Deterministic Multi-sensor Measurement-adaptive Birth using Labeled Random Finite Sets
CoRR(2023)
摘要
Measurement-adaptive track initiation remains a critical design requirement of many practical multi-target tracking systems. For labeled random finite sets multi-object filters, prior work has been established to construct a labeled multi-object birth density using measurements from multiple sensors. A truncation procedure has also been provided that leverages a stochastic Gibbs sampler to truncate the birth density for scalability. In this work, we introduce a deterministic herded Gibbs sampling truncation solution for efficient multi-sensor adaptive track initialization. Removing the stochastic behavior of the track initialization procedure without impacting average tracking performance enables a more robust tracking solution more suitable for safety-critical applications. Simulation results for linear sensing scenarios are provided to verify performance.
更多查看译文
关键词
birth,random,multi-sensor,measurement-adaptive
AI 理解论文
溯源树
样例
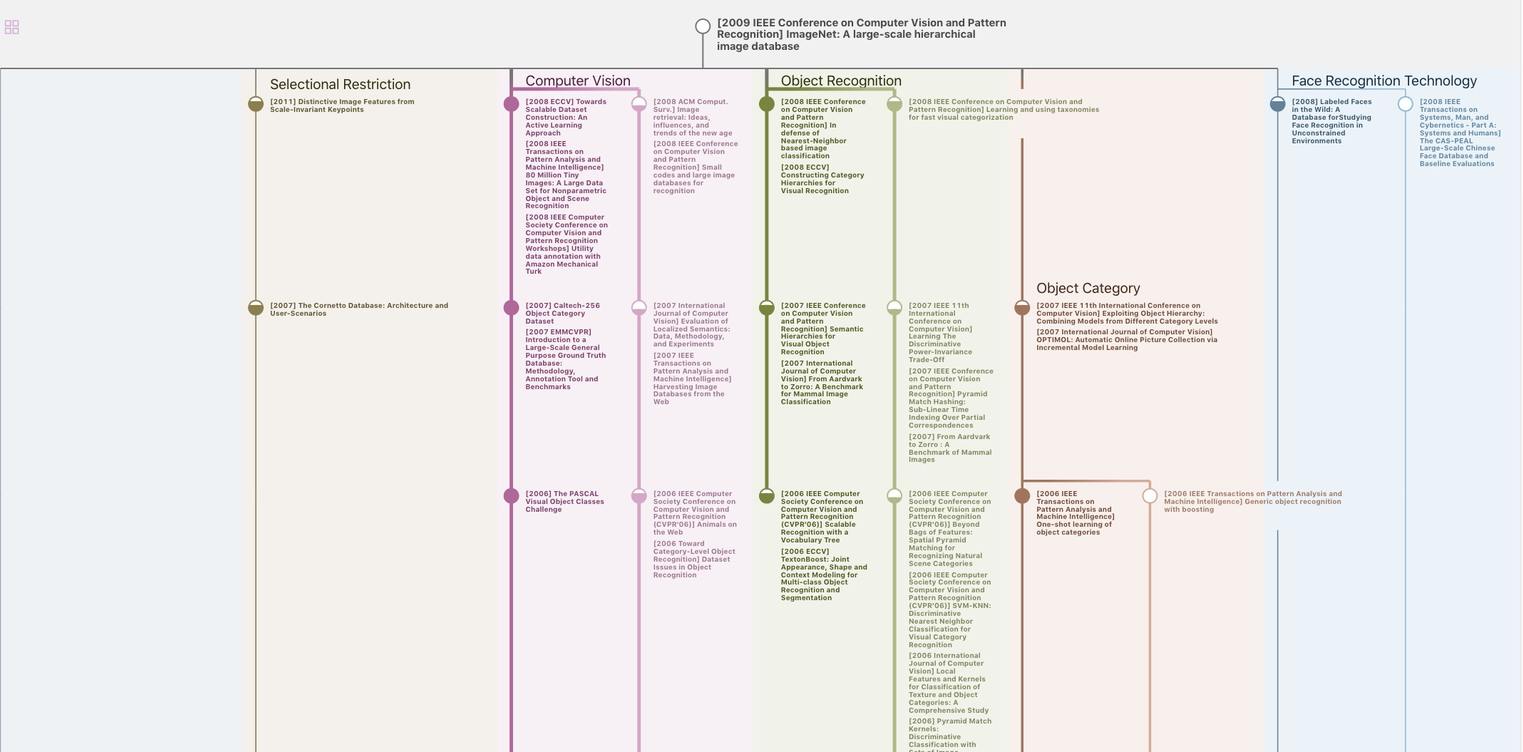
生成溯源树,研究论文发展脉络
Chat Paper
正在生成论文摘要