Improving breast tumor segmentation via shape-wise prior-guided information on cone-beam breast CT images
Physics in medicine and biology(2023)
摘要
Objective. Due to the blurry edges and uneven shape of breast tumors, breast tumor segmentation can be a challenging task. Recently, deep convolution networks based approaches achieve satisfying segmentation results. However, the learned shape information of breast tumors might be lost owing to the successive convolution and down-sampling operations, resulting in limited performance.Approach. To this end, we propose a novel shape-guided segmentation (SGS) framework that guides the segmentation networks to be shape-sensitive to breast tumors by prior shape information. Different from usual segmentation networks, we guide the networks to model shape-shared representation with the assumption that shape information of breast tumors can be shared among samples. Specifically, on the one hand, we propose a shape guiding block (SGB) to provide shape guidance through a superpixel pooling-unpooling operation and attention mechanism. On the other hand, we further introduce a shared classification layer (SCL) to avoid feature inconsistency and additional computational costs. As a result, the proposed SGB and SCL can be effortlessly incorporated into mainstream segmentation networks (e.g. UNet) to compose the SGS, facilitating compact shape friendly representation learning.Main results. Experiments conducted on a private dataset and a public dataset demonstrate the effectiveness of the SGS compared to other advanced methods.Significance. We propose a united framework to encourage existing segmentation networks to improve breast tumor segmentation by prior shape information. The source code will be made available at https://github.com/TxLin7/Shape-Seg.
更多查看译文
关键词
breast tumor segmentation,deep convolution networks,prior shape information,superpixel,attention mechanism
AI 理解论文
溯源树
样例
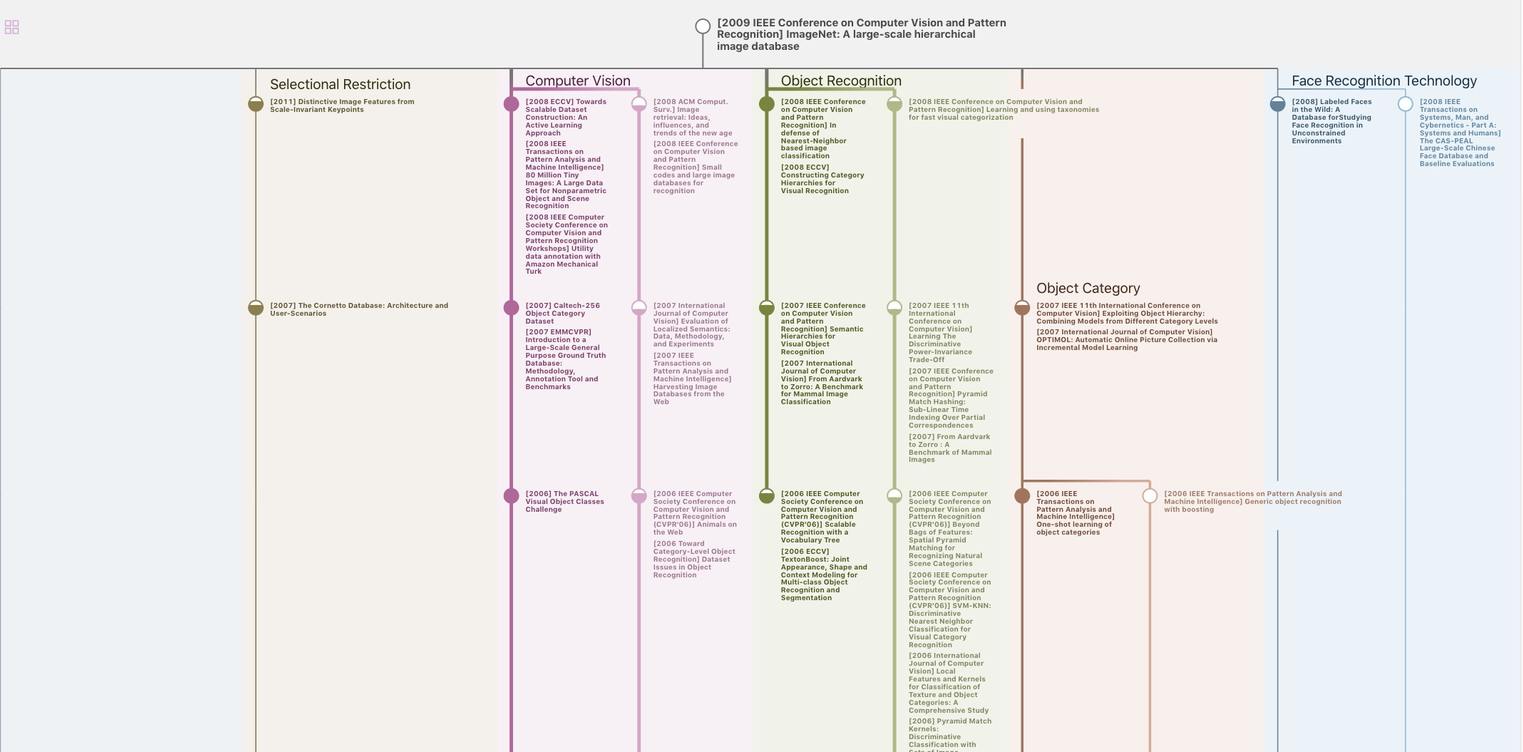
生成溯源树,研究论文发展脉络
Chat Paper
正在生成论文摘要