DSV: An Alignment Validation Loss for Self-supervised Outlier Model Selection
MACHINE LEARNING AND KNOWLEDGE DISCOVERY IN DATABASES: RESEARCH TRACK, ECML PKDD 2023, PT I(2023)
摘要
Self-supervised learning (SSL) has proven effective in solving various problems by generating internal supervisory signals. Unsupervised anomaly detection, which faces the high cost of obtaining true labels, is an area that can greatly benefit from SSL. However, recent literature suggests that tuning the hyperparameters (HP) of data augmentation functions is crucial to the success of SSL-based anomaly detection (SSAD), yet a systematic method for doing so remains unknown. In this work, we propose DSV (Discordance and Separability Validation), an unsupervised validation loss to select high-performing detection models with effective augmentation HPs. DSV captures the alignment between an augmentation function and the anomaly-generating mechanism with surrogate losses, which approximate the discordance and separability of test data, respectively. As a result, the evaluation via DSV leads to selecting an effective SSAD model exhibiting better alignment, which results in high detection accuracy. We theoretically derive the degree of approximation conducted by the surrogate losses and empirically show that DSV outperforms a wide range of baselines on 21 real-world tasks.
更多查看译文
关键词
Anomaly detection,Self-supervised learning,Unsupervised model selection,Data augmentation
AI 理解论文
溯源树
样例
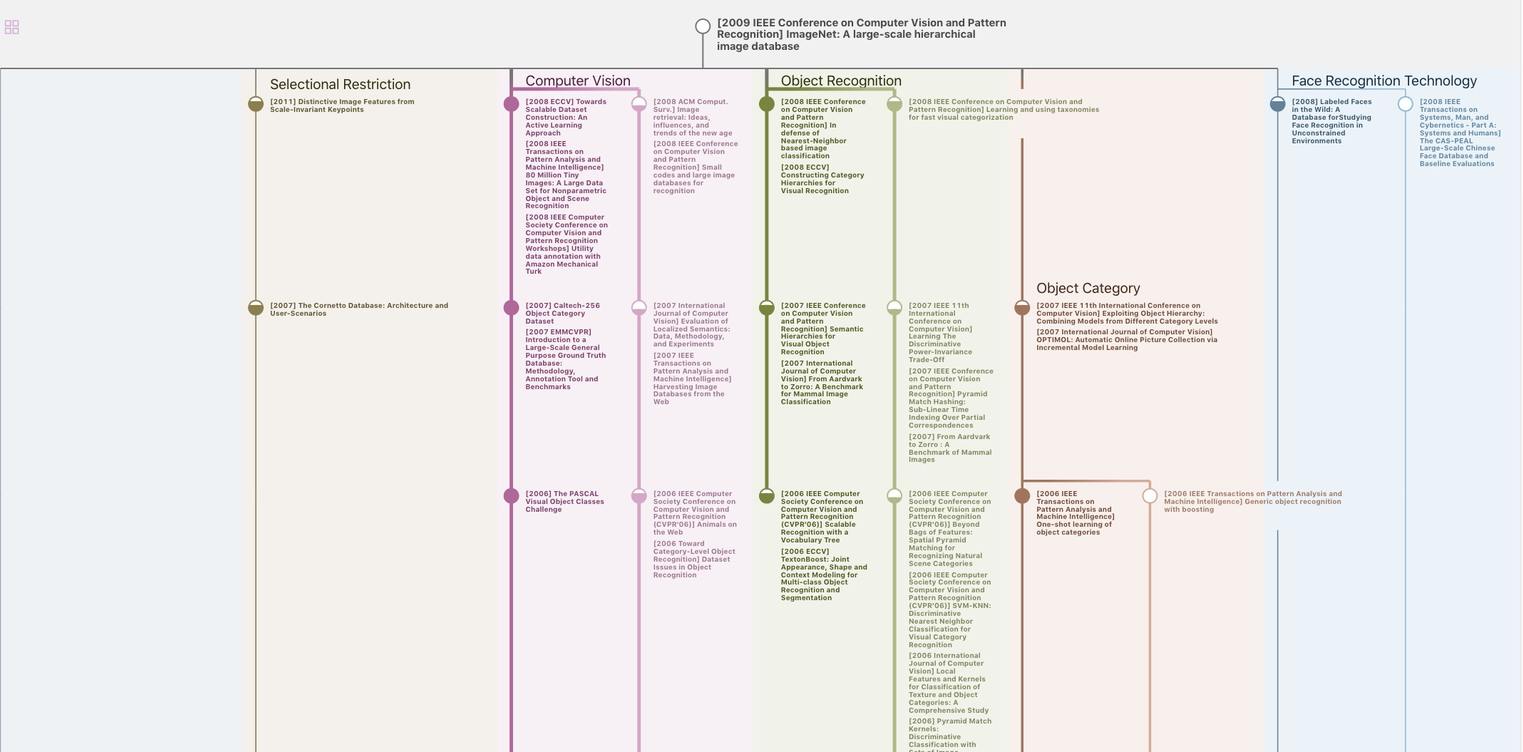
生成溯源树,研究论文发展脉络
Chat Paper
正在生成论文摘要