Do DL models and training environments have an impact on energy consumption?
CoRR(2023)
摘要
Current research in the computer vision field mainly focuses on improving
Deep Learning (DL) correctness and inference time performance. However, there
is still little work on the huge carbon footprint that has training DL models.
This study aims to analyze the impact of the model architecture and training
environment when training greener computer vision models. We divide this goal
into two research questions. First, we analyze the effects of model
architecture on achieving greener models while keeping correctness at optimal
levels. Second, we study the influence of the training environment on producing
greener models. To investigate these relationships, we collect multiple metrics
related to energy efficiency and model correctness during the models' training.
Then, we outline the trade-offs between the measured energy efficiency and the
models' correctness regarding model architecture, and their relationship with
the training environment. We conduct this research in the context of a computer
vision system for image classification. In conclusion, we show that selecting
the proper model architecture and training environment can reduce energy
consumption dramatically (up to 81.38
correctness. Also, we find evidence that GPUs should scale with the models'
computational complexity for better energy efficiency.
更多查看译文
关键词
energy
consumption,dl models,training environments
AI 理解论文
溯源树
样例
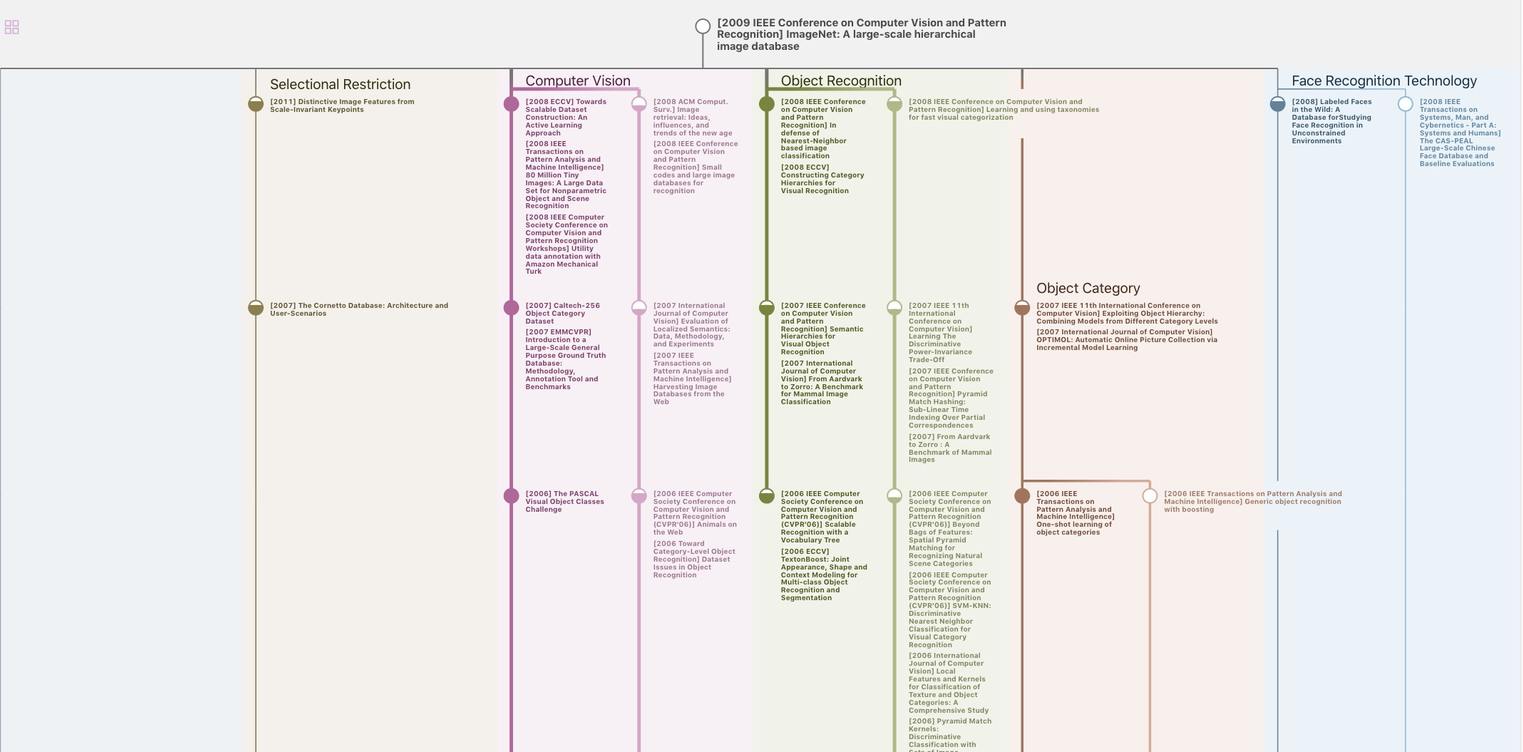
生成溯源树,研究论文发展脉络
Chat Paper
正在生成论文摘要