Going Beyond Local: Global Graph-Enhanced Personalized News Recommendations
PROCEEDINGS OF THE 17TH ACM CONFERENCE ON RECOMMENDER SYSTEMS, RECSYS 2023(2023)
摘要
Precisely recommending candidate news articles to users has always been a core challenge for personalized news recommendation systems. Most recent works primarily focus on using advanced natural language processing techniques to extract semantic information from rich textual data, employing content-based methods derived from local historical news. However, this approach lacks a global perspective, failing to account for users' hidden motivations and behaviors beyond semantic information. To address this challenge, we propose a novel model called GLORY (Global-LOcal news Recommendation sYstem), which combines global representations learned from other users with local representations to enhance personalized recommendation systems. We accomplish this by constructing a Global-aware Historical News Encoder, which includes a global news graph and employs gated graph neural networks to enrich news representations, thereby fusing historical news representations by a historical news aggregator. Similarly, we extend this approach to a Global Candidate News Encoder, utilizing a global entity graph and a candidate news aggregator to enhance candidate news representation. Evaluation results on two public news datasets demonstrate that our method outperforms existing approaches. Furthermore, our model offers more diverse recommendations.
更多查看译文
关键词
News Recommendation,Graph Neural Network,News Modeling
AI 理解论文
溯源树
样例
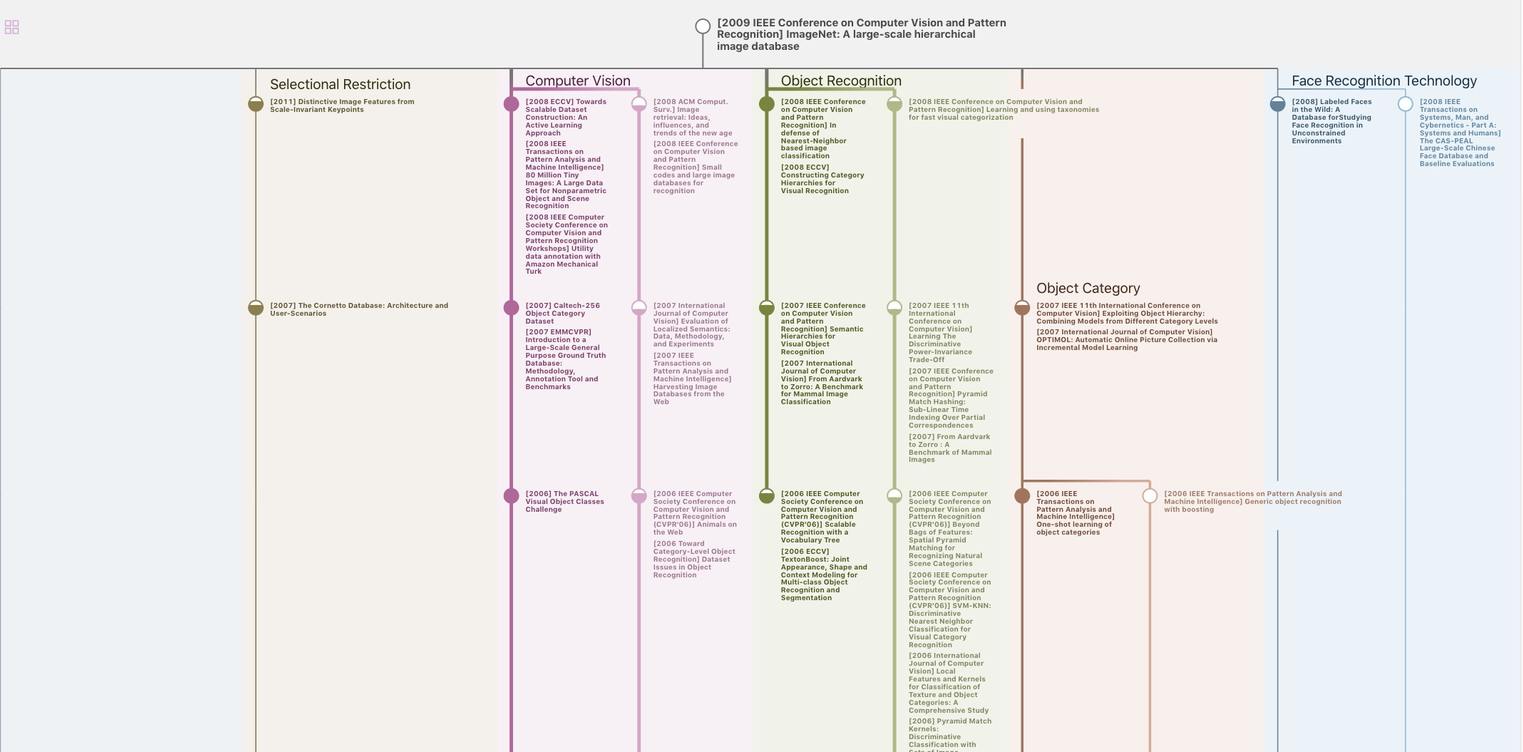
生成溯源树,研究论文发展脉络
Chat Paper
正在生成论文摘要