Comparing mechanism-based and machine learning models for predicting the effects of glucose accessibility on tumor cell proliferation
Scientific Reports(2023)
摘要
Glucose plays a central role in tumor metabolism and development and is a target for novel therapeutics. To characterize the response of cancer cells to blockade of glucose uptake, we collected time-resolved microscopy data to track the growth of MDA-MB-231 breast cancer cells. We then developed a mechanism-based, mathematical model to predict how a glucose transporter (GLUT1) inhibitor (Cytochalasin B) influences the growth of the MDA-MB-231 cells by limiting access to glucose. The model includes a parameter describing dose dependent inhibition to quantify both the total glucose level in the system and the glucose level accessible to the tumor cells. Four common machine learning models were also used to predict tumor cell growth. Both the mechanism-based and machine learning models were trained and validated, and the prediction error was evaluated by the coefficient of determination ( R 2 ). The random forest model provided the highest accuracy predicting cell dynamics ( R 2 = 0.92), followed by the decision tree ( R 2 = 0.89), k -nearest-neighbor regression ( R 2 = 0.84), mechanism-based ( R 2 = 0.77), and linear regression model ( R 2 = 0.69). Thus, the mechanism-based model has a predictive capability comparable to machine learning models with the added benefit of elucidating biological mechanisms.
更多查看译文
关键词
glucose accessibility,machine learning models,machine learning,tumor cell proliferation,mechanism-based
AI 理解论文
溯源树
样例
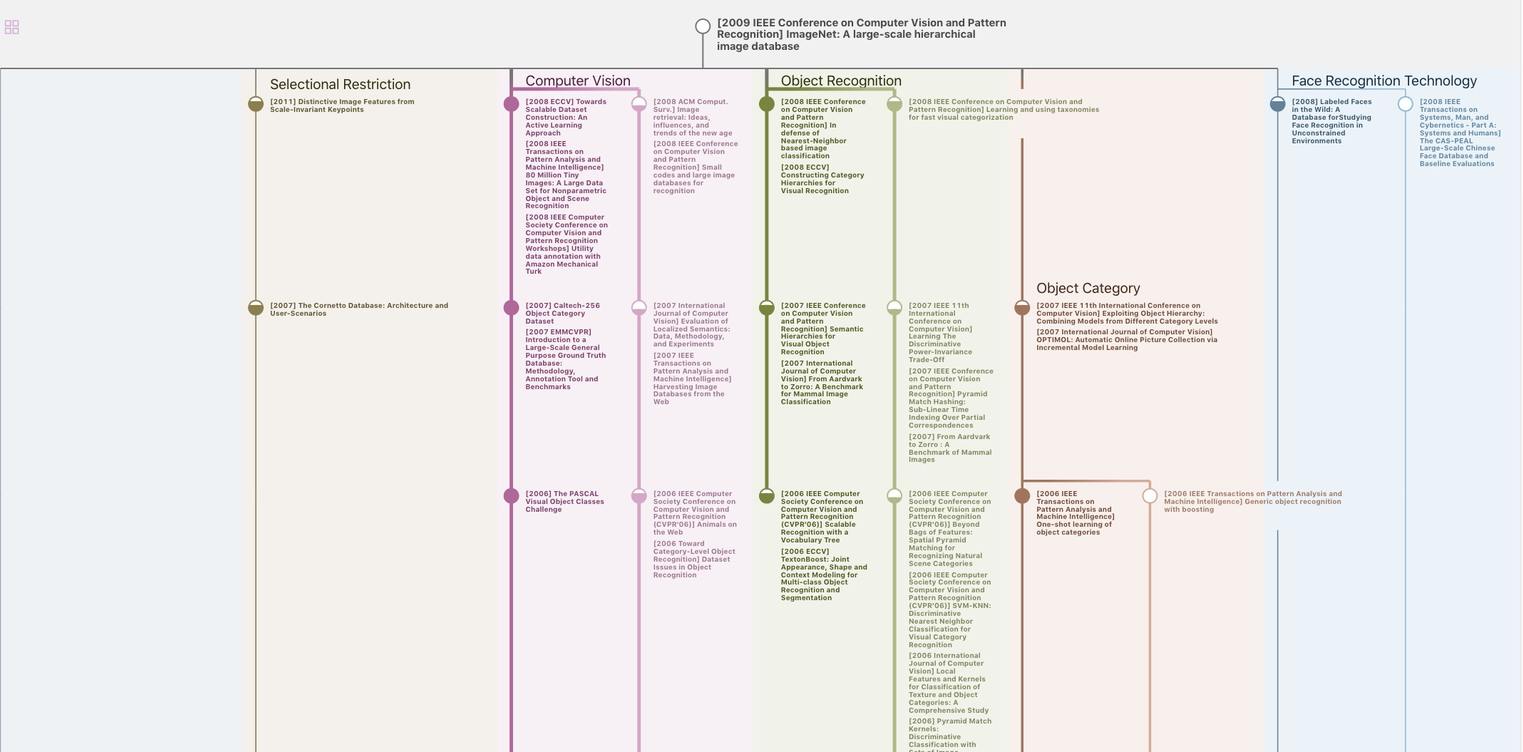
生成溯源树,研究论文发展脉络
Chat Paper
正在生成论文摘要