Deep Network Approximation: Beyond ReLU to Diverse Activation Functions
JOURNAL OF MACHINE LEARNING RESEARCH(2024)
摘要
This paper explores the expressive power of deep neural networks for a diverse range of activation functions. An activation function set A is defined to encompass the majority of commonly used activation functions, such as ReLU, LeakyReLU, ReLU2, ELU, CELU, SELU, Softplus, GELU, SiLU, Swish, Mish, Sigmoid, Tanh, Arctan, Softsign, dSiLU, and SRS. We demonstrate that for any activation function rho is an element of A , a ReLU network of width N and depth L can be approximated to arbitrary precision by a 0-activated network of width 3N and depth 2L on any bounded set. This finding enables the extension of most approximation results achieved with ReLU networks to a wide variety of other activation functions, albeit with slightly increased constants. Significantly, we establish that the (width, depth) scaling factors can be further reduced from (3, 2) to (1, 1) if rho falls within a specific subset of A . This subset includes activation functions such as ELU, CELU, SELU, Softplus, GELU, SiLU, Swish, and Mish.
更多查看译文
关键词
deep neural networks,rectified linear unit,diverse activation functions,expressive power,nonlinear approximation
AI 理解论文
溯源树
样例
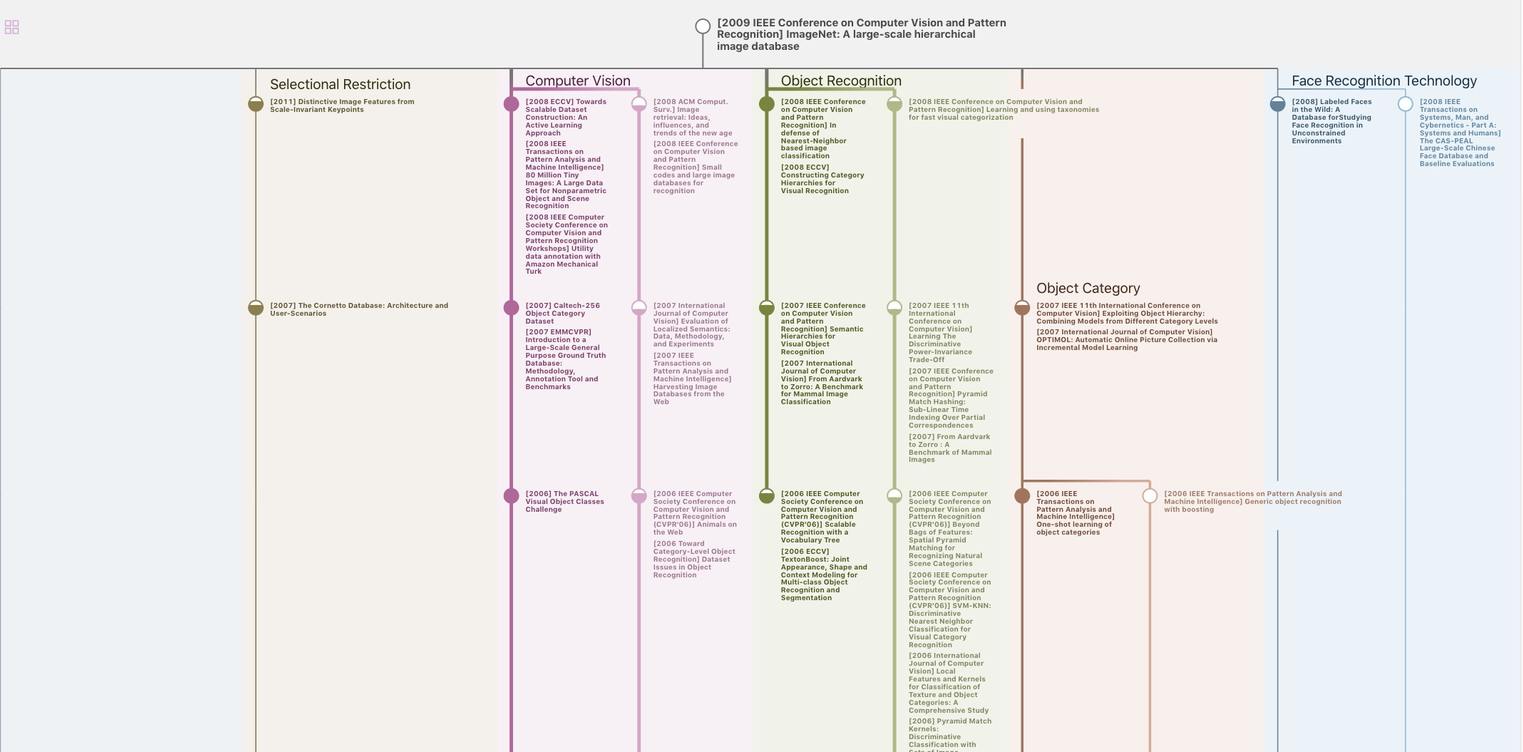
生成溯源树,研究论文发展脉络
Chat Paper
正在生成论文摘要