Hg and As pollution in the soil-plant system evaluated by combining multispectral UAV-RS, geochemical survey and machine learning.
Environmental pollution (Barking, Essex : 1987)(2023)
摘要
The combination of a low-density geochemical survey, multispectral data obtained with Unmanned Aerial Vehicle-Remote Sensing (UAV-RS), and a machine learning technique was tested in the search for a statistically robust prediction of contaminant distribution in soil and vegetation, for zones with a highly variable pollutant load. To this end, a novel methodology was devised by means of a limited geochemical study of topsoil and vegetation combined with multispectral data obtained by UAV-RS. The methodology was verified in an area affected by Hg and As contamination that typifies abandoned mining-metallurgy sites in recent decades. A broad selection of spectral indices were calculated to evaluate soil-plant system response, and four machine learning techniques (Multiple Linear Regression, Random Forest, Generalized Boosted Models, and Multivariate Adaptive Regression Spline) were tested to obtain robust statistical models. Random Forest (RF) provided the best non-biased models for As and Hg concentration in soil and vegetation, with R2 and rRMSE (%) ranging from 0.501 to 0.630 and from 180.72 to 46.31, respectively, and with acceptable values for RPD and RPIQ statistics. The prediction and mapping of contaminant content and distribution in the study area were well enough adjusted to the geochemical data and revealed superior accuracy for As than Hg, and for vegetation than topsoil. The results were more precise than those obtained in comparable studies that applied satellite or spectrometry data. In conclusion, the methodology presented emerges as a powerful tool for studies addressing soil and vegetation pollution and an alternative approach to classical geochemical studies, which are time-consuming and expensive.
更多查看译文
AI 理解论文
溯源树
样例
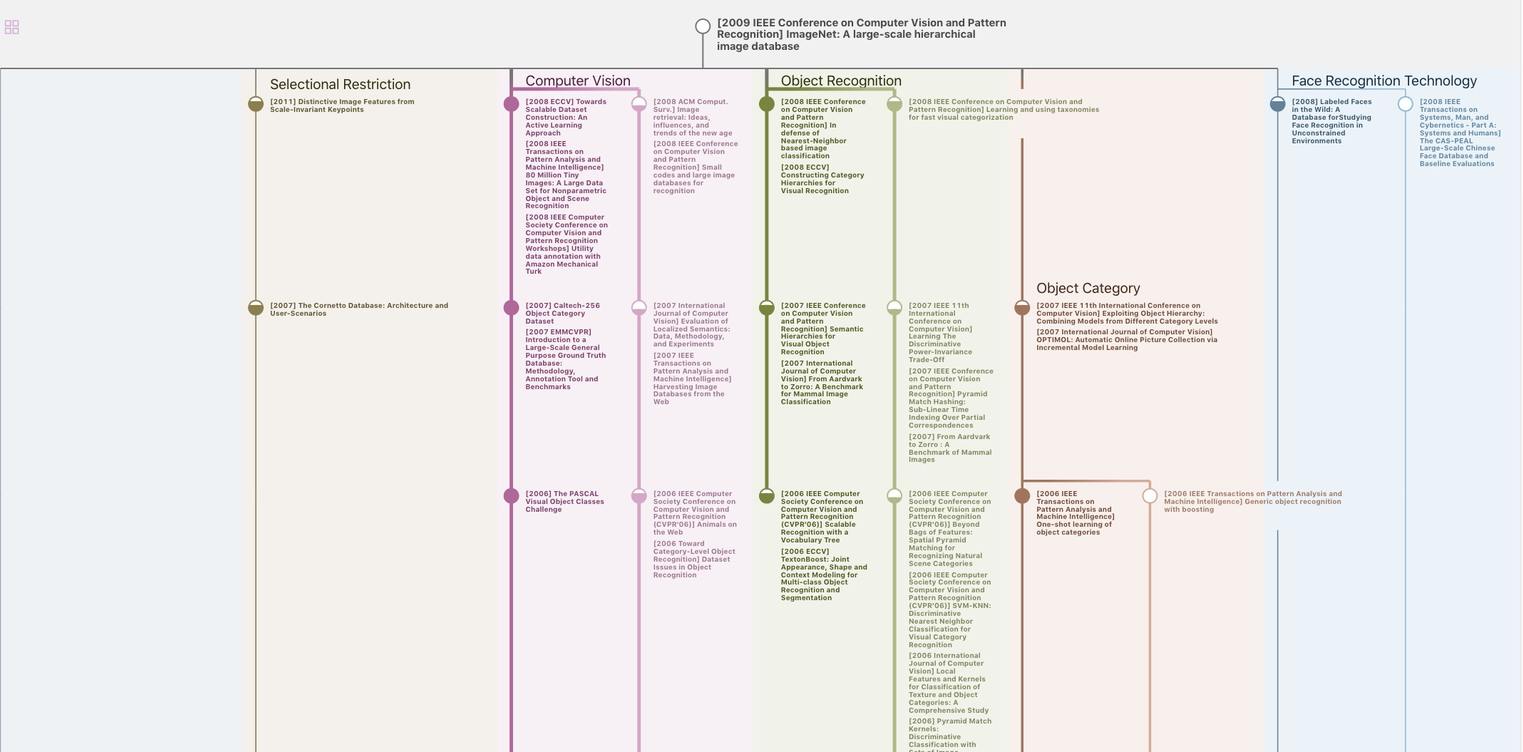
生成溯源树,研究论文发展脉络
Chat Paper
正在生成论文摘要