy can be appealing because it saves on recruitment time and effort (the control group is never actively recruited). However, randomising before recruitment can result in substantial power losses if a sizeable proportion of the intervention arm cannot be enroled. The alternative is to randomise after recruitment, but from a power perspective this is not failsafe either: as shown in Figure 1, care model trials can also lose statistical power when gaps in intervention delivery curtail implementation fidelity and a portion of the intervention group receives an insufficient dose of the intervention (in time, intensity, or other dimensions that might be relevant for a given theory of change).7 Taken together, small trial populations and overly optimistic power calculations combined with the special challenges of implementing care model RCTs mean that such trials can easily become underpowered. However, there are strategies innovators can use to avoid falling prey to the false-negative conundrum in care model RCTs. Be conservative when estimating effect size for power calculations. Innovators are always optimistic, but over-inflated expectations about average effect size can lead to insufficient statistical power. During trial design, innovators should partner with evaluators to vet the plausibility of assumptions and the underlying theory of change. Patient representatives may also offer valuable insight about the likelihood of achieving the envisioned benefits. One caution is to remember the potential variability in effect estimates. Using a value below the anticipated mean effect size is a prudent hedge that would result in a more conservative estimate of sample needed. Anticipate challenges to intervention uptake and engagement. Optimism about the smooth implementation of trial procedures is also common. Innovators and evaluators should anticipate and minimise sources of sample attrition at all trial stages, and plan for a large enough sample size to yield adequate power even after accounting for potential gaps in patient engagement. Evaluators and implementers should also establish a system for gathering data on reasons for incomplete uptake and engagement, to enable learning not just about whether an intervention works, but also how it could be improved at the next stage. Use preparatory (proof of concept) studies before undertaking full-scale trials. Small pilot trials or evaluability assessments can be used to help avoid underpowered RCTs.8, 9 Organisationally sponsored care model trials within learning health systems are not generally subject to formal study sections and competitive funding processes. Absent these external forces that encourage use of pilot trials, innovators and evaluators should still have the discipline to invest the time and effort required to complete pilot trials as a means of improving the evidence yield from subsequent RCTs. The traditional use of pilot RCTs is feasibility and acceptability, but they can also be valuable for developing realistic estimates of likely average effect size, refining population criteria, selecting outcome measures, and establishing high-yield enrollment, engagement, and retention strategies. Temper interpretation of null results. Statistics 101 teaches that one must never accept the null hypothesis, but in practice this is often the outcome when a trial yields a null result. Whether or not the results of a null trial are to be submitted for publication, all trial stakeholders should think carefully about what it means when study results are not statistically significant and interrogate whether power problems may have undercut the study's ability to detect an intervention effect. This is not an argument to propagate interventions that failed the statistical test. Neither is it an invitation to turn to per protocol analyses (where treated patients are compared to the full control group) or other less rigorous evaluation approaches, as these are potentially highly biased. Rather, it is a call to more systematically engage in planned study of trial implementation to understand whether issues in trial design and implementation may have hurt the statistical power of a completed RCT and contributed to the null result. An intervention that fell prey to power problems might merit re-trial with an improved trial protocol that addresses implementation flaws and statistical power. Consider additional analysis strategies alongside ITT when a care model RCT is underpowered due to incomplete uptake or engagement. When a trial is underpowered due to variable participant responsiveness, the gold standard ITT analysis may tell an incomplete story. Evaluators and innovators should remember that ITT represents the effect of treatment assignment, which can be problematic when treatment assignment does not consistently lead to treatment receipt. In these cases, the ITT measure of the intervention effect is based on a mixture of treated and untreated patients, necessarily lowering the average effect size. There are several analysis strategies that can be considered alongside ITT in situations when low uptake or engagement leads to power loss, including the Complier Average Causal Effect10, 11 and the Distillation Method.12 These statistical methods can help elucidate whether the intervention worked for those patients who actually received it without introducing biases inherent in ‘as treated’ or ‘per protocol’ analyses. Together with evaluation experts, innovators should consider secondary analyses using one or more of these approaches. In planning trials of health care innovations, it is critical that we think more carefully about power, because underpowered evaluations have little chance of detecting programme effects even when they are present. There are real risks to repeatedly mounting underpowered trials in a learning health system. Evaluation practices from the classic pharma-trial framework may not translate seamlessly to care model trials, and for the latter may be erring in the direction of throwing out good interventions (because of false-negative results). And because null evaluations are rarely published,2 programme designers may be re-testing ideas that have already been trialed. Perhaps a more serious risk: care delivery innovators could lose patience with rigorous evaluation if every intervention fails rigorous testing. This would be a concerning outcome, because less rigorous evaluation approaches such as simple pre-post comparisons are fraught with bias and can lead to spurious conclusions that interventions are effective. Extramurally funded trials are generally subject to rigorous review processes that make them less prone to common sources of power problems, but many care model trials within learning health systems do not benefit from this type of vetting process. Thus, for a learning health system to succeed, all stakeholders including innovators, evaluators, sponsors, and patients must collaborate to design better care model trials, paying special attention to the issues of statistical power and the prevention of underpowered trials. Furthermore, when a trial's results are null, study teams should seek to understand whether power problems may have contributed to the absence of an effect. This work was supported by The Commonwealth Fund, a national, private foundation based in New York City that supports independent research on health care issues and makes grants to improve health care practice and policy. The views presented here are those of the authors and not necessarily those of The Commonwealth Fund, its directors, officers, or staff. The authors declare no conflict of interest. Data sharing is not applicable to this article as no new data were created or analysed in this study.
更多查看译文
关键词
evaluation,implementation fidelity,innovation,models of care,randomised controlled trials,statistical power
AI 理解论文
溯源树
样例
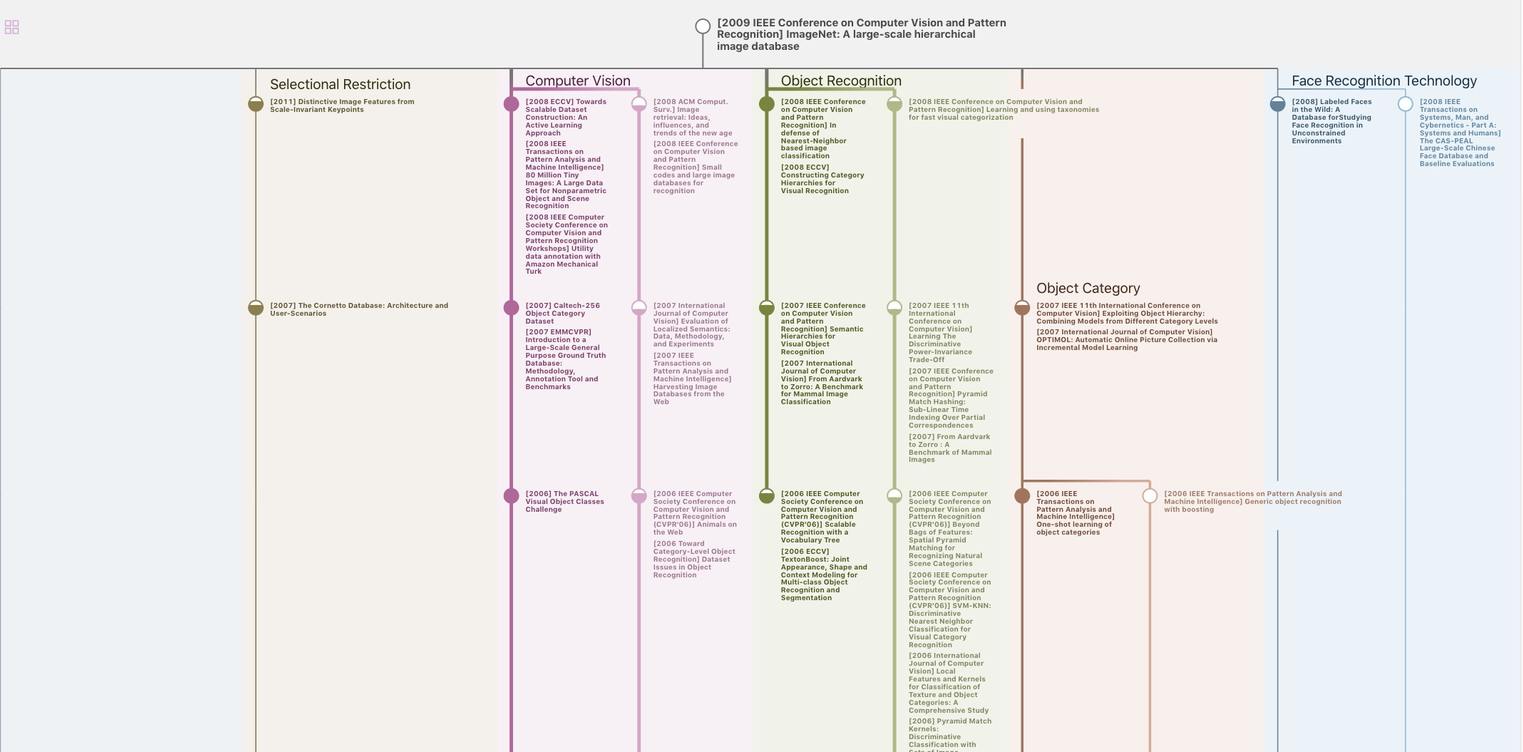
生成溯源树,研究论文发展脉络
Chat Paper
正在生成论文摘要