MOKPE: drug–target interaction prediction via manifold optimization based kernel preserving embedding
BMC bioinformatics(2023)
摘要
Background In many applications of bioinformatics, data stem from distinct heterogeneous sources. One of the well-known examples is the identification of drug–target interactions (DTIs), which is of significant importance in drug discovery. In this paper, we propose a novel framework, manifold optimization based kernel preserving embedding (MOKPE), to efficiently solve the problem of modeling heterogeneous data. Our model projects heterogeneous drug and target data into a unified embedding space by preserving drug–target interactions and drug–drug, target–target similarities simultaneously. Results We performed ten replications of ten-fold cross validation on four different drug–target interaction network data sets for predicting DTIs for previously unseen drugs. The classification evaluation metrics showed better or comparable performance compared to previous similarity-based state-of-the-art methods. We also evaluated MOKPE on predicting unknown DTIs of a given network. Our implementation of the proposed algorithm in R together with the scripts that replicate the reported experiments is publicly available at https://github.com/ocbinatli/mokpe .
更多查看译文
关键词
Drug–target interaction prediction, Drug repurposing, Manifold optimization, Kernel methods, Machine learning
AI 理解论文
溯源树
样例
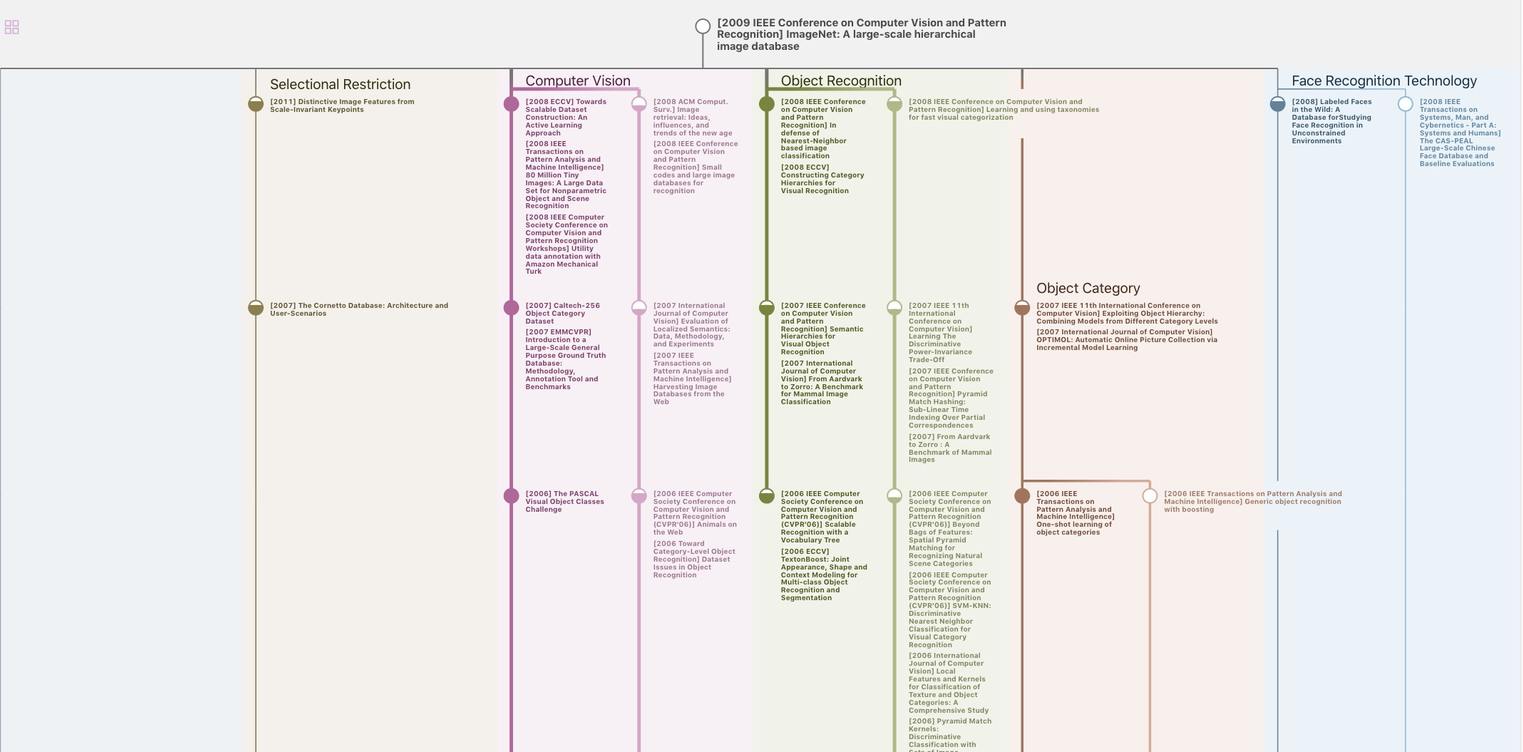
生成溯源树,研究论文发展脉络
Chat Paper
正在生成论文摘要