Position statement on clinical evaluation of imaging AI.
The Lancet. Digital health(2023)
摘要
Governments and medical associations across the world, including the US Food and Drug Administration, the UK Medicines and Healthcare products Regulatory Agency, the Royal College of Radiologists, and the European Society of Radiology, believe the advent of health technologies associated with artificial intelligence (AI) will be the most radical change in how medical care is delivered in our lifetime.1Royal College of RadiologistsRCR position statement on artificial intelligence.http://www.rcr.ac.uk/posts/rcr-position-statement-artificial-intelligenceDate: 2018Date accessed: March 6, 2023Google Scholar, 2FDAMHRAHealth CanadaGood machine learning practice for medical device development: guiding principles.https://www.fda.gov/medical-devices/software-medical-device-samd/good-machine-learning-practice-medical-device-development-guiding-principlesDate: 2021Date accessed: March 6, 2023Google Scholar At a time of unprecedented demand for medical imaging, when hospitals struggle with staffing shortages, AI tools could provide a solution. Traditionally, the basis of medical image interpretation relies on a visual, mainly qualitative, assessment, which is dependent on the observer's level of training and experience. For example, in oncological practice, contouring a three-dimensional volume of interest, such as a tumour or adjacent structures, is a key step in planning radiotherapy treatment. When done manually, this process is time-consuming and subject to inter-observer variation. In the last decade, advances in high-performance computing have transformed medical images into high-dimensional data, which can be digitally mined to extract added insights. These advancements have coincided with the development of sophisticated AI algorithms that, in contrast to traditional radiology, do tasks in an automated, almost-instantaneous, and highly consistent manner. AI tools excel at medical image analysis—they can automatically detect complex anomalous patterns in radiological images and can provide quantitative information on disease. In clinical research settings, these tools are already being applied in screening, detection of disease, lesion classification, diagnosis, assessment of prognosis, advancing our understanding of basic disease processes, and improving our accuracy of assessing treatment responses.3McCague C Ramlee S Reinius M et al.Introduction to radiomics for a clinical audience.Clin Radiol. 2023; 78: 83-98Summary Full Text Full Text PDF PubMed Scopus (3) Google Scholar However, these technologies might not be an instant panacea, as the translation from research to implementation in a clinical setting is a complex technical, ethical, and regulatory challenge. The most basic of these issues pertains to the validation of an AI tool's performance at clinical tasks. In research, an AI tool's performance is quantitatively evaluated by use of statistical metrics of agreement between the AI algorithm and the ground truth (which is usually generated by a human). Quantitative metrics are objective, often simple to use via statistical software, and do not require additional clinical expertise. Concerns with this quantitative-metrics-only approach exist. First, a quantitative-metrics-only approach to performance evaluation might not give a clear indication of the performance of an AI algorithm in clinical practice; in some cases this evaluation might underestimate AI algorithms with genuine clinical value and in other cases, most worryingly, it might overestimate their clinical utility. This misinterpretation can lead to vast amounts of developer time being wasted, producing tools with no potential for clinical translation.4Roberts M Driggs D Thorpe M et al.Common pitfalls and recommendations for using machine learning to detect and prognosticate for COVID-19 using chest radiographs and CT scans.Nat Mach Intell. 2021; 3: 199-217Crossref Scopus (406) Google Scholar Second, the quoted quantitative performance is often assessed on private, retrospective, and sometimes in-silico datasets. Third, the health-care professionals’ involvement in the application of the quantitative-metrics-only approach is passive, restricted only to the generation of the ground truth that the AI performance is quantitatively compared against. These features of the quantitative-metrics-only-based approach prevent transparency and lead to an absence of trust from health-care professionals, which ultimately affects the trust of patients and the general public in these devices. Translation of AI-based contouring tools—also known as segmentation tools—from research to a clinical setting is one such example. A robust and reliable automated segmentation tool would have clinical utility by automatically segmenting medical images, which is an essential and time-consuming step in radiotherapy planning and the development of prognostic radiomic biomarkers. Currently, quantitative metrics, including the overlap-based dice similarity coefficient, are the most common methods of measuring the performance of AI-based segmentation tools. However, this approach does not identify or classify the errors an algorithm might be making. This lack of transparency could mask serious errors, or allow poor algorithmic performance to be concealed.5Reinke A Eisenmann M Onogur S et al.How to exploit weaknesses in biomedical challenge design and organization.in: Frangi AF Schnabel JA Davatzikos C Medical image computing and computer assisted intervention—MICCAI 2018: 21st International Conference, Granada, Spain, September 16–20, 2018, proceedings, part IV. Springer International Publishing, Cham2018: 388-395Crossref Scopus (23) Google Scholar, 6Heller N Isensee F Maier-Hein KH et al.The state of the art in kidney and kidney tumor segmentation in contrast-enhanced CT imaging: results of the KiTS19 challenge.Med Image Anal. 2021; 67101821Summary Full Text Full Text PDF PubMed Scopus (150) Google Scholar Additionally, most research efforts focus on developing algorithms that produce high dice similarity coefficient scores, rather than creating a clinically relevant and usable segmentation tool. Some aspects of clinical use, (eg, how well an AI tool collaborates with a clinician to allow faster, high-quality segmentations) are not considered in the current quantitative-metrics-only-based assessment frameworks. Finally, many groups developing segmentation tools do not have clinical expertise, which means systematic errors obvious to a domain expert might be overlooked. How should we better validate imaging AI tools, increase trust in their performance, and ultimately aid adoption into clinical practice? We postulate that an essential part of the answer is to involve health-care professionals in the development and validation of AI-based tools in an active, well-structured, and reproducible manner. Research in other areas of AI translation has suggested that involving domain experts (whose work is affected by an algorithm) in the early development of AI tools increases trust in the tools.7Thomas RL Uminsky D Reliance on metrics is a fundamental challenge for AI.Patterns (N Y). 2022; 3100476Google Scholar Additionally, combining the qualitative insights of these experts with appropriately chosen quantitative metrics8Maier-Hein L Reinke A Christodoulou E et al.Metrics reloaded: pitfalls and recommendations for image analysis validation. arXiv.https://arxiv.org/abs/2206.01653Date: 2022Google Scholar is a good way to establish utility and further build user's trust in the device.7Thomas RL Uminsky D Reliance on metrics is a fundamental challenge for AI.Patterns (N Y). 2022; 3100476Google Scholar CONSORT-AI and SPIRIT-AI have both highlighted the importance of aligning the development of AI-based interventions with actual clinical needs, so that they are better integrated into clinical practice. However, there is no clear guidance on how health-care practitioners should be involved in this process. The radiomic quality score and the checklist for artificial intelligence in medical imaging have improved the rigour and transparency of AI-based medical image analysis research, ensuring that studies are done with methodological soundness, and potential biases and limitations are appropriately addressed. However, neither checklist assesses whether a clinical domain expert was part of the research team during model creation.9Lambin P Leijenaar RTH Deist TM et al.Radiomics: the bridge between medical imaging and personalized medicine.Nat Rev Clin Oncol. 2017; 14: 749-762Crossref PubMed Scopus (2417) Google Scholar, 10Mongan J Moy L Kahn Jr, CE Checklist for artificial intelligence in medical imaging (CLAIM): a guide for authors and reviewers.Radiol Artif Intell. 2020; 2e200029Crossref Google Scholar We propose that future gold standard AI-based medical image analysis development must involve a clinical domain expert in an active role by default. When validating the performance of AI-based medical imaging tools, qualitative assessment by a health-care professional whose work will be affected by the tool should be combined with established quantitative metrics. This involvement will improve the developers’ understanding of the strengths and weaknesses of the tools, and aid clinician trust. To facilitate this validation, well-defined evaluation frameworks to standardise qualitative assessment and maximise feedback to the developers are required. These frameworks should be clearly structured, semiquantitative, and reproducible. They should contain a clear sampling strategy that is appropriate for the tool's clinical application and target population and should assess AI performance both in isolation and as an assistant to a health-care professional. The frameworks should be used before clinical implementation and frequently after implementation to ensure performance is maintained and protect against automation bias. The medical image analysis community, along with relevant interest groups and societies, should take the lead in developing frameworks to guide and structure the appraisal of AI tools by health-care professionals. This strategy will enable the adoption of safe, effective, and trustworthy AI technologies into the clinical workflow. MCO has received honoraria from GSK and AI for Global Goals, and is a co-founder and shareholder in 52North Health. FG has received consulting fees from Kherion, Alphabet, and Bayer, and honoraria from GE HealthCare;.GH receives a salary for his role as Chief Clinical Data Officer for Health Data Research UK. PMcL is Chief Safety Officer and a clinical evaluator for Change Healthcare. ES has received honoraria from GE HealthCare, and is a co-founder and shareholder in Lucida Medical. RW has received honoraria from GE HealthCare. All other authors declare no competing interests. Download .pdf (.22 MB) Help with pdf files Supplementary appendix
更多查看译文
AI 理解论文
溯源树
样例
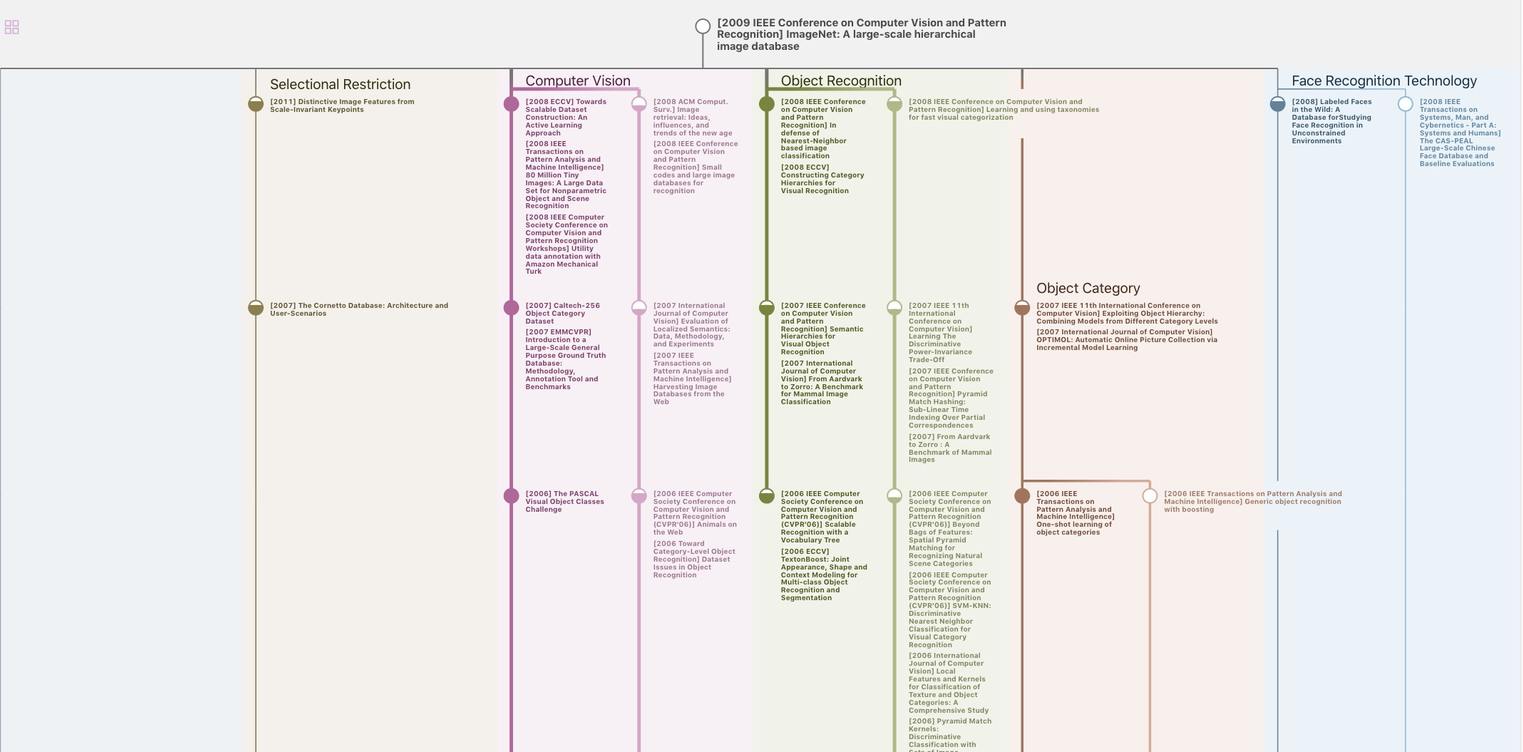
生成溯源树,研究论文发展脉络
Chat Paper
正在生成论文摘要