Using modular connectome-based predictive modeling to reveal brain-behavior relationships of individual differences in working memory
Brain structure & function(2023)
摘要
Working memory plays a crucial role in our daily lives, and brain imaging has been used to predict working memory performance. Here, we present an improved connectome-based predictive modeling approach for building a predictive model of individual working memory performance from whole-brain functional connectivity. The model was built using n-back task-based fMRI and resting-state fMRI data from the Human Connectome Project. Compared to prior models, our model was more interpretable, demonstrated a closer connection to the known anatomical and functional network. The model also demonstrates strong generalization on nine other cognitive behaviors from the HCP database and can well predict the working memory performance of healthy individuals in external datasets. By comparing the differences in prediction effects of different brain networks and anatomical feature analysis on n-back tasks, we found the essential role of some networks in differentiating between high and low working memory loads conditions.
更多查看译文
关键词
Brain-behavior prediction, Connectome-based predictive modeling, Functional connectivity, Feature selection, Working memory
AI 理解论文
溯源树
样例
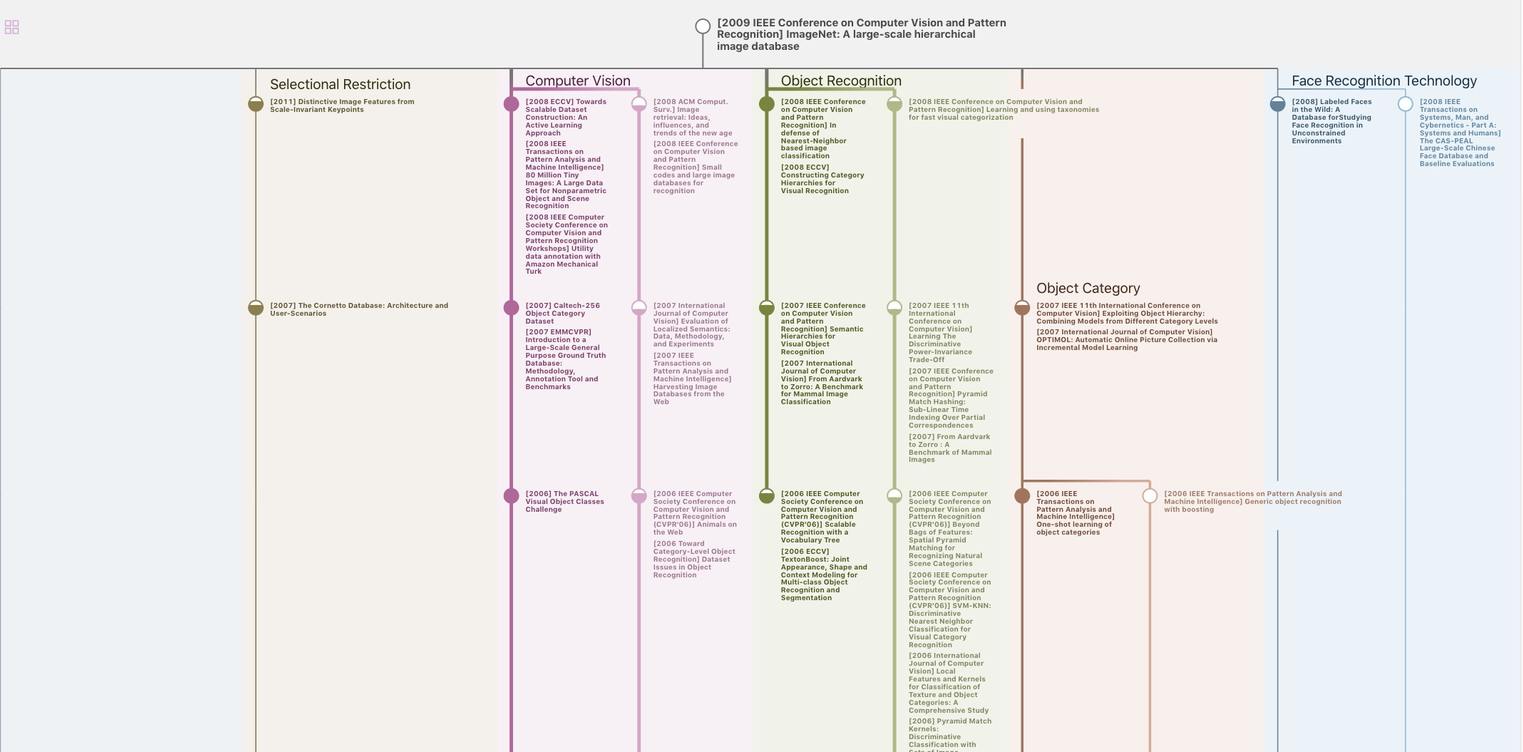
生成溯源树,研究论文发展脉络
Chat Paper
正在生成论文摘要