Atomic structures, conformers and thermodynamic properties of 32k atmospheric molecules
Scientific Data(2023)
摘要
Low-volatile organic compounds (LVOCs) drive key atmospheric processes, such as new particle formation (NPF) and growth. Machine learning tools can accelerate studies of these phenomena, but extensive and versatile LVOC datasets relevant for the atmospheric research community are lacking. We present the GeckoQ dataset with atomic structures of 31,637 atmospherically relevant molecules resulting from the oxidation of α -pinene, toluene and decane. For each molecule, we performed comprehensive conformer sampling with the COSMO conf program and calculated thermodynamic properties with density functional theory (DFT) using the Conductor-like Screening Model (COSMO). Our dataset contains the geometries of the 7 Mio. conformers we found and their corresponding structural and thermodynamic properties, including saturation vapor pressures ( p Sat ), chemical potentials and free energies. The p Sat were compared to values calculated with the group contribution method SIMPOL. To validate the dataset, we explored the relationship between structural and thermodynamic properties, and then demonstrated a first machine-learning application with Gaussian process regression.
更多查看译文
关键词
atmospheric molecules,atomic structures,thermodynamic properties
AI 理解论文
溯源树
样例
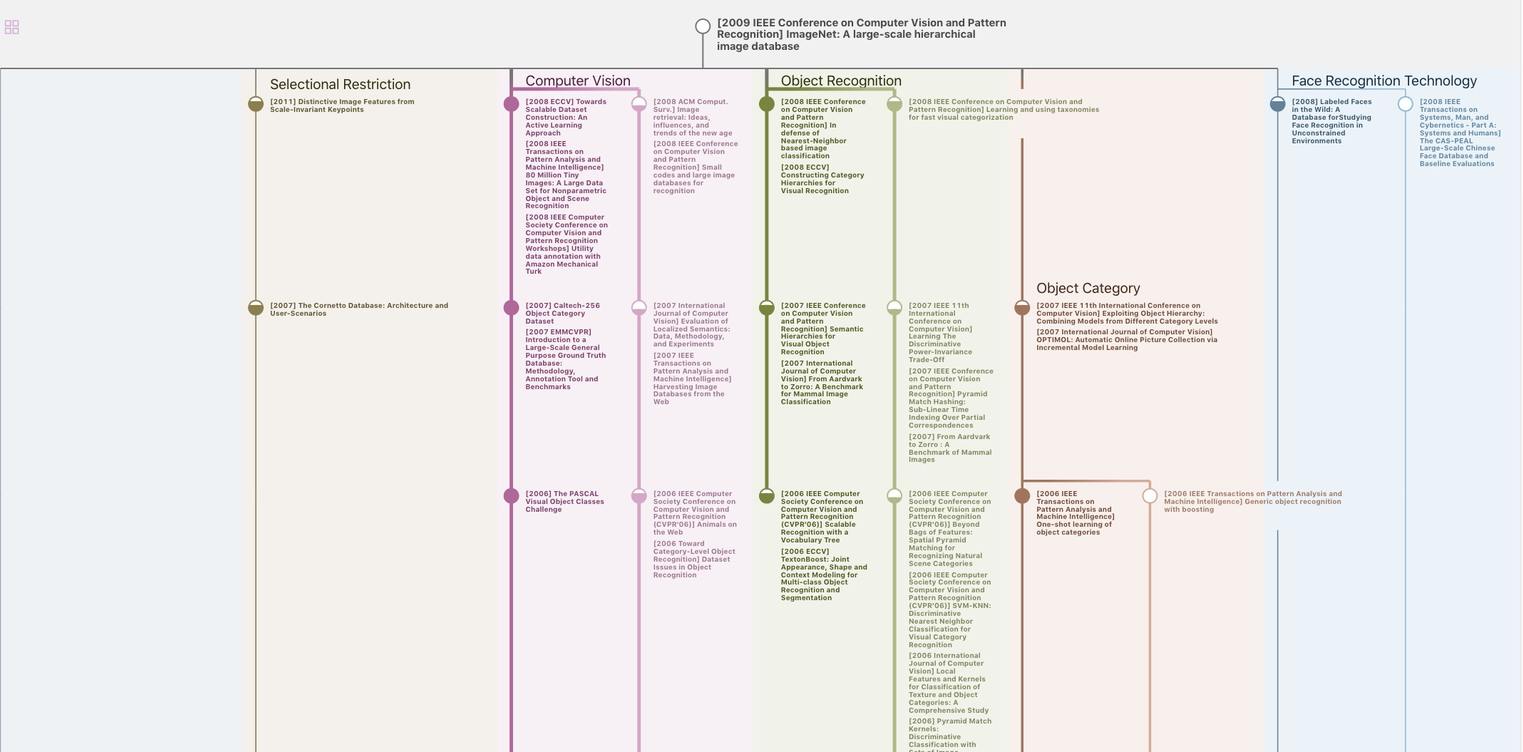
生成溯源树,研究论文发展脉络
Chat Paper
正在生成论文摘要