Time-to-Event Modeling with Hypernetwork based Hawkes Process
KDD 2023(2023)
摘要
Many real-world applications are associated with collection of events with timestamps, known as time-to-event data. Earthquake occurrences, social networks, and user activity logs can be represented as a sequence of discrete events observed in continuous time. Temporal point process serves as an essential tool for modeling such time-to-event data in continuous time space. Despite having massive amounts of event sequence data from various domains like social media, healthcare etc., real world application of temporal point process faces two major challenges: 1) it is not generalizable to predict events from unseen event sequences in dynamic environment 2) they are not capable of thriving in continually evolving environment with minimal supervision while retaining previously learnt knowledge. To tackle these issues, we propose HyperHawkes, a hypernetwork based temporal point process framework which is capable of modeling time of event occurrence for unseen sequences and consequently, zero-shot learning for time-to-event modeling. We also develop a hypernetwork based continually learning temporal point process for continuous modeling of time-to-event sequences with minimal forgetting. HyperHawkes augments the temporal point process with zero-shot modeling and continual learning capabilities. We demonstrate the application of the proposed framework through our experiments on real-world datasets. Our results show the efficacy of the proposed approach in terms of predicting future events under zero-shot regime for unseen event sequences. We also show that the proposed model is able to learn the time-to-event sequences continually while retaining information from previous event sequences, mitigating catastrophic forgetting in neural temporal point process.
更多查看译文
关键词
Time-to-Event Modeling,Point Process,Neural Hawkes Process
AI 理解论文
溯源树
样例
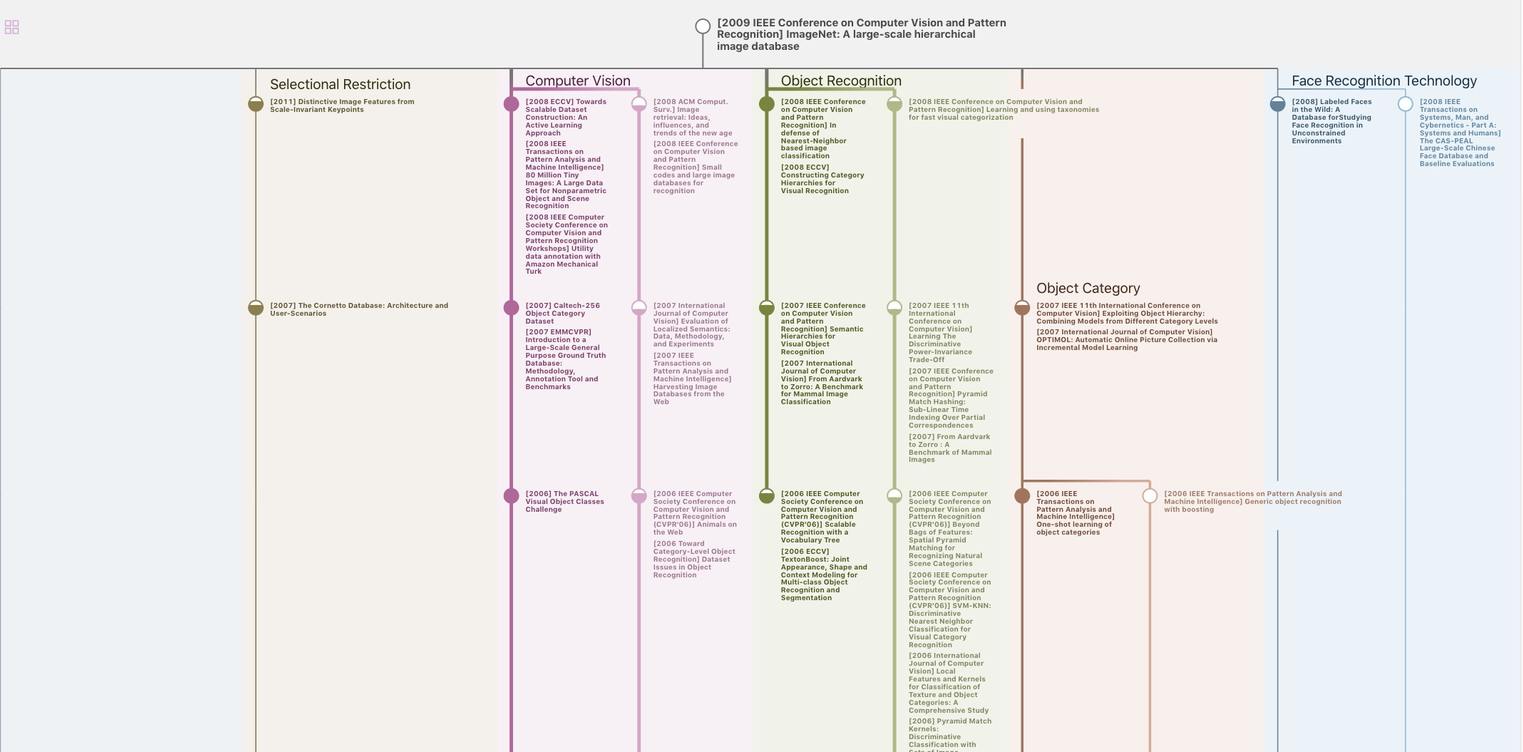
生成溯源树,研究论文发展脉络
Chat Paper
正在生成论文摘要