Navigating Alignment for Non-identical Client Class Sets: A Label Name-Anchored Federated Learning Framework.
KDD(2023)
摘要
Traditional federated classification methods, even those designed for non-IID clients, assume that each client annotates its local data with respect to the same universal class set. In this paper, we focus on a more general yet practical setting, non-identical client class sets, where clients focus on their own (different or even non-overlapping) class sets and seek a global model that works for the union of these classes. If one views classification as finding the best match between representations produced by data/label encoder, such heterogeneity in client class sets poses a new significant challenge-local encoders at different clients may operate in different and even independent latent spaces, making it hard to aggregate at the server. We propose a novel framework, FEDALIGN(1), to align the latent spaces across clients from both label and data perspectives. From a label perspective, we leverage the expressive natural language class names as a common ground for label encoders to anchor class representations and guide the data encoder learning across clients. From a data perspective, during local training, we regard the global class representations as anchors and leverage the data points that are close/far enough to the anchors of locally-unaware classes to align the data encoders across clients. Our theoretical analysis of the generalization performance and extensive experiments on four real-world datasets of different tasks confirm that FEDALIGN outperforms various state-of-the-art (non-IID) federated classification methods.
更多查看译文
关键词
federated learning,non-IID,label semantics modeling
AI 理解论文
溯源树
样例
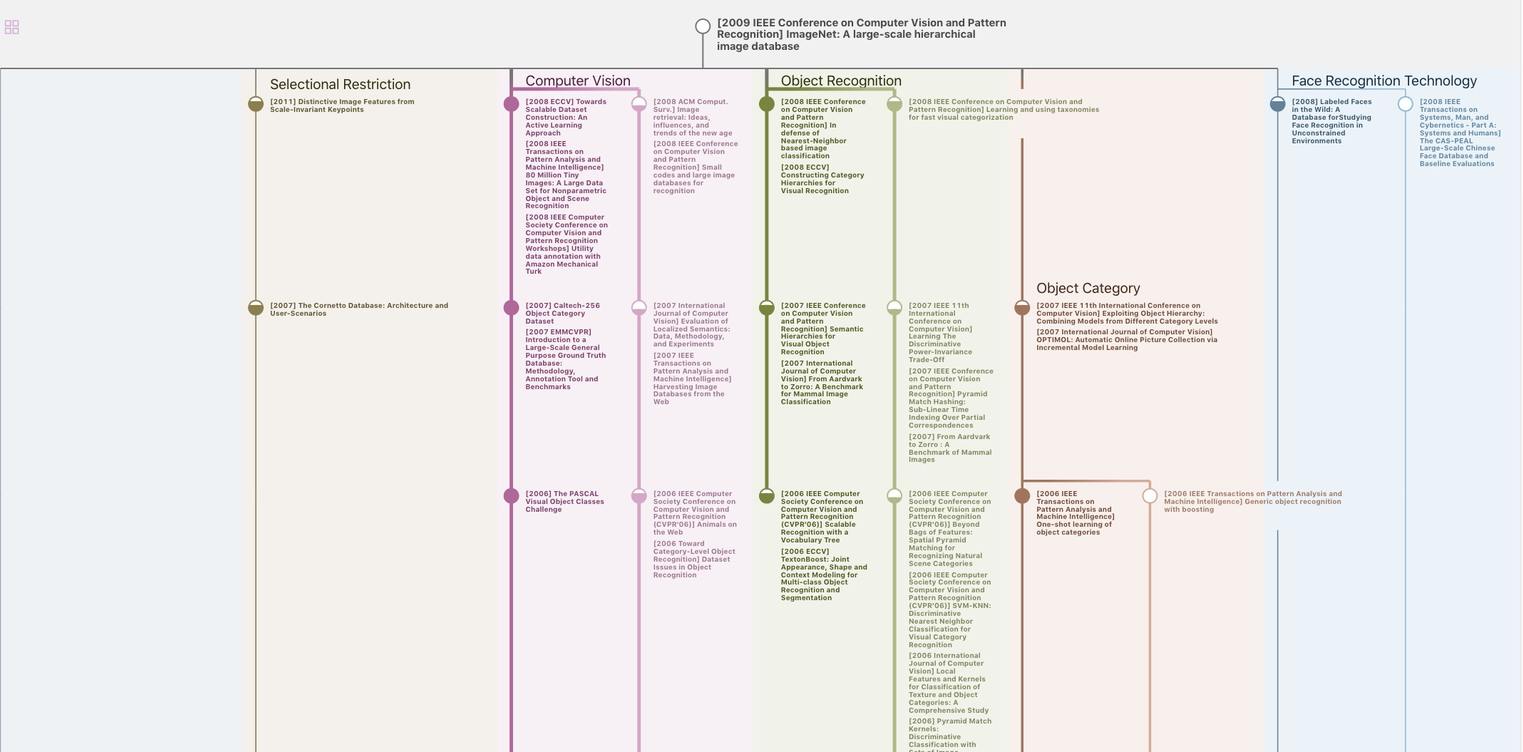
生成溯源树,研究论文发展脉络
Chat Paper
正在生成论文摘要