Context-aware lightweight remote-sensing image super-resolution network.
Frontiers in neurorobotics(2023)
摘要
In recent years, remote-sensing image super-resolution (RSISR) methods based on convolutional neural networks (CNNs) have achieved significant progress. However, the limited receptive field of the convolutional kernel in CNNs hinders the network's ability to effectively capture long-range features in images, thus limiting further improvements in model performance. Additionally, the deployment of existing RSISR models to terminal devices is challenging due to their high computational complexity and large number of parameters. To address these issues, we propose a Context-Aware Lightweight Super-Resolution Network (CALSRN) for remote-sensing images. The proposed network primarily consists of Context-Aware Transformer Blocks (CATBs), which incorporate a Local Context Extraction Branch (LCEB) and a Global Context Extraction Branch (GCEB) to explore both local and global image features. Furthermore, a Dynamic Weight Generation Branch (DWGB) is designed to generate aggregation weights for global and local features, enabling dynamic adjustment of the aggregation process. Specifically, the GCEB employs a Swin Transformer-based structure to obtain global information, while the LCEB utilizes a CNN-based cross-attention mechanism to extract local information. Ultimately, global and local features are aggregated using the weights acquired from the DWGB, capturing the global and local dependencies of the image and enhancing the quality of super-resolution reconstruction. The experimental results demonstrate that the proposed method is capable of reconstructing high-quality images with fewer parameters and less computational complexity compared with existing methods.
更多查看译文
关键词
convolutional neural network, transformer, remote-sensing image super-resolution, lightweight network, context-aware
AI 理解论文
溯源树
样例
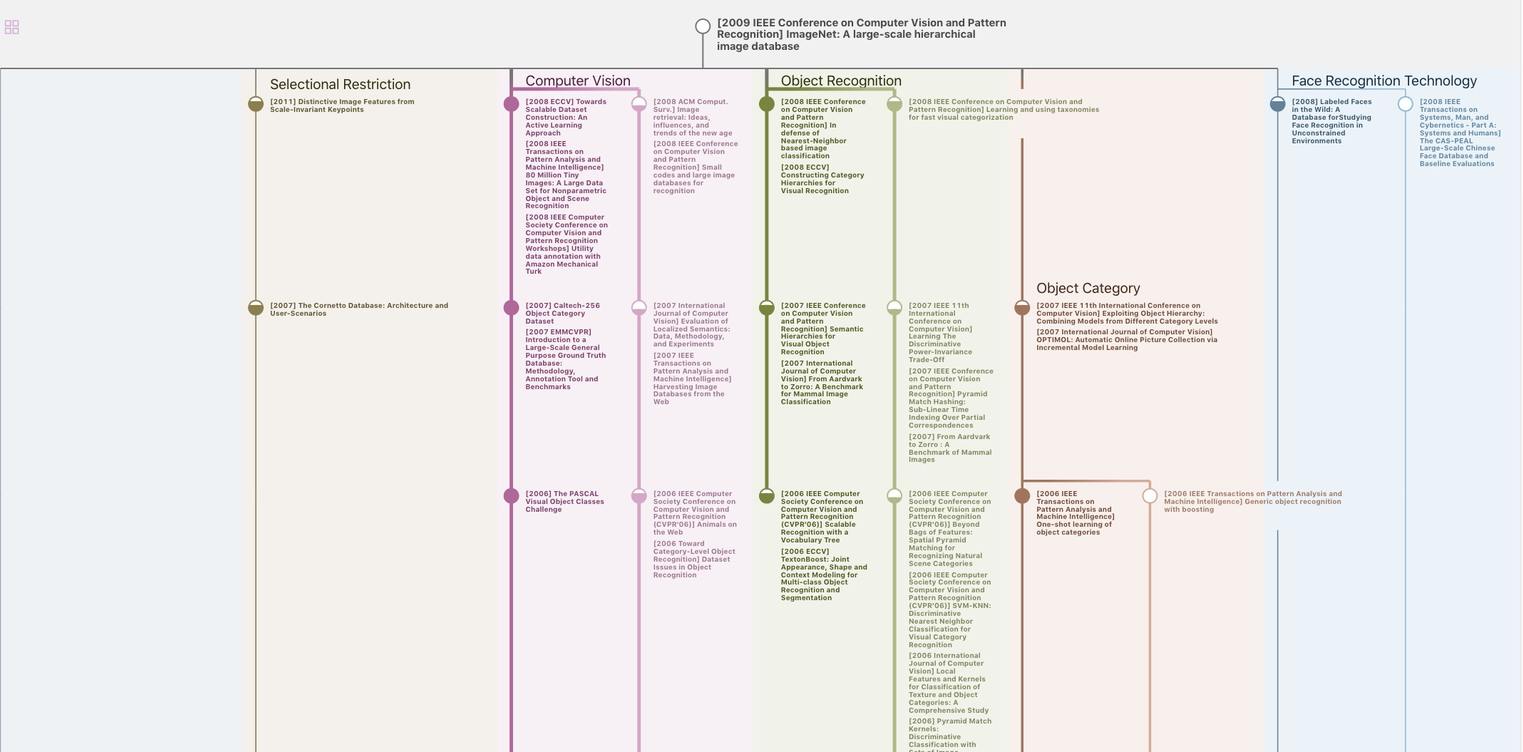
生成溯源树,研究论文发展脉络
Chat Paper
正在生成论文摘要