Use of Natural Language Processing of Patient-Initiated Electronic Health Record Messages to Identify Patients With COVID-19 Infection
JAMA network open(2023)
摘要
Importance Natural language processing (NLP) has the potential to enable faster treatment access by reducing clinician response time and improving electronic health record (EHR) efficiency. Objective To develop an NLP model that can accurately classify patient-initiated EHR messages and triage COVID-19 cases to reduce clinician response time and improve access to antiviral treatment. Design, Setting, and Participants This retrospective cohort study assessed development of a novel NLP framework to classify patient-initiated EHR messages and subsequently evaluate the model's accuracy. Included patients sent messages via the EHR patient portal from 5 Atlanta, Georgia, hospitals between March 30 and September 1, 2022. Assessment of the model's accuracy consisted of manual review of message contents to confirm the classification label by a team of physicians, nurses, and medical students, followed by retrospective propensity score-matched clinical outcomes analysis. Exposure Prescription of antiviral treatment for COVID-19. Main Outcomes and Measures The 2 primary outcomes were (1) physician-validated evaluation of the NLP model's message classification accuracy and (2) analysis of the model's potential clinical effect via increased patient access to treatment. The model classified messages into COVID-19-other (pertaining to COVID-19 but not reporting a positive test), COVID-19-positive (reporting a positive at-home COVID-19 test result), and non-COVID-19 (not pertaining to COVID-19). Results Among 10172 patients whose messages were included in analyses, the mean (SD) age was 58 (17) years; 6509 patients (64.0%) were women and 3663 (36.0%) were men. In terms of race and ethnicity, 2544 patients (25.0%) were African American or Black, 20 (0.2%) were American Indian or Alaska Native, 1508 (14.8%) were Asian, 28 (0.3%) were Native Hawaiian or other Pacific Islander, 5980 (58.8%) were White, 91 (0.9%) were more than 1 race or ethnicity, and 1 (0.01%) chose not to answer. The NLP model had high accuracy and sensitivity, with a macro F1 score of 94% and sensitivity of 85% for COVID-19-other, 96% for COVID-19-positive, and 100% for non-COVID-19 messages. Among the 3048 patient-generated messages reporting positive SARS-CoV-2 test results, 2982 (97.8%) were not documented in structured EHR data. Mean (SD) message response time for COVID-19-positive patients who received treatment (364.10 [784.47] minutes) was faster than for those who did not (490.38 [1132.14] minutes; P=.03). Likelihood of antiviral prescription was inversely correlated with message response time (odds ratio, 0.99 [95% CI, 0.98-1.00]; P=.003). Conclusions and Relevance In this cohort study of 2982 COVID-19-positive patients, a novel NLP model classified patient-initiated EHR messages reporting positive COVID-19 test results with high sensitivity. Furthermore, when responses to patient messages occurred faster, patients were more likely to receive antiviral medical prescription within the 5-day treatment window. Although additional analysis on the effect on clinical outcomes is needed, these findings represent a possible use case for integration of NLP algorithms into clinical care.
更多查看译文
关键词
natural language processing,infection,patients,patient-initiated
AI 理解论文
溯源树
样例
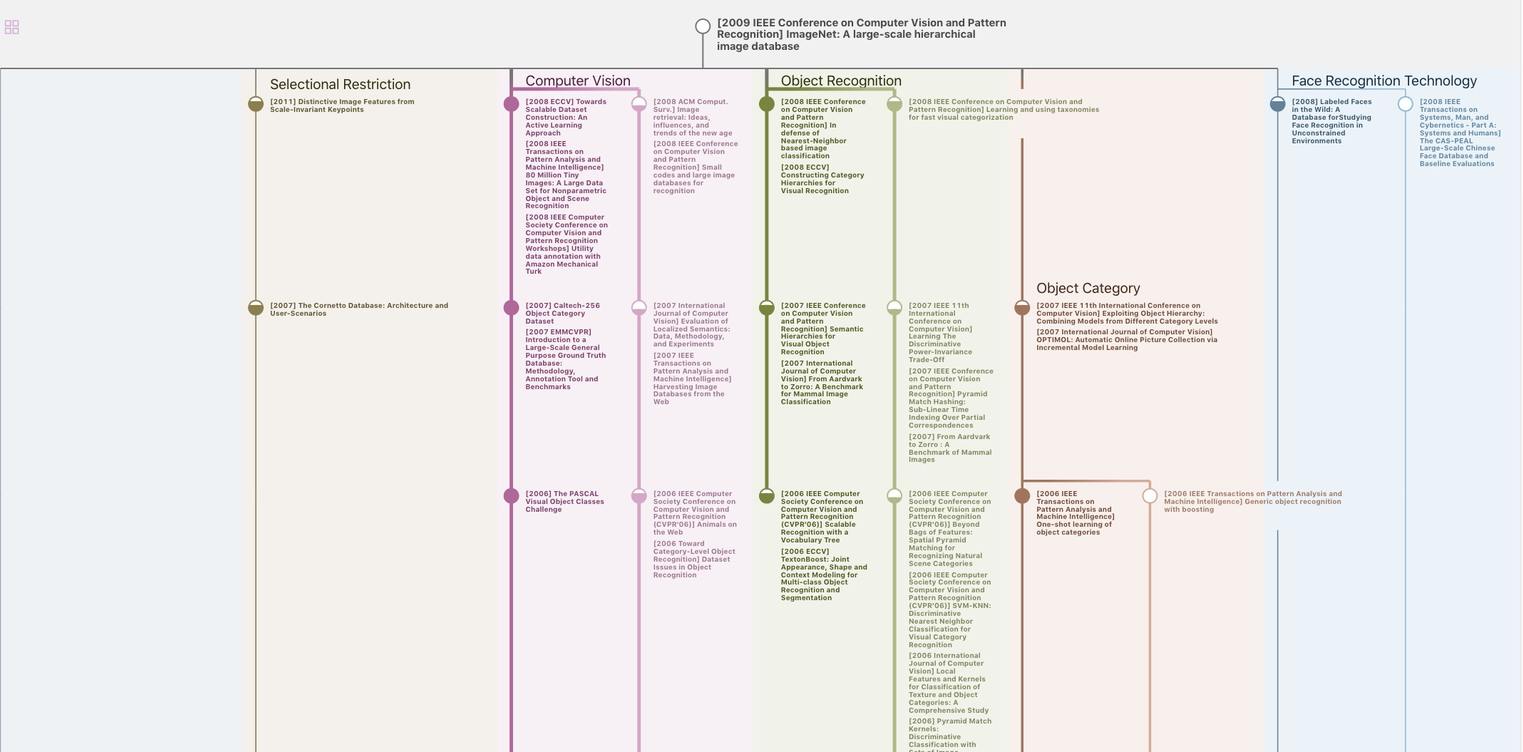
生成溯源树,研究论文发展脉络
Chat Paper
正在生成论文摘要