Community-based Dynamic Graph Learning for Popularity Prediction.
KDD(2023)
摘要
Popularity prediction, which aims to forecast how many users would like to interact with a target item or online content in the future, can help online shopping or social media platforms to identify popular items or digital contents. Many efforts have been made to study how the multi-faceted factors, such as item features, user preferences, and social influence, affect user-item interactions, but little work has focused on the evolutionary dynamics of these factors for individuals or groups. In that light, this paper develops a community-based dynamic graph learning method for popularity prediction. First, a dynamic graph learning framework is proposed to maintain a dynamic representation for each item or user entity and update the representations according to the newly observed user-item interactions. Second, a community detection module is designed to capture the evolving community structures and identify the most influential nodes. More importantly, our framework leverages a community-level message passing during the learning process to balance local and global information propagation. Finally, we predict the popularity of the target item or online content based on the learned representations. Our experimental results based on three real-world datasets demonstrate that the proposed method achieves better performance than the baselines. Our method could not only model the changes in a user's preferences, but also capture how the communities evolve over time.
更多查看译文
关键词
Popularity Prediction,Dynamic Graph Learning,Community Detection
AI 理解论文
溯源树
样例
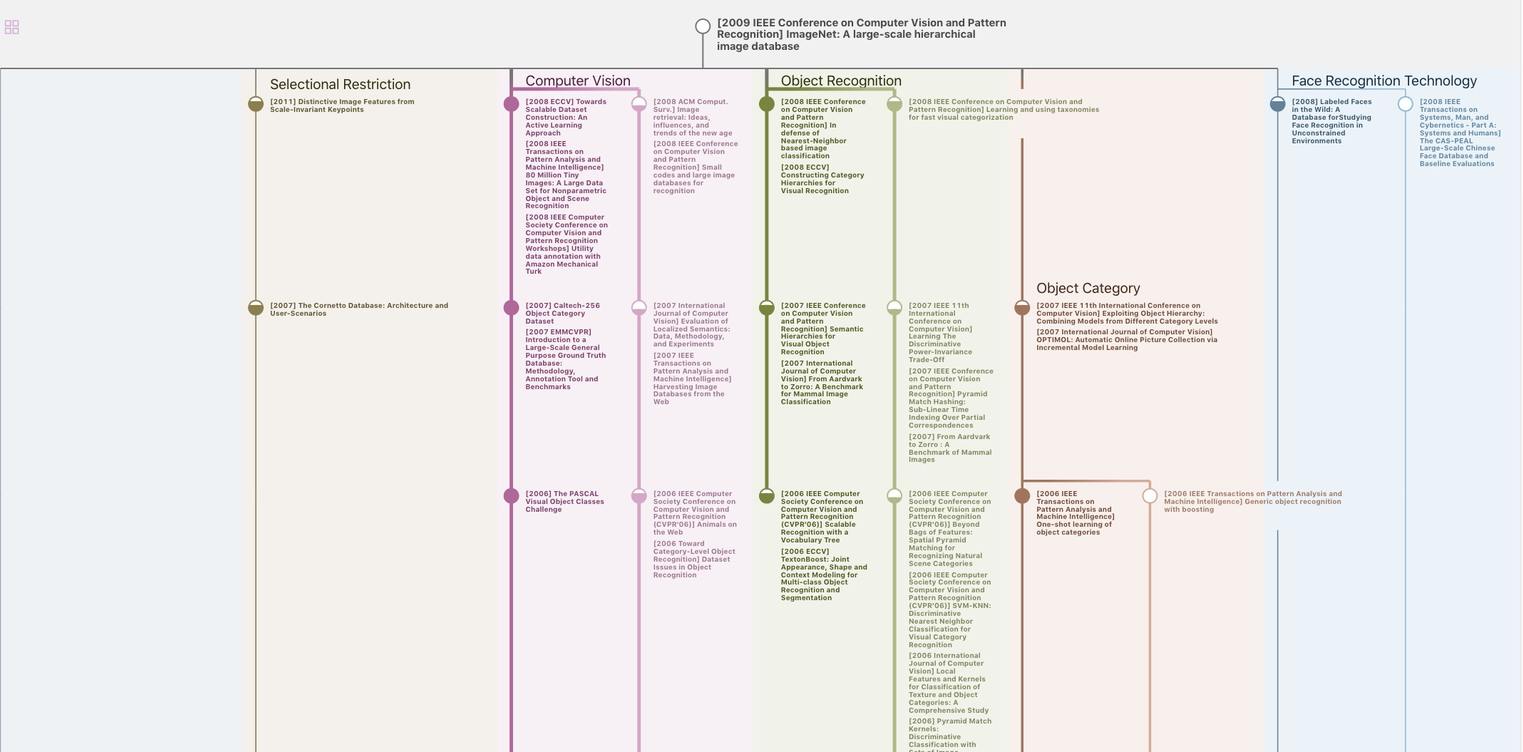
生成溯源树,研究论文发展脉络
Chat Paper
正在生成论文摘要