Tipping Point Detection Using Reservoir Computing.
Research (Washington, D.C.)(2023)
摘要
Detection in high fidelity of tipping points, the emergence of which is often induced by invisible changes in internal structures or/and external interferences, is paramountly beneficial to understanding and predicting complex dynamical systems (CDSs). Detection approaches, which have been fruitfully developed from several perspectives (e.g., statistics, dynamics, and machine learning), have their own advantages but still encounter difficulties in the face of high-dimensional, fluctuating datasets. Here, using the reservoir computing (RC), a recently notable, resource-conserving machine learning method for reconstructing and predicting CDSs, we articulate a model-free framework to accomplish the detection only using the time series observationally recorded from the underlying unknown CDSs. Specifically, we encode the information of the CDS in consecutive time durations of finite length into the weights of the readout layer in an RC, and then we use the learned weights as the dynamical features and establish a mapping from these features to the system's changes. Our designed framework can not only efficiently detect the changing positions of the system but also accurately predict the intensity change as the intensity information is available in the training data. We demonstrate the efficacy of our supervised framework using the dataset produced by representative physical, biological, and real-world systems, showing that our framework outperforms those traditional methods on the short-term data produced by the time-varying or/and noise-perturbed systems. We believe that our framework, on one hand, complements the major functions of the notable RC intelligent machine and, on the other hand, becomes one of the indispensable methods for deciphering complex systems.
更多查看译文
关键词
point detection,computing
AI 理解论文
溯源树
样例
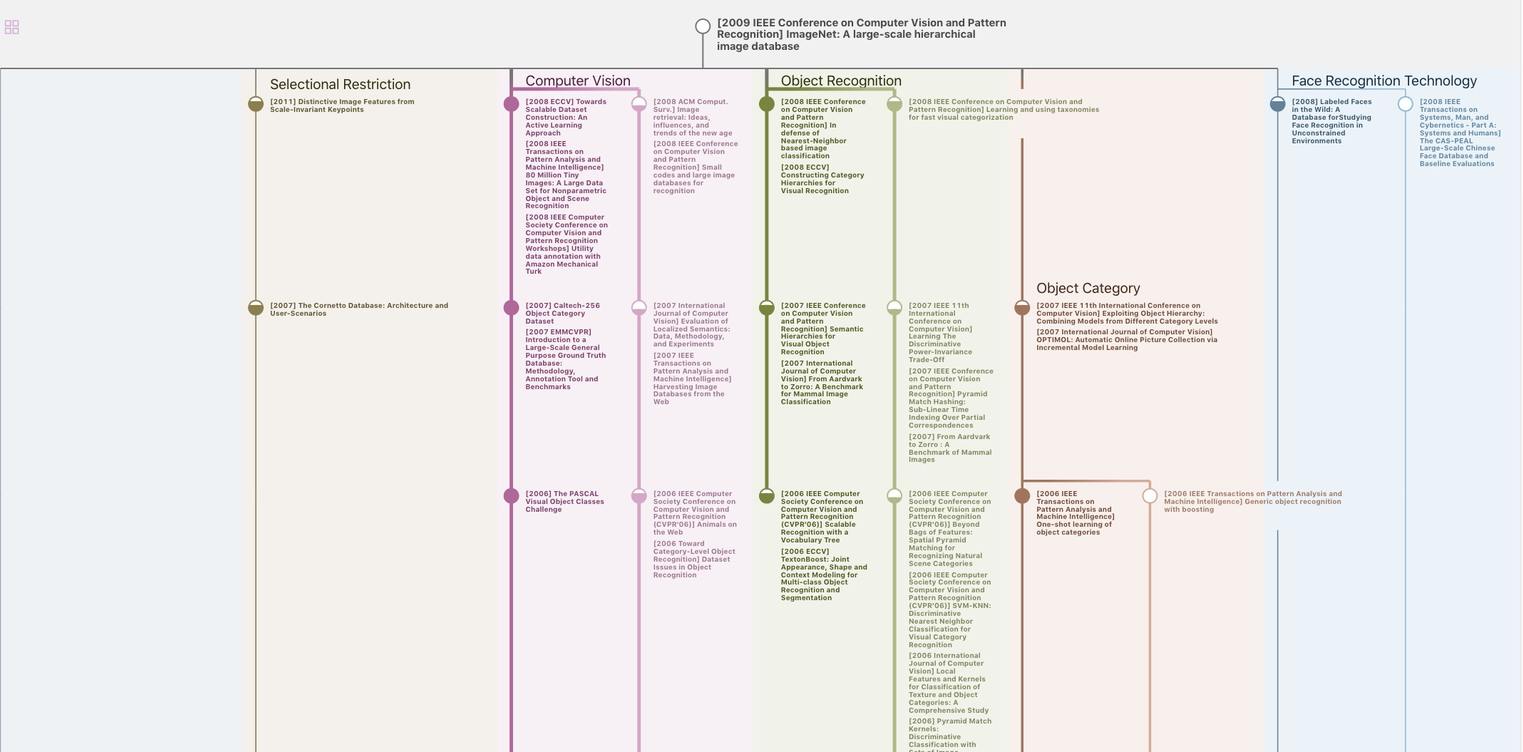
生成溯源树,研究论文发展脉络
Chat Paper
正在生成论文摘要