Neural representation dynamics reveal computational principles of cognitive task learning.
bioRxiv : the preprint server for biology(2023)
摘要
During cognitive task learning, neural representations must be rapidly constructed for novel task performance, then optimized for robust practiced task performance. How the geometry of neural representations changes to enable this transition from novel to practiced performance remains unknown. We hypothesized that practice involves a shift from compositional representations (task-general activity patterns that can be flexibly reused across tasks) to conjunctive representations (task-specific activity patterns specialized for the current task). Functional MRI during learning of multiple complex tasks substantiated this dynamic shift from compositional to conjunctive representations, which was associated with reduced cross-task interference (via pattern separation) and behavioral improvement. Further, we found that conjunctions originated in subcortex (hippocampus and cerebellum) and slowly spread to cortex, extending multiple memory systems theories to encompass task representation learning. The formation of conjunctive representations hence serves as a computational signature of learning, reflecting cortical-subcortical dynamics that optimize task representations in the human brain.
更多查看译文
关键词
neural representation dynamics,cognitive task,computational principles,learning
AI 理解论文
溯源树
样例
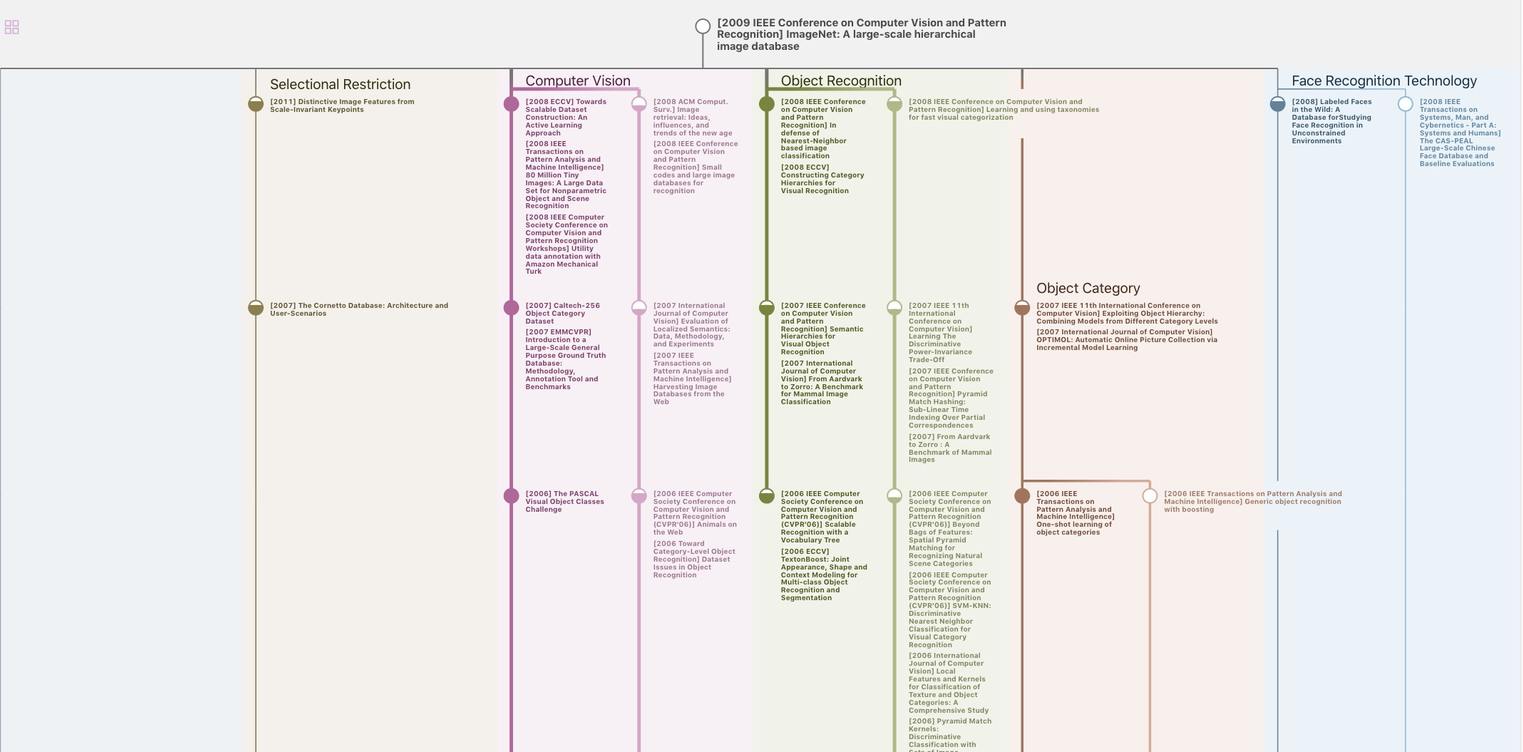
生成溯源树,研究论文发展脉络
Chat Paper
正在生成论文摘要