Detecting Paroxysmal Atrial Fibrillation From an Electrocardiogram in Sinus Rhythm: External Validation of the AI Approach.
JACC. Clinical electrophysiology(2023)
摘要
BACKGROUND:Atrial fibrillation (AF) may occur asymptomatically and can be diagnosed only with electrocardiography (ECG) while the arrhythmia is present.
OBJECTIVES:The aim of this study was to independently validate the approach of using artificial intelligence (AI) to identify underlying paroxysmal AF from a 12-lead ECG in sinus rhythm (SR).
METHODS:An AI algorithm was trained to identify patients with underlying paroxysmal AF, using electrocardiographic data from all in- and outpatients from a single center with at least 1 ECG in SR. For patients without AF, all ECGs in SR were included. For patients with AF, all ECGs in SR starting 31 days before the first AF event were included. The patients were randomly allocated to training, internal validation, and testing datasets in a 7:1:2 ratio. In a secondary analysis, the AF prevalence of the testing group was modified. Additionally, the performance of the algorithm was validated at an external hospital.
RESULTS:The dataset consisted of 494,042 ECGs in SR from 142,310 patients. Testing the model on the first ECG of each patient (AF prevalence 9.0%) resulted in accuracy of 78.1% (95% CI: 77.6%-78.5%), area under the receiver-operating characteristic curve of 0.87 (95% CI: 0.86-0.87), and area under the precision recall curve (AUPRC) of 0.48 (95% CI: 0.46-0.50). In a low-risk group (AF prevalence 3%), the AUPRC decreased to 0.21 (95% CI: 0.18-0.24). In a high-risk group (AF prevalence 30%), the AUPRC increased to 0.76 (95% CI: 0.75-0.78). This performance was robust when validated in an external hospital.
CONCLUSIONS:The approach of using an AI-enabled electrocardiographic algorithm for the identification of patients with underlying paroxysmal AF from ECGs in SR was independently validated.
更多查看译文
AI 理解论文
溯源树
样例
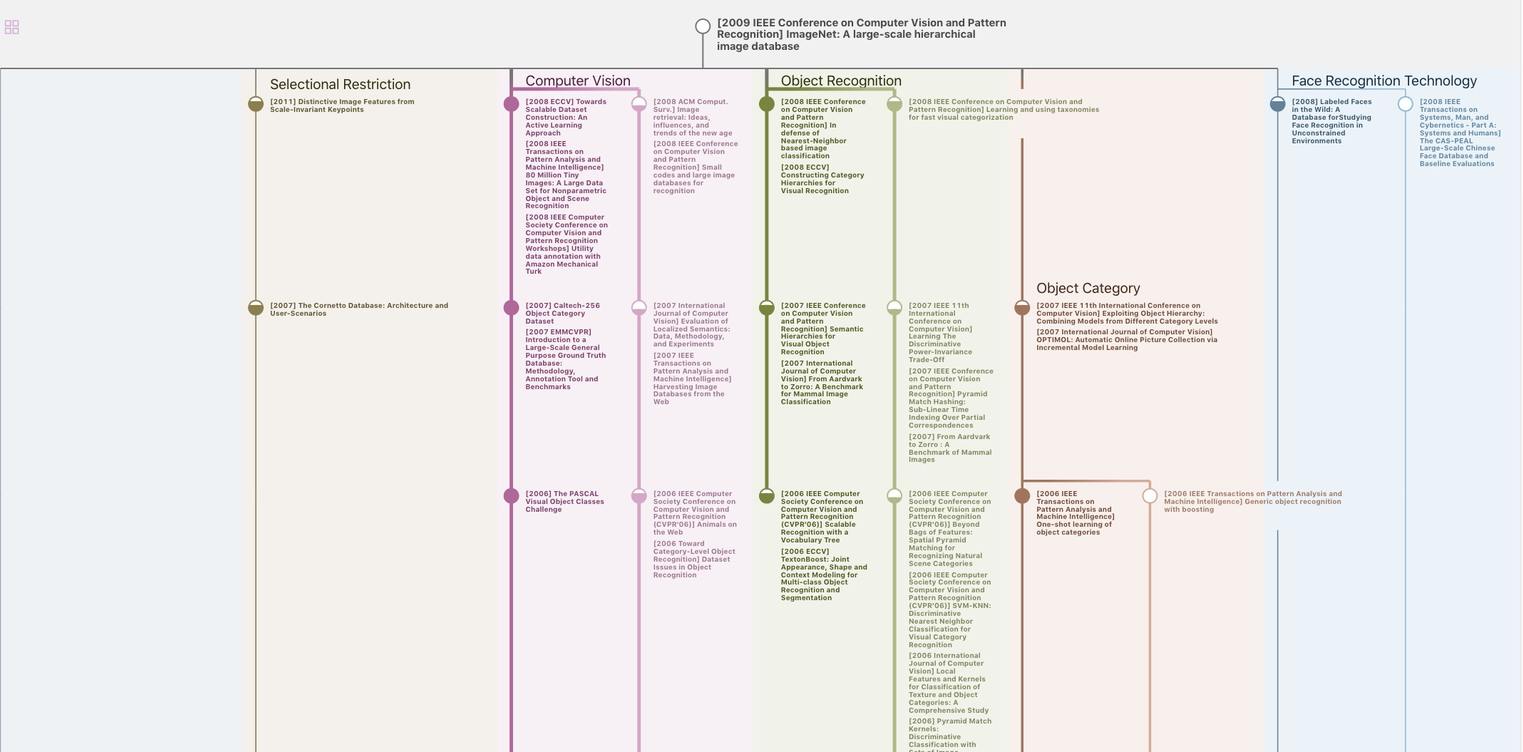
生成溯源树,研究论文发展脉络
Chat Paper
正在生成论文摘要