Dependence and Model Selection in LLP: The Problem of Variants.
KDD(2023)
摘要
The problem of Learning from Label Proportions (LLP) has received considerable research attention and has numerous practical applications. In LLP, a hypothesis assigning labels to items is learned using knowledge of only the proportion of labels found in predefined groups, called bags. While a number of algorithmic approaches to learning in this context have been proposed, very little work has addressed the model selection problem for LLP. Nonetheless, it is not obvious how to extend straightforward model selection approaches to LLP, in part because of the lack of item labels. More fundamentally, we argue that a careful approach to model selection for LLP requires consideration of the dependence structure that exists between bags, items, and labels. In this paper we formalize this structure and show how it affects model selection. We show how this leads to improved methods of model selection that we demonstrate outperform the state of the art over a wide range of datasets and LLP algorithms.
更多查看译文
关键词
Learning from Label Proportions,Hyperparameter Selection,Weakly Supervised Learning
AI 理解论文
溯源树
样例
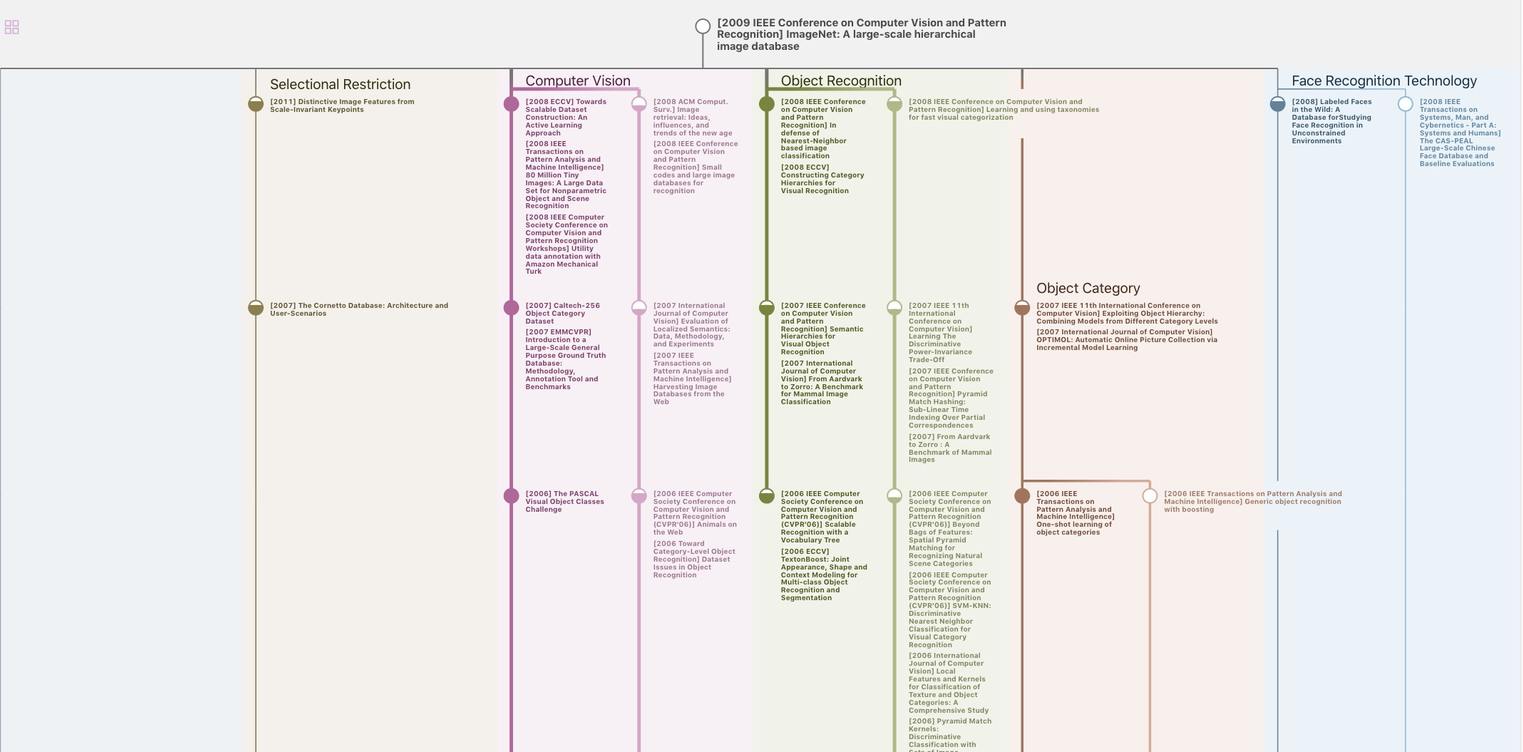
生成溯源树,研究论文发展脉络
Chat Paper
正在生成论文摘要