Transformer-Based Molecular Generative Model for Antiviral Drug Design
Journal of chemical information and modeling(2023)
摘要
Since the Simplified Molecular Input Line Entry System(SMILES)is oriented to the atomic-level representation of molecules and isnot friendly in terms of human readability and editable, however,IUPAC is the closest to natural language and is very friendly in termsof human-oriented readability and performing molecular editing, wecan manipulate IUPAC to generate corresponding new molecules and produceprogramming-friendly molecular forms of SMILES. In addition, antiviraldrug design, especially analogue-based drug design, is also more appropriateto edit and design directly from the functional group level of IUPACthan from the atomic level of SMILES, since designing analogues involvesaltering the R group only, which is closer to the knowledge-basedmolecular design of a chemist. Herein, we present a novel data-drivenself-supervised pretraining generative model called "TransAntivirus"to make select-and-replace edits and convert organic molecules intothe desired properties for design of antiviral candidate analogues.The results indicated that TransAntivirus is significantly superiorto the control models in terms of novelty, validity, uniqueness, anddiversity. TransAntivirus showed excellent performance in the designand optimization of nucleoside and non-nucleoside analogues by chemicalspace analysis and property prediction analysis. Furthermore, to validatethe applicability of TransAntivirus in the design of antiviral drugs,we conducted two case studies on the design of nucleoside analoguesand non-nucleoside analogues and screened four candidate lead compounds against anticoronavirus disease (COVID-19). Finally, we recommendthis framework for accelerating antiviral drug discovery.
更多查看译文
关键词
molecular generative model,transformer-based
AI 理解论文
溯源树
样例
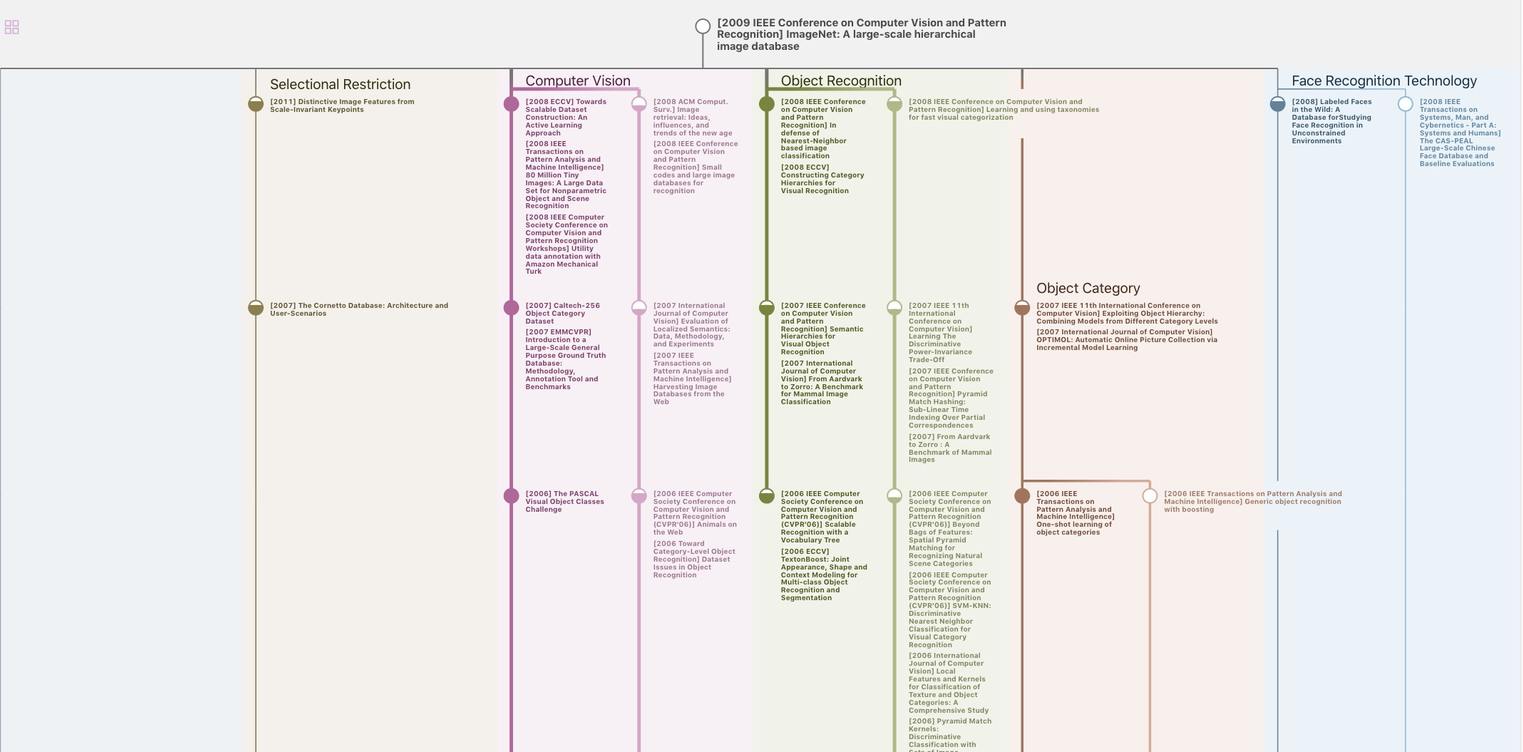
生成溯源树,研究论文发展脉络
Chat Paper
正在生成论文摘要