Iterative integration of deep learning in hybrid Earth surface system modelling
Nature Reviews Earth & Environment(2023)
摘要
Earth system modelling (ESM) is essential for understanding past, present and future Earth processes. Deep learning (DL), with the data-driven strength of neural networks, has promise for improving ESM by exploiting information from Big Data. Yet existing hybrid ESMs largely have deep neural networks incorporated only during the initial stage of model development. In this Perspective, we examine progress in hybrid ESM, focusing on the Earth surface system, and propose a framework that integrates neural networks into ESM throughout the modelling lifecycle. In this framework, DL computing systems and ESM-related knowledge repositories are set up in a homogeneous computational environment. DL can infer unknown or missing information, feeding it back into the knowledge repositories, while the ESM-related knowledge can constrain inference results of the DL. By fostering collaboration between ESM-related knowledge and DL systems, adaptive guidance plans can be generated through question-answering mechanisms and recommendation functions. As users interact iteratively, the hybrid system deepens its understanding of their preferences, resulting in increasingly customized, scalable and accurate guidance plans for modelling Earth processes. The advancement of this framework necessitates interdisciplinary collaboration, focusing on explainable DL and maintaining observational data to ensure the reliability of simulations.
更多查看译文
关键词
deep learning,iterative integration,earth,surface,modelling
AI 理解论文
溯源树
样例
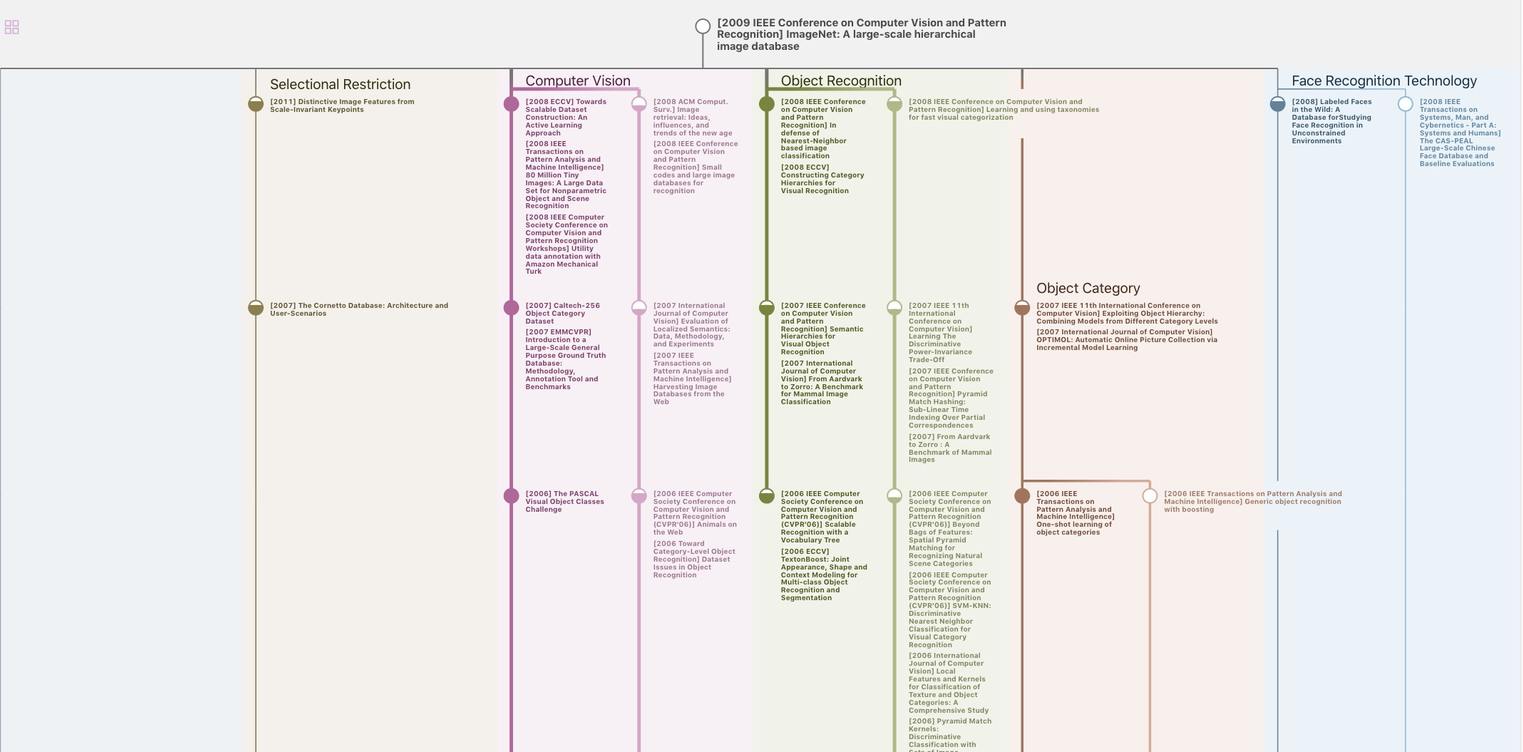
生成溯源树,研究论文发展脉络
Chat Paper
正在生成论文摘要