Untrained deep learning-based differential phase-contrast microscopy.
Optics letters(2023)
摘要
Quantitative differential phase-contrast (DPC) microscopy produces phase images of transparent objects based on a number of intensity images. To reconstruct the phase, in DPC microscopy, a linearized model for weakly scattering objects is considered; this limits the range of objects to be imaged, and requires additional measurements and complicated algorithms to correct for system aberrations. Here, we present a self-calibrated DPC microscope using an untrained neural network (UNN), which incorporates the nonlinear image formation model. Our method alleviates the restrictions on the object to be imaged and simultaneously reconstructs the complex object information and aberrations, without any training dataset. We demonstrate the viability of UNN-DPC microscopy through both numerical simulations and LED microscope-based experiments.
更多查看译文
关键词
learning-based,phase-contrast
AI 理解论文
溯源树
样例
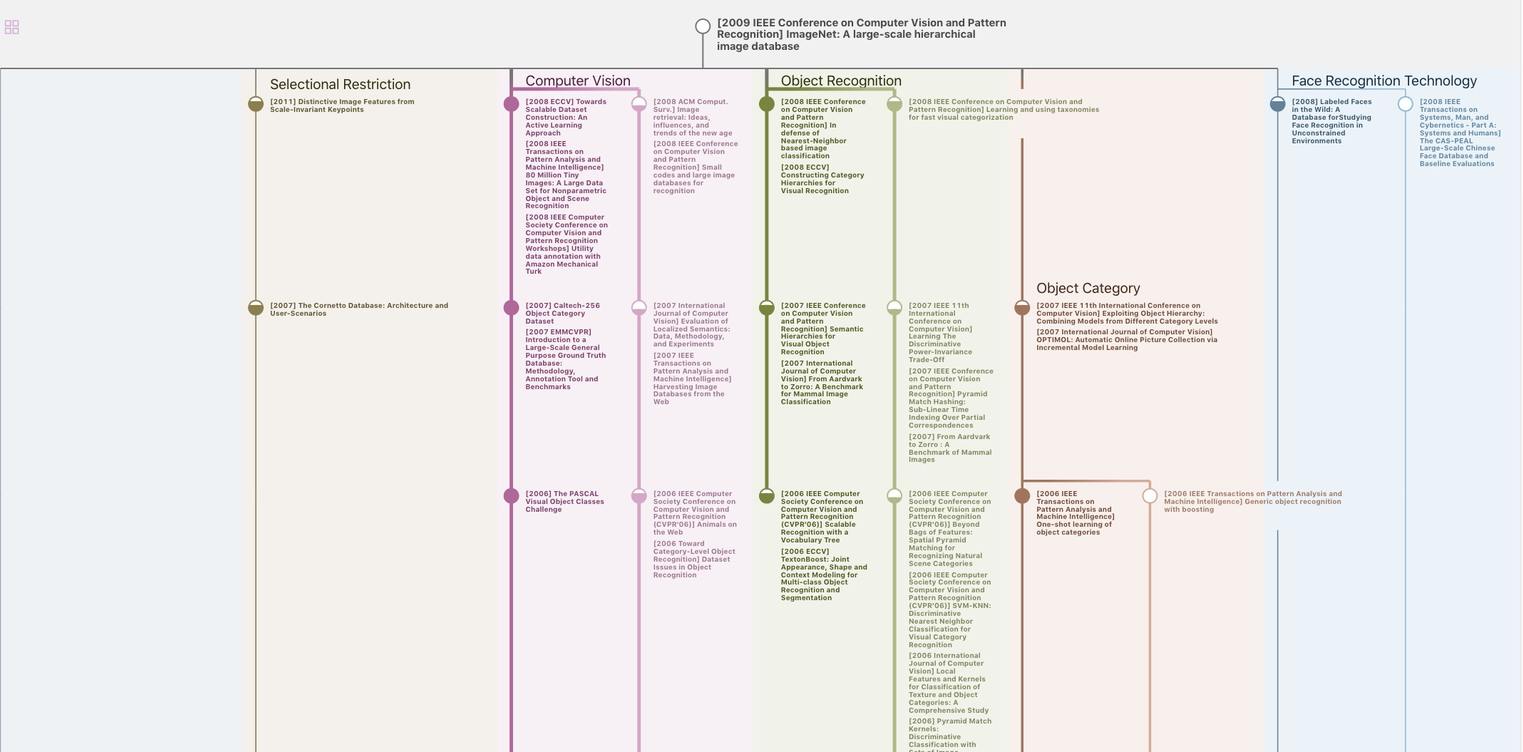
生成溯源树,研究论文发展脉络
Chat Paper
正在生成论文摘要