MSTGAN: A Multi-Slot Conditional Generative Adversarial Network Based on Swin Transformer for Channel Estimation
IEEE Communications Letters(2023)
摘要
Accurate channel estimation is the key to enhance the transmission performance of communication systems. In this letter, we propose a multi-slot conditional generative adversarial network (cGAN) based on Swin Transformer called MSTGAN for channel estimation in a single-input single-output (SISO) scenario. Specifically, the proposed MSTGAN could learn the temporal correlation feature from continuous channel slots by the 3D convolution and extract the deep feature well by the Swin Transformer to improve the accuracy of channel estimation. The model is trained with data augmentation to reduce the computational cost for offline training. The simulation results demonstrate that the proposed method outperforms the linear minimum mean square error (LMMSE) method and other deep learning methods. Furthermore, extension schemes of the MSTGAN to the multiple-input multiple-output (MIMO) case are also provided.
更多查看译文
关键词
Channel estimation,deep learning,conditional generative adversarial network,swin transformer,multi-slot
AI 理解论文
溯源树
样例
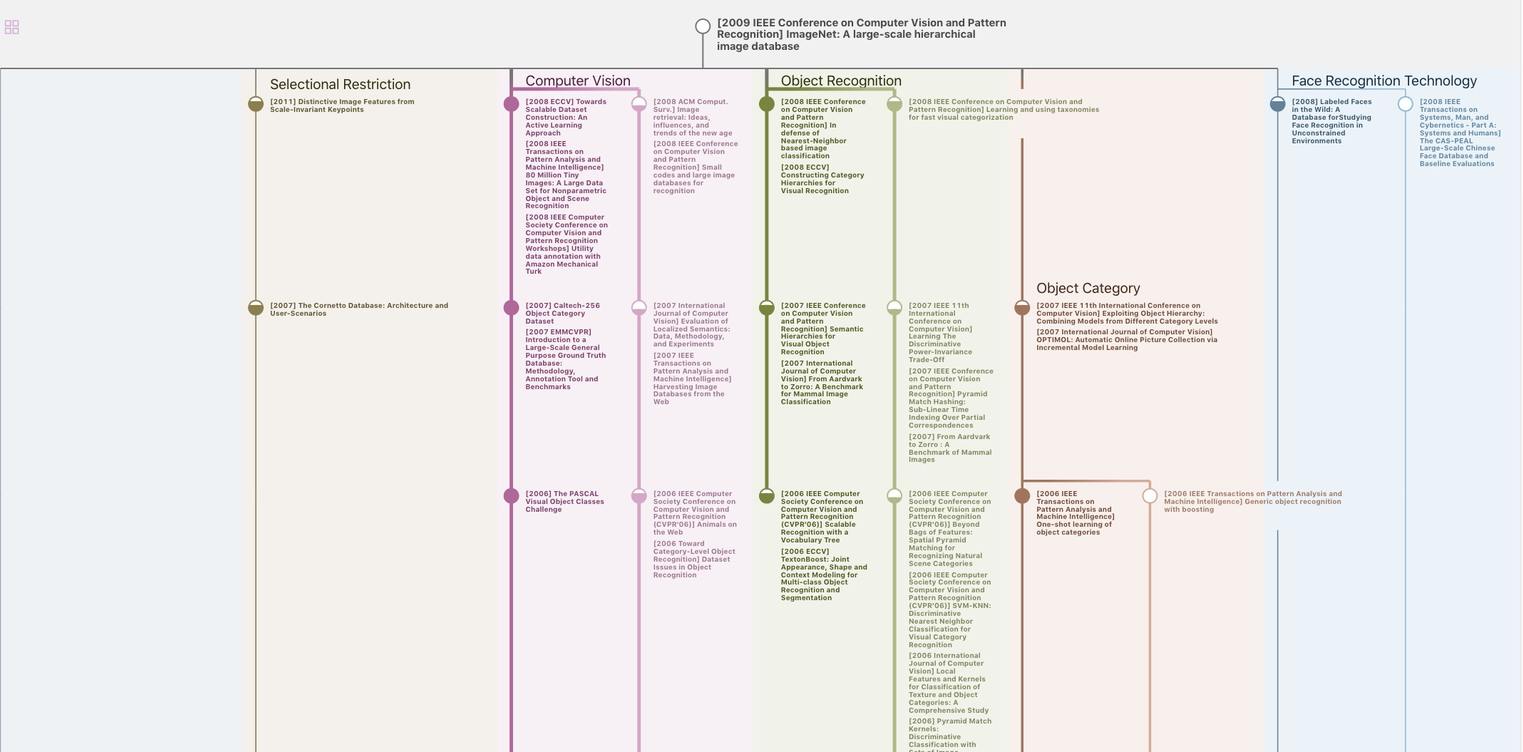
生成溯源树,研究论文发展脉络
Chat Paper
正在生成论文摘要