HUGE: Huge Unsupervised Graph Embeddings with TPUs
KDD 2023(2023)
摘要
Graphs are a representation of structured data that captures the relationships between sets of objects. With the ubiquity of available network data, there is increasing industrial and academic need to quickly analyze graphs with billions of nodes and trillions of edges. A common first step for network understanding is Graph Embedding, the process of creating a continuous representation of nodes in a graph. A continuous representation is often more amenable, especially at scale, for solving downstream machine learning tasks such as classification, link prediction, and clustering. A high-performance graph embedding architecture leveraging Tensor Processing Units (TPUs) with configurable amounts of high-bandwidth memory is presented that simplifies the graph embedding problem and can scale to graphs with billions of nodes and trillions of edges. We verify the embedding space quality on real and synthetic large-scale datasets.
更多查看译文
关键词
graph embedding,scalable algorithms,tensor processing units
AI 理解论文
溯源树
样例
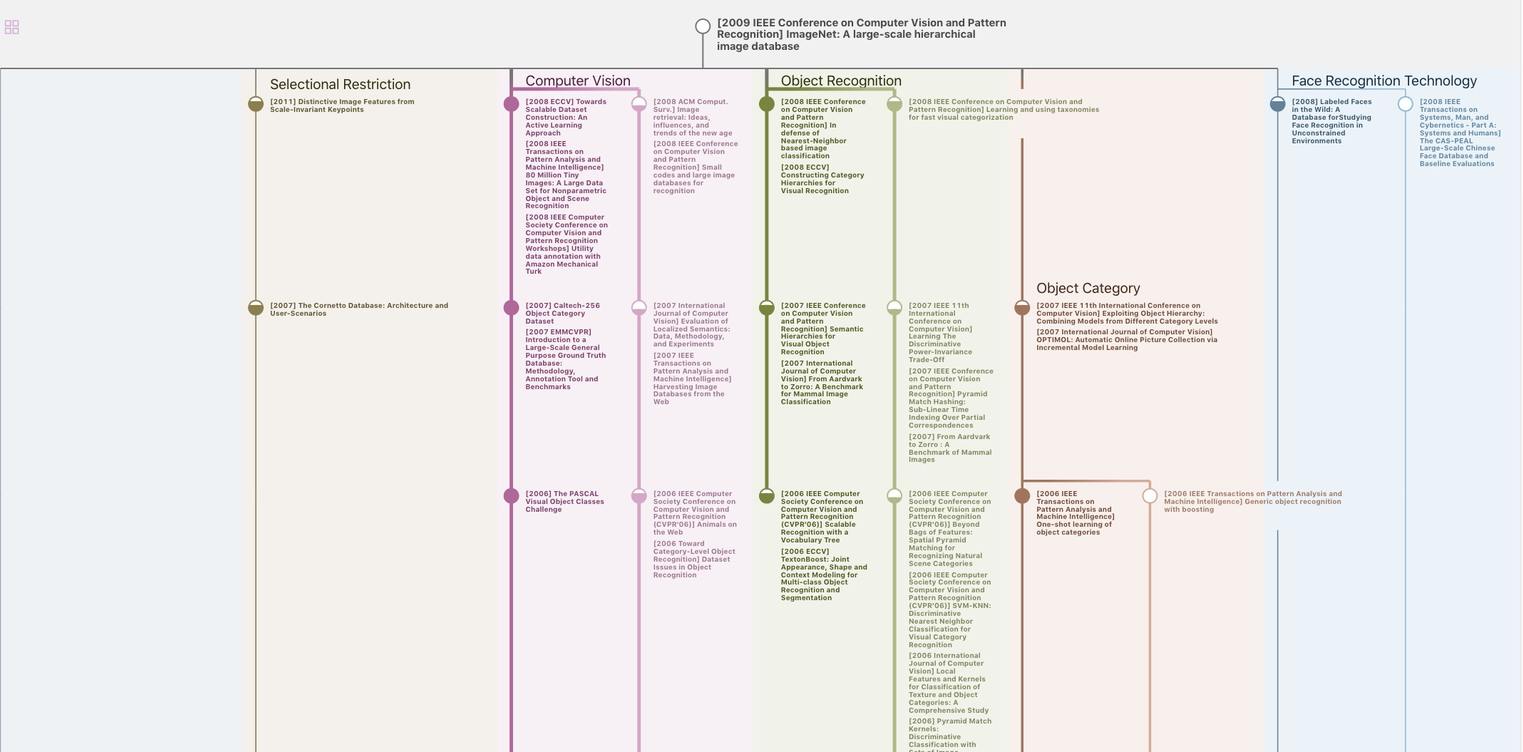
生成溯源树,研究论文发展脉络
Chat Paper
正在生成论文摘要