[Non-rigid registration for medical images based on deformable convolution and multi-scale feature focusing modules].
Sheng wu yi xue gong cheng xue za zhi = Journal of biomedical engineering = Shengwu yixue gongchengxue zazhi(2023)
摘要
Non-rigid registration plays an important role in medical image analysis. U-Net has been proven to be a hot research topic in medical image analysis and is widely used in medical image registration. However, existing registration models based on U-Net and its variants lack sufficient learning ability when dealing with complex deformations, and do not fully utilize multi-scale contextual information, resulting insufficient registration accuracy. To address this issue, a non-rigid registration algorithm for X-ray images based on deformable convolution and multi-scale feature focusing module was proposed. First, it used residual deformable convolution to replace the standard convolution of the original U-Net to enhance the expression ability of registration network for image geometric deformations. Then, stride convolution was used to replace the pooling operation of the downsampling operation to alleviate feature loss caused by continuous pooling. In addition, a multi-scale feature focusing module was introduced to the bridging layer in the encoding and decoding structure to improve the network model's ability of integrating global contextual information. Theoretical analysis and experimental results both showed that the proposed registration algorithm could focus on multi-scale contextual information, handle medical images with complex deformations, and improve the registration accuracy. It is suitable for non-rigid registration of chest X-ray images.
更多查看译文
关键词
deformable convolution,medical images,registration,focusing,[non-rigid,multi-scale
AI 理解论文
溯源树
样例
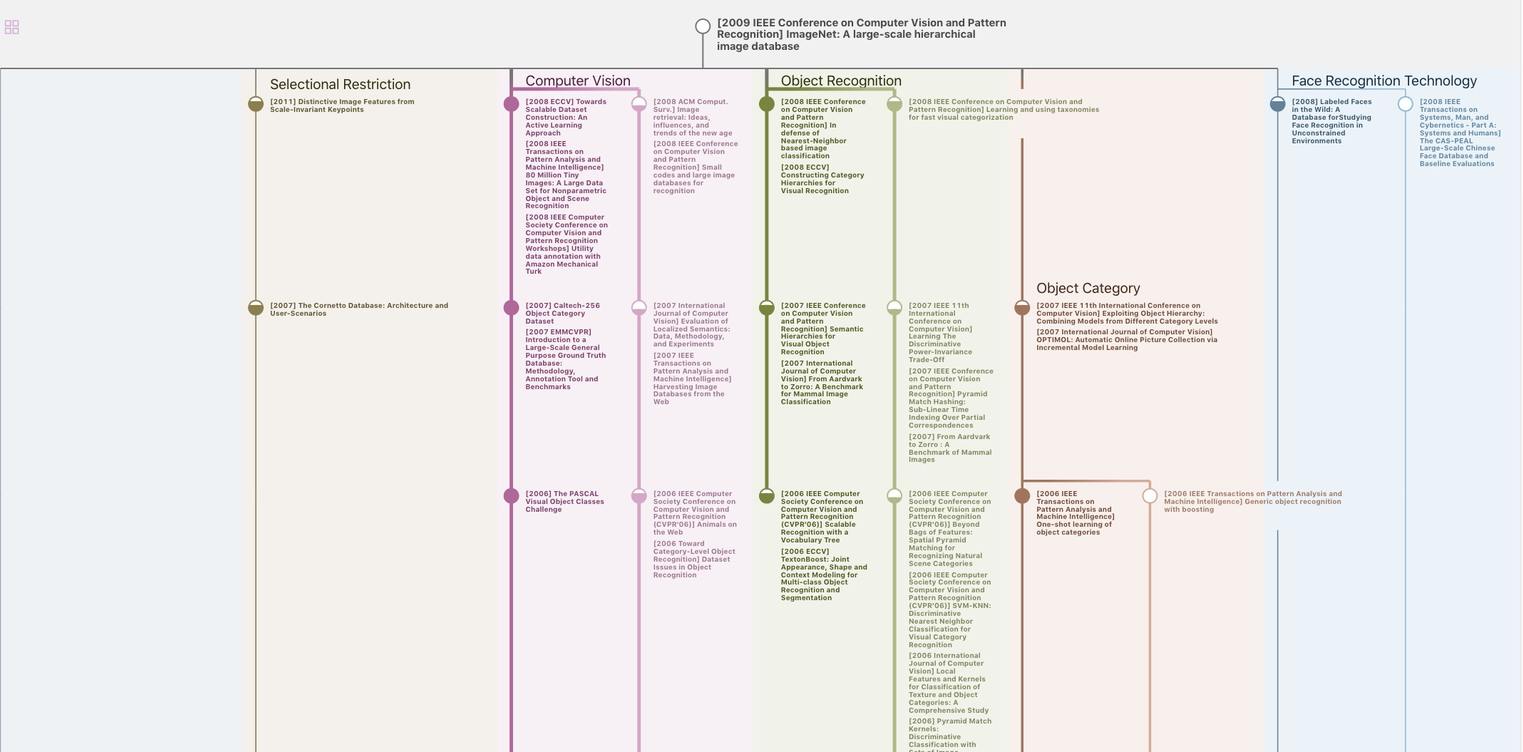
生成溯源树,研究论文发展脉络
Chat Paper
正在生成论文摘要