Computational textural mapping harmonises sampling variation and reveals multidimensional histopathological fingerprints
British Journal of Cancer(2023)
摘要
Background Technical factors can bias H&E digital slides potentially compromising computational histopathology studies. Here, we hypothesised that sample quality and sampling variation can introduce even greater and undocumented technical fallacy. Methods Using The Cancer Genome Atlas (TCGA) clear-cell renal cell carcinoma (ccRCC) as a model disease, we annotated ~78,000 image tiles and trained deep learning models to detect histological textures and lymphocyte infiltration at the tumour core and its surrounding margin and correlated these with clinical, immunological, genomic, and transcriptomic profiles. Results The models reached 95% validation accuracy for classifying textures and 95% for lymphocyte infiltration enabling reliable profiling of ccRCC samples. We validated the lymphocyte-per-texture distributions in the Helsinki dataset ( n = 64). Texture analysis indicated constitutive sampling bias by TCGA clinical centres and technically suboptimal samples. We demonstrate how computational texture mapping (CTM) can abrogate these issues by normalising textural variance. CTM-harmonised histopathological architecture resonated with both expected associations and novel molecular fingerprints. For instance, tumour fibrosis associated with histological grade, epithelial-to-mesenchymal transition, low mutation burden and metastasis. Conclusions This study highlights texture-based standardisation to resolve technical bias in computational histopathology and understand the molecular basis of tissue architecture. All code, data and models are released as a community resource.
更多查看译文
关键词
computational textural mapping harmonises,sampling,variation
AI 理解论文
溯源树
样例
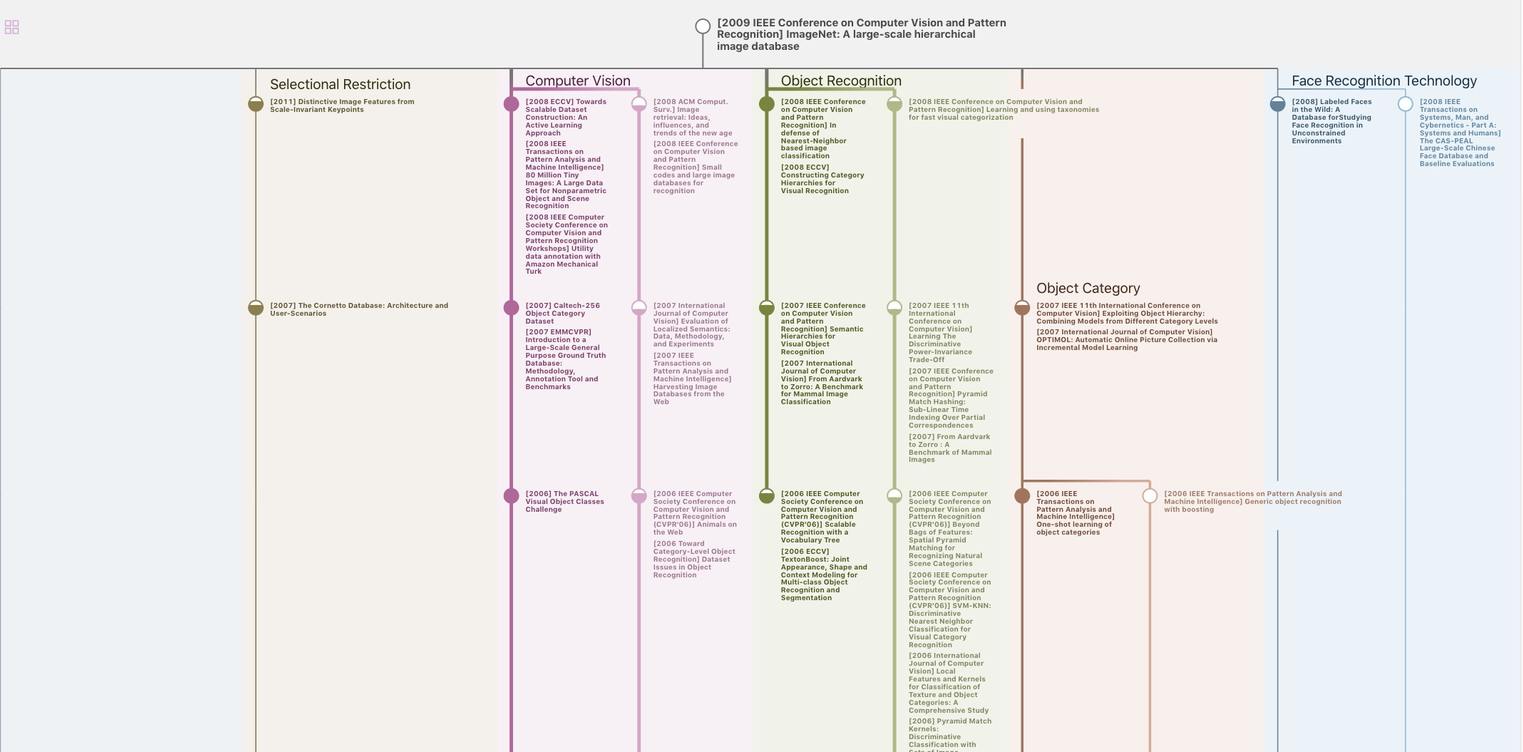
生成溯源树,研究论文发展脉络
Chat Paper
正在生成论文摘要