Data Utilization Versus Privacy Protection in Semantic Communications
IEEE Wireless Communications(2023)
摘要
Semantic communication is considered as a promising enabler for saving gradually exhausted spectrum resources due to its innovative idea of aggressively extracting critical information. Although the learnable semantic-aware codec brings exciting compression gains, its data-driven kernel endogenously incurs conflicts between data utilization and privacy protection. Namely, the data utility loss hurts transmission reliability while the need toward knowledge sharing brings privacy disclosure concerns. In this article, we first analyze the key challenges of semantic communication derived from its high dependence on sample richness and diversity. To strike an efficient trade-off between data utilization and privacy protection, we propose a joint online inference and offline training framework for semantic communication, which covers privacy-protected codec training and personalized codec deployment. Specifically, a novel distributed learning strategy is developed for codec training, where model splitting between clients and servers makes gradient leakage attacks computationally prohibitive and partial model aggregation provides customized data utilization. For personalized codec deployment, a model pre-training and distillation scheme is designed by exploiting modality-aware semantic associations. Lastly, an experimental case study demonstrates the superiority of our proposed strategy in balancing data utilization and privacy protection in semantic communications.
更多查看译文
关键词
semantic communications,privacy,data,protection,utilization
AI 理解论文
溯源树
样例
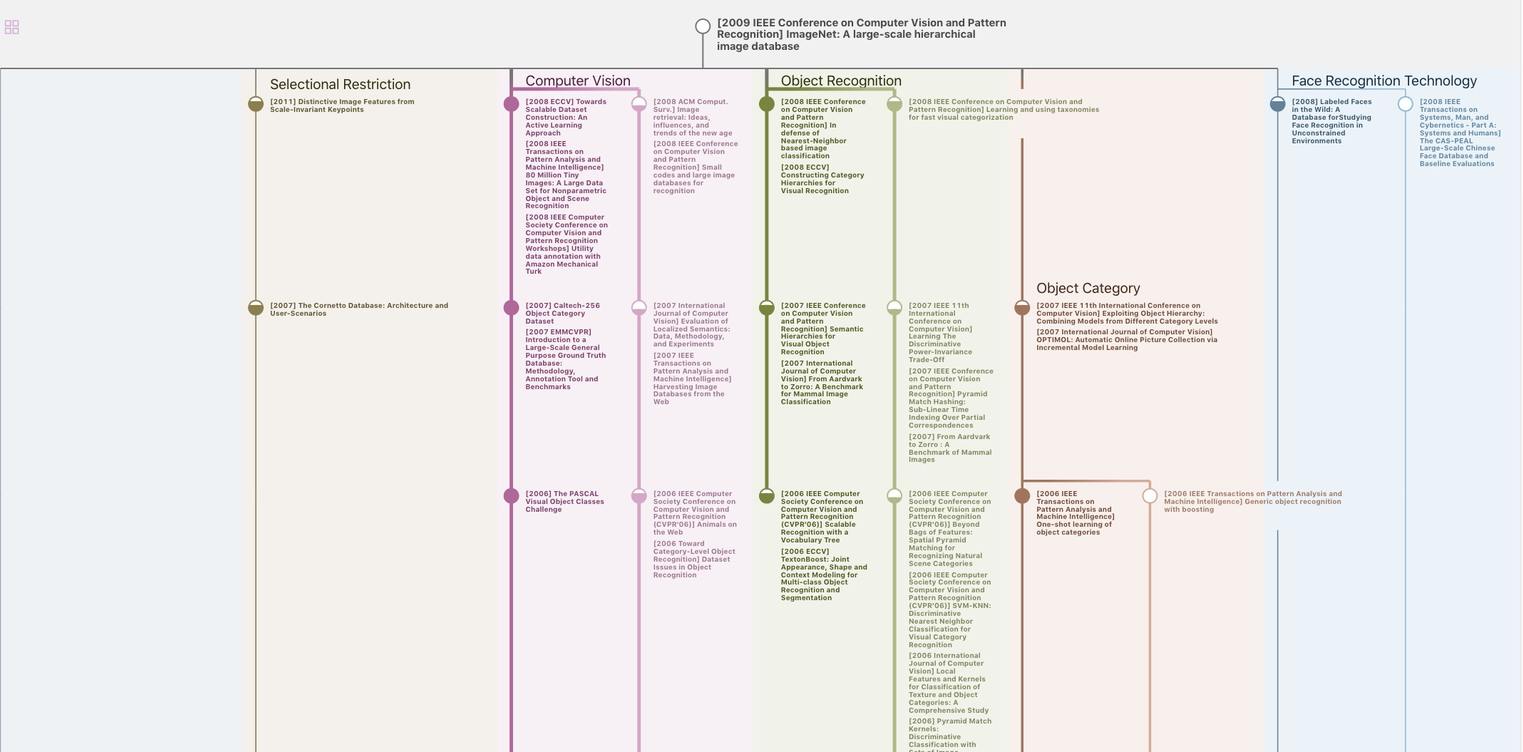
生成溯源树,研究论文发展脉络
Chat Paper
正在生成论文摘要