Use of high-speed angiography HSA-derived boundary conditions and Physics Informed Neural Networks (PINNs) for comprehensive estimation of neurovascular hemodynamics.
Proceedings of SPIE--the International Society for Optical Engineering(2023)
摘要
Purpose:Physics-informed neural networks (PINNs) and computational fluid dynamics (CFD) have both demonstrated an ability to derive accurate hemodynamics if boundary conditions (BCs) are known. Unfortunately, patient-specific BCs are often unknown, and assumptions based upon previous investigations are used instead. High speed angiography (HSA) may allow extraction of these BCs due to the high temporal fidelity of the modality. We propose to investigate whether PINNs using convection and Navier-Stokes equations with BCs derived from HSA data may allow for extraction of accurate hemodynamics in the vasculature.
Materials and Methods:Imaging data generated from
in vitroResults:Imaging-based PINNs show an ability to capture such hemodynamic phenomena as vortices in aneurysms and regions of rapid transience, such as outlet vessel blood flow within a carotid artery bifurcation phantom. These networks work best with small solution spaces and high temporal resolution of the input angiographic data, meaning HSA image sequences represent an ideal medium for such solution spaces.
Conclusions:The study shows the feasibility of obtaining patient-specific velocity and pressure fields using an assumption-free data driven approach based purely on governing physical equations and imaging data.
更多查看译文
AI 理解论文
溯源树
样例
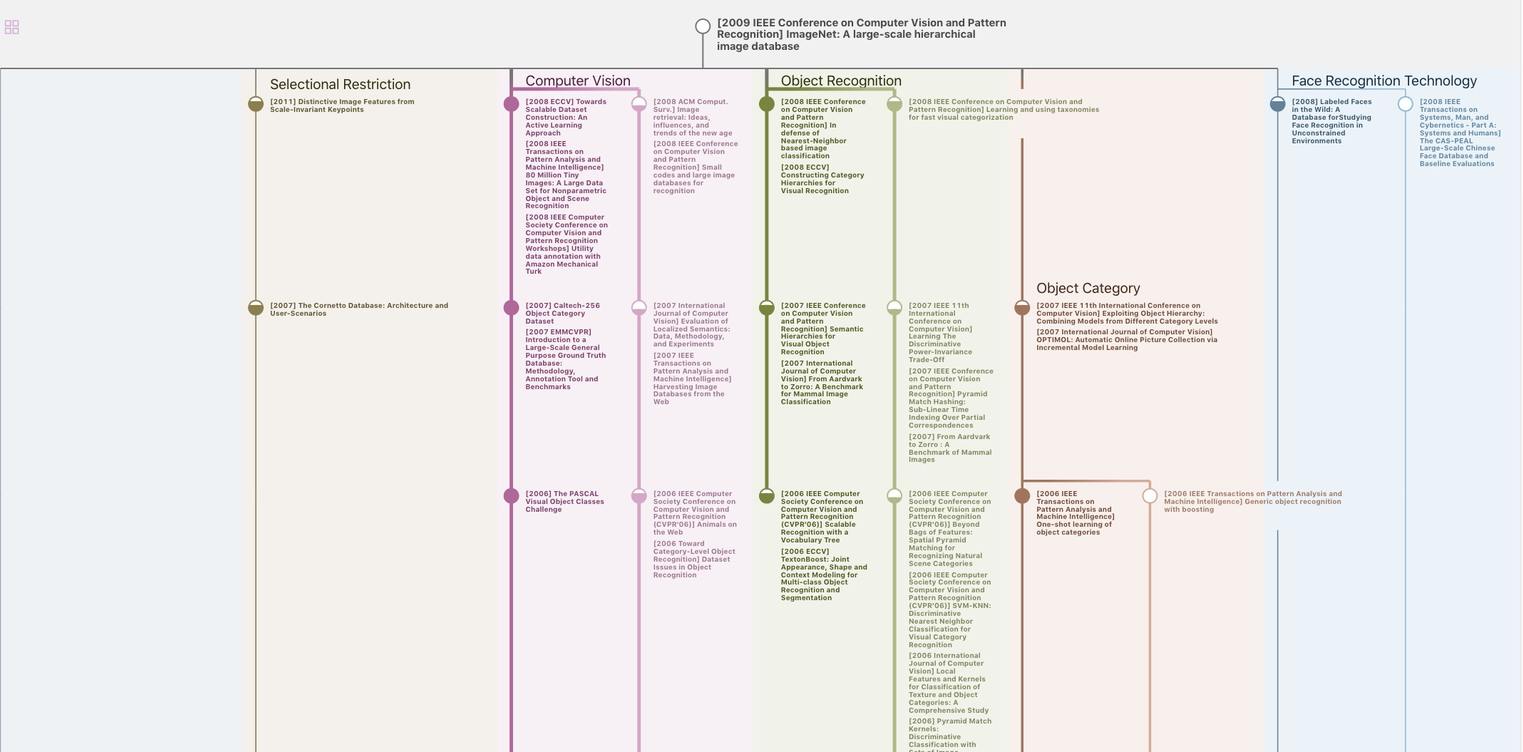
生成溯源树,研究论文发展脉络
Chat Paper
正在生成论文摘要