Urban Region Representation Learning with OpenStreetMap Building Footprints.
KDD(2023)
摘要
The prosperity of crowdsourcing geospatial data provides increasing opportunities to understand our cities. In particular, Open-StreetMap (OSM) has become a prominent vault of geospatial data on the Web. In this context, learning urban region representations from OSM data, which is unexplored in previous work, could be profitable for various downstream tasks. In this work, we utilize OSM buildings (footprints) complemented with points of interest (POIs) to learn region representations, as buildings' shapes, spatial distributions, and properties have tight linkages to different urban functions. However, appealing as it seems, urban buildings often exhibit complex patterns to form dense or sparse areas, which brings significant challenges for unsupervised feature extraction. To address the challenges, we propose RegionDCL1, an unsupervised framework to deeply mine urban buildings. In a nutshell, we leverage random points generated by Poisson Disk Sampling to tackle data-sparse areas and utilize triplet loss with a novel adaptive margin to preserve inter-region correlations. Furthermore, we train our model with group-level and region-level contrastive learning, making it adaptive to varying region partitions. Extensive experiments in two global cities demonstrate that RegionDCL consistently outperforms the state-of-the-art counterparts across different region partitions, and outputs effective representations for inferring urban land use and population density.
更多查看译文
关键词
Geospatial data mining,OpenStreetMap,urban regions,representation learning
AI 理解论文
溯源树
样例
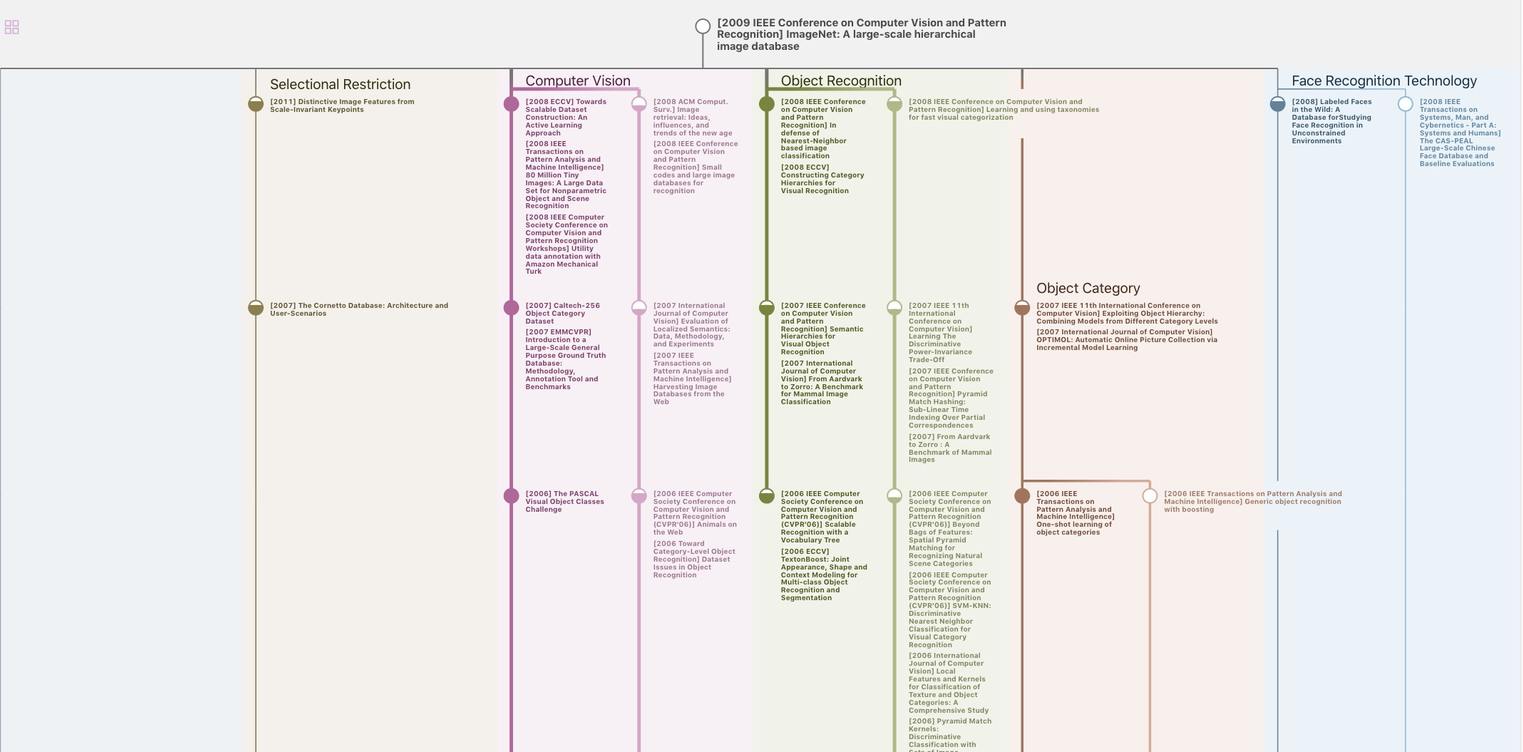
生成溯源树,研究论文发展脉络
Chat Paper
正在生成论文摘要