FLAMES2Graph: An Interpretable Federated Multivariate Time Series Classification Framework.
KDD(2023)
摘要
Increasing privacy concerns have led to decentralized and federated machine learning techniques that allow individual clients to consult and train models collaboratively without sharing private information. Some of these applications, such as medical and healthcare, require the final decisions to be interpretable. One common form of data in these applications is multivariate time series, where deep neural networks, especially convolutional neural networks based approaches, have established excellent performance in their classification tasks. However, promising results and performance of deep learning models are a black box, and their decisions cannot always be guaranteed and trusted. While several approaches address the interpretability of deep learning models for multivariate time series data in a centralized environment, less effort has been made in a federated setting. In this work, we introduce FLAMES2Graph, a new horizontal federated learning framework designed to interpret the deep learning decisions of each client. FLAMES2Graph extracts and visualizes those input subsequences that are highly activated by a convolutional neural network. Besides, an evolution graph is created to capture the temporal dependencies between the extracted distinct subsequences. The federated learning clients only share this temporal evolution graph with the centralized server instead of trained model weights to create a global evolution graph. Our extensive experiments on various datasets from well-known multivariate benchmarks indicate that the FLAMES2Graph framework significantly outperforms other state-of-the-art federated methods while keeping privacy and augmenting network decision interpretation.
更多查看译文
关键词
Federated learning,Interpretability,Neural networks,Time series
AI 理解论文
溯源树
样例
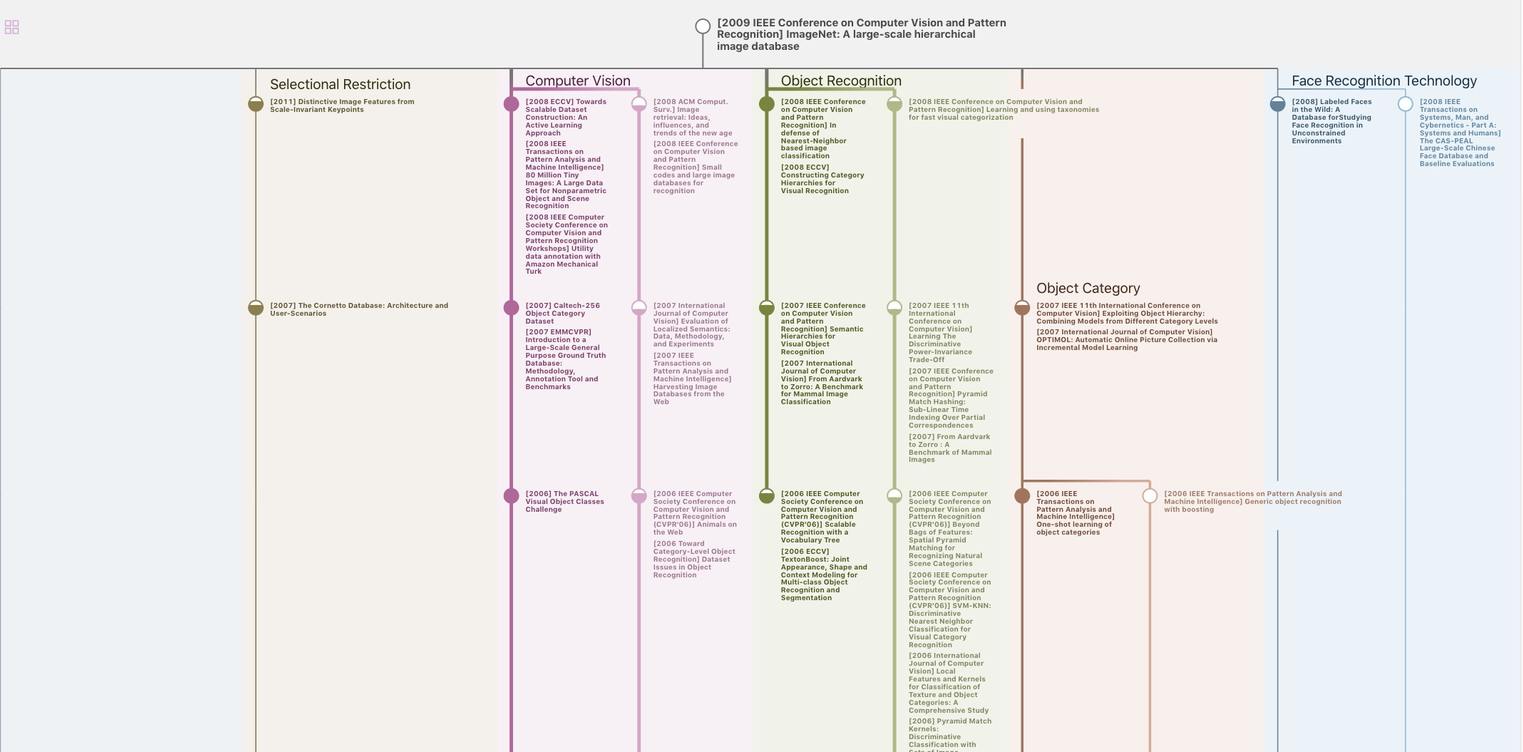
生成溯源树,研究论文发展脉络
Chat Paper
正在生成论文摘要