Efficient SGD Neural Network Training via Sublinear Activated Neuron Identification
CoRR(2023)
摘要
Deep learning has been widely used in many fields, but the model training process usually consumes massive computational resources and time. Therefore, designing an efficient neural network training method with a provable convergence guarantee is a fundamental and important research question. In this paper, we present a static half-space report data structure that consists of a fully connected two-layer neural network for shifted ReLU activation to enable activated neuron identification in sublinear time via geometric search. We also prove that our algorithm can converge in $O(M^2/\epsilon^2)$ time with network size quadratic in the coefficient norm upper bound $M$ and error term $\epsilon$.
更多查看译文
关键词
sublinear activated neuron
AI 理解论文
溯源树
样例
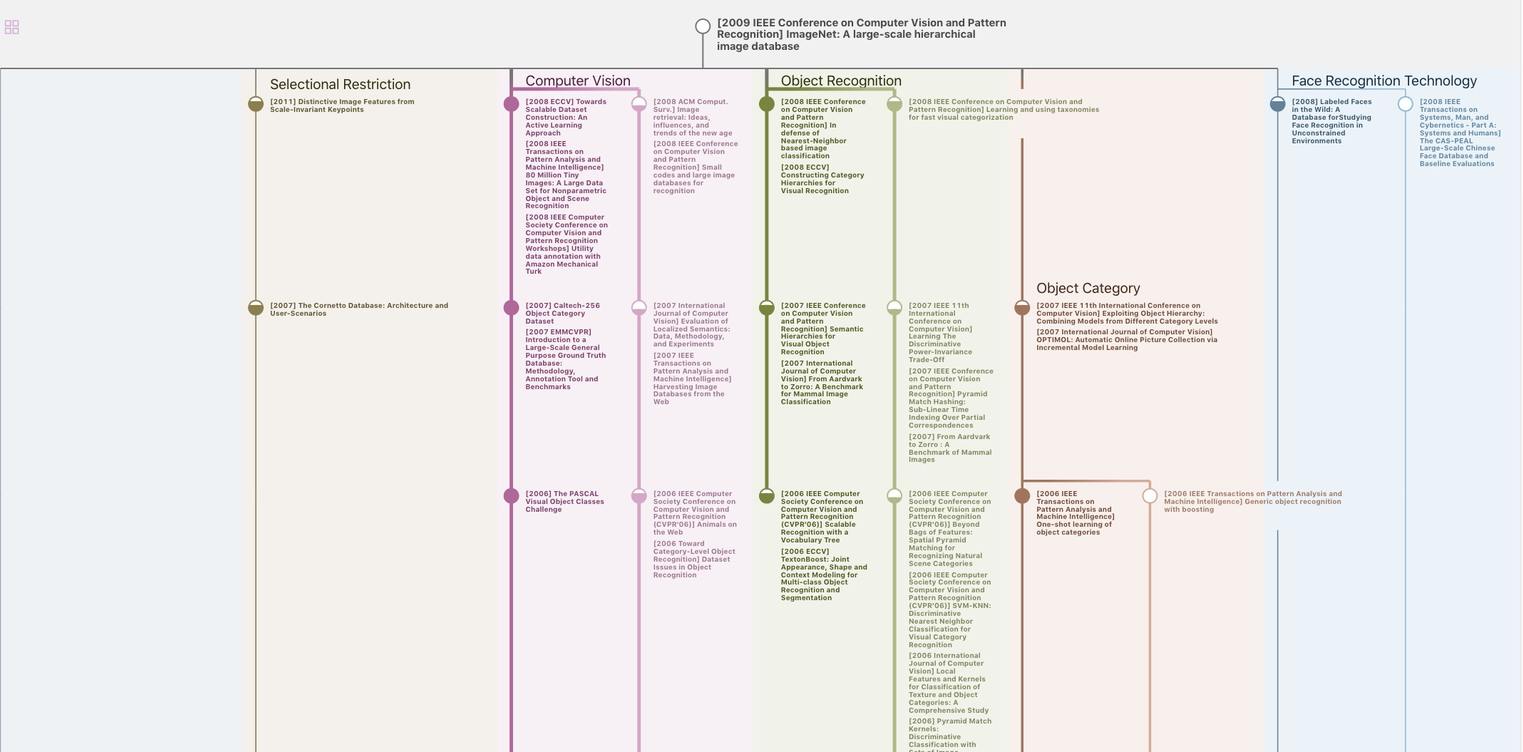
生成溯源树,研究论文发展脉络
Chat Paper
正在生成论文摘要