Identifying Coarse-Grained Representations for Electronic Predictions
Journal of chemical theory and computation(2023)
摘要
Coarse-grained (CG) simulations are an important computationaltool in chemistry and materials science. Recently, systematic "bottom-up"CG models have been introduced to capture electronic structure variationsof molecules and polymers at the CG resolution. However, the performanceof these models is limited by the ability to select reduced representationsthat preserve electronic structure information, which remains a challenge.We propose two methods for (i) identifying important electronicallycoupled atomic degrees of freedom and (ii) scoring the efficacy ofCG representations used in conjunction with CG electronic predictions.The first method is a physically motivated approach that incorporatesnuclear vibrations and electronic structure derived from simple quantumchemical calculations. We complement this physically motivated approachwith a machine learning technique based on the marginal contributionof nuclear degrees of freedom to electronic prediction accuracy usingan equivariant graph neural network. By integrating these two approaches,we can both identify critical electronically coupled atomic coordinatesand score the efficacy of arbitrary CG representations for makingelectronic predictions. We leverage this capability to make a connectionbetween optimized CG representations and the future potential for"bottom-up" development of simplified model Hamiltoniansincorporating nonlinear vibrational modes.
更多查看译文
关键词
representations,coarse-grained
AI 理解论文
溯源树
样例
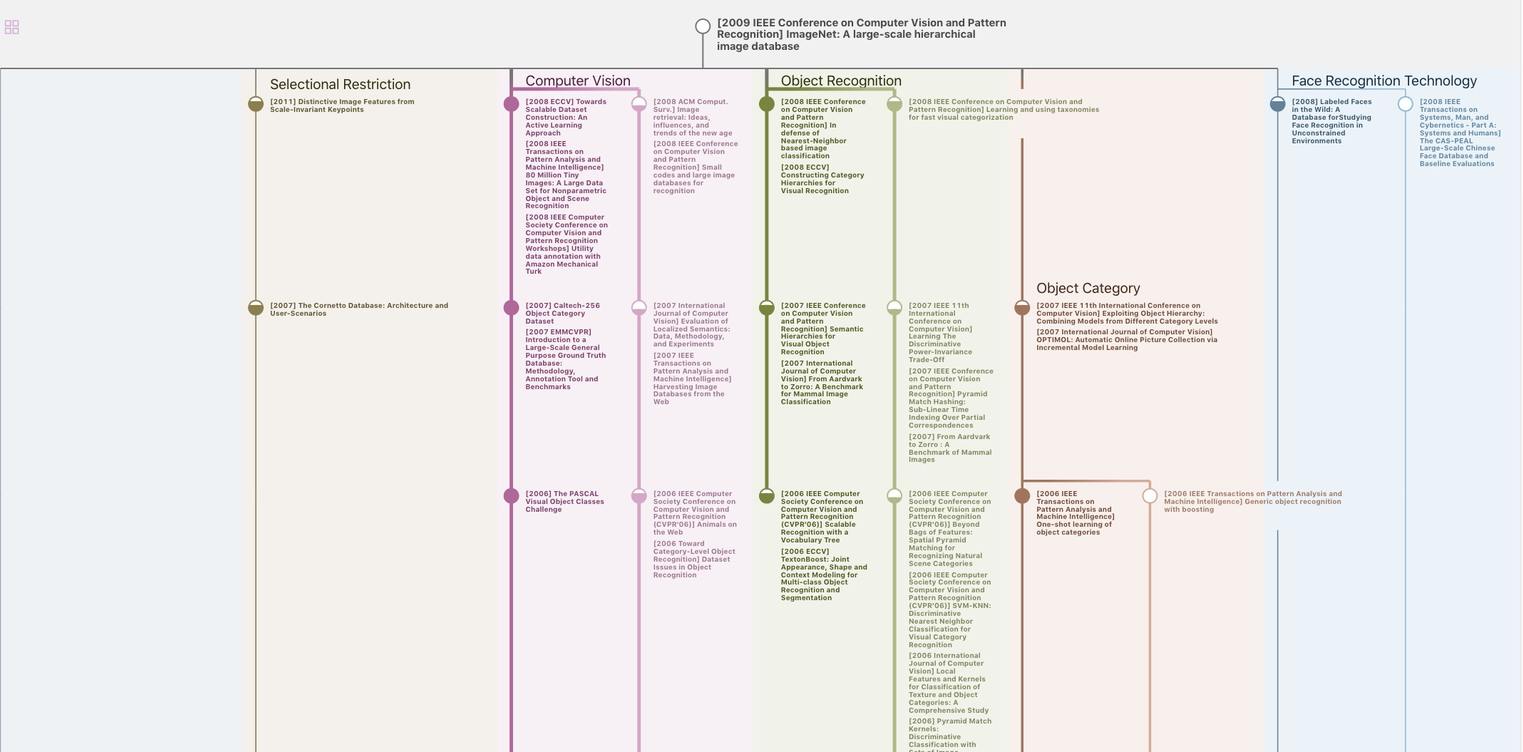
生成溯源树,研究论文发展脉络
Chat Paper
正在生成论文摘要