Editorial: Graph representation learning in biological network.
Frontiers in bioinformatics(2023)
摘要
Despite the recent introduction of such methods, the increasing interest of the scientific community has consolidated these approaches. Consequently, there are many applications of graph representation learning to model and analyze massive and multimodal biological data generated from high throughput omics technology, epidemiological data, and electronic health records. These approaches share the common idea of encoding network structure, represented as the adjacency matrix of the graph, into a latent lowdimensional space. Such methods are based on many algorithms, from matrix factorization to deep learning and complex non-linear models based on noneuclidean geometries. The relevance of these methods is the possibility to efficiently map in a low-dimensional space both topology and biology (i.e., all the available metadata for nodes and edges).We are delighted to announce the publication of a special issue on "Graph Representation Learning in Biological Networks" in Frontiers in Bioinformatics. This special issue aims to showcase the latest research and advancements in graph representation learning and its applications to biological networks. The issue contains high-quality manuscripts covering many different applications. The above high-quality manuscripts will significantly enrich the knowledge base of researchers already working in similar domains or the nascent research stage in graph representation learning.
更多查看译文
关键词
graph representation learning,network
AI 理解论文
溯源树
样例
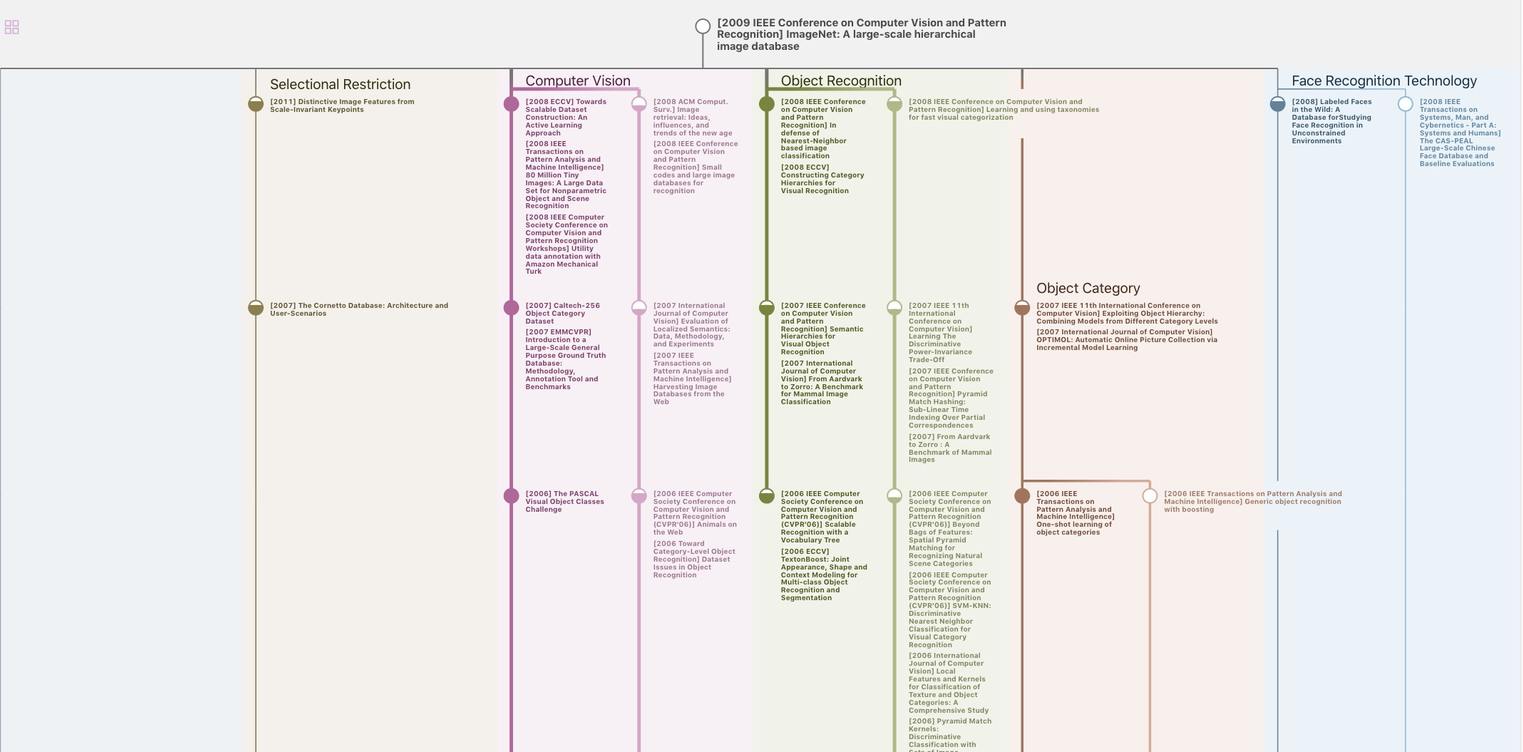
生成溯源树,研究论文发展脉络
Chat Paper
正在生成论文摘要