Fluorine spillover for ceria- vs silica-supported palladium nanoparticles: A MD study using machine learning potentials.
The Journal of chemical physics(2023)
摘要
Supported metallic nanoparticles play a central role in catalysis. However, predictive modeling is particularly challenging due to the structural and dynamic complexity of the nanoparticle and its interface with the support, given that the sizes of interest are often well beyond those accessible via traditional ab initio methods. With recent advances in machine learning, it is now feasible to perform MD simulations with potentials retaining near-density-functional theory (DFT) accuracy, which can elucidate the growth and relaxation of supported metal nanoparticles, as well as reactions on those catalysts, at temperatures and time scales approaching those relevant to experiments. Furthermore, the surfaces of the support materials can also be modeled realistically through simulated annealing to include effects such as defects and amorphous structures. We study the adsorption of fluorine atoms on ceria and silica supported palladium nanoparticles using machine learning potential trained by DFT data using the DeePMD framework. We show defects on ceria and Pd/ceria interfaces are crucial for the initial adsorption of fluorine, while the interplay between Pd and ceria and the reverse oxygen migration from ceria to Pd control spillover of fluorine from Pd to ceria at later stages. In contrast, silica supports do not induce fluorine spillover from Pd particles.
更多查看译文
关键词
palladium nanoparticles,fluorine,silica-supported
AI 理解论文
溯源树
样例
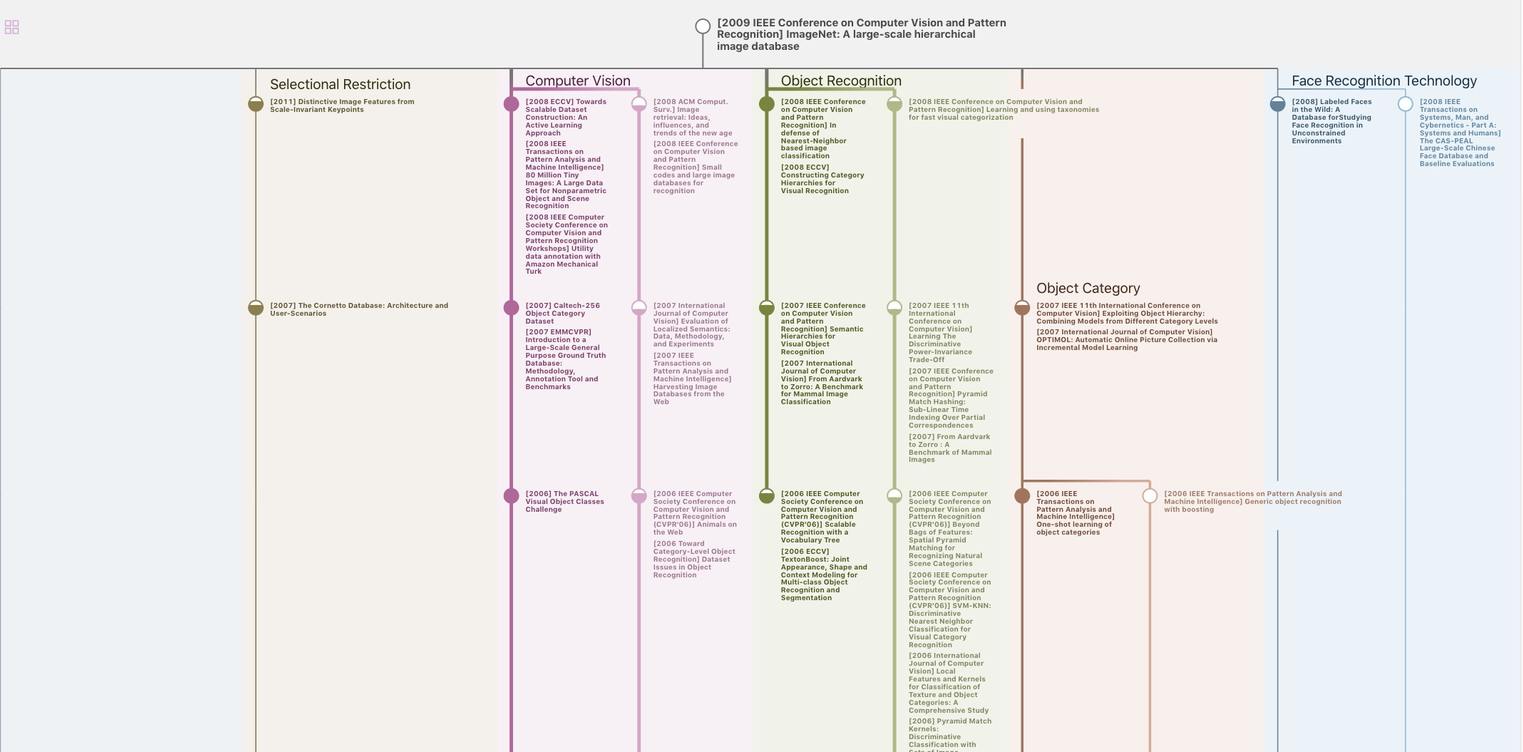
生成溯源树,研究论文发展脉络
Chat Paper
正在生成论文摘要