Performances and variability of CT radiomics for the prediction of microvascular invasion and survival in patients with HCC: a matter of chance or standardisation?
European radiology(2023)
摘要
Objectives To measure the performance and variability of a radiomics-based model for the prediction of microvascular invasion (MVI) and survival in patients with resected hepatocellular carcinoma (HCC), simulating its sequential development and application. Methods This study included 230 patients with 242 surgically resected HCCs who underwent preoperative CT, of which 73/230 (31.7%) were scanned in external centres. The study cohort was split into training set (158 patients, 165 HCCs) and held-out test set (72 patients, 77 HCCs), stratified by random partitioning, which was repeated 100 times, and by a temporal partitioning to simulate the sequential development and clinical use of the radiomics model. A machine learning model for the prediction of MVI was developed with least absolute shrinkage and selection operator (LASSO). The concordance index (C-index) was used to assess the value to predict the recurrence-free (RFS) and overall survivals (OS). Results In the 100-repetition random partitioning cohorts, the radiomics model demonstrated a mean AUC of 0.54 (range 0.44–0.68) for the prediction of MVI, mean C-index of 0.59 (range 0.44–0.73) for RFS, and 0.65 (range 0.46–0.86) for OS in the held-out test set. In the temporal partitioning cohort, the radiomics model yielded an AUC of 0.50 for the prediction of MVI, a C-index of 0.61 for RFS, and 0.61 for OS, in the held-out test set. Conclusions The radiomics models had a poor performance for the prediction of MVI with a large variability in the model performance depending on the random partitioning. Radiomics models demonstrated good performance in the prediction of patient outcomes. Clinical relevance statement Patient selection within the training set strongly influenced the performance of the radiomics models for predicting microvascular invasion; therefore, a random approach to partitioning a retrospective cohort into a training set and a held-out set seems inappropriate. Key Points • The performance of the radiomics models for the prediction of microvascular invasion and survival widely ranged (AUC range 0.44–0.68) in the randomly partitioned cohorts. • The radiomics model for the prediction of microvascular invasion was unsatisfying when trying to simulate its sequential development and clinical use in a temporal partitioned cohort imaged with a variety of CT scanners. • The performance of the radiomics models for the prediction of survival was good with similar performances in the 100-repetition random partitioning and temporal partitioning cohorts.
更多查看译文
关键词
Artificial intelligence, Liver neoplasms, Carcinoma, hepatocellular, Machine learning, Survival
AI 理解论文
溯源树
样例
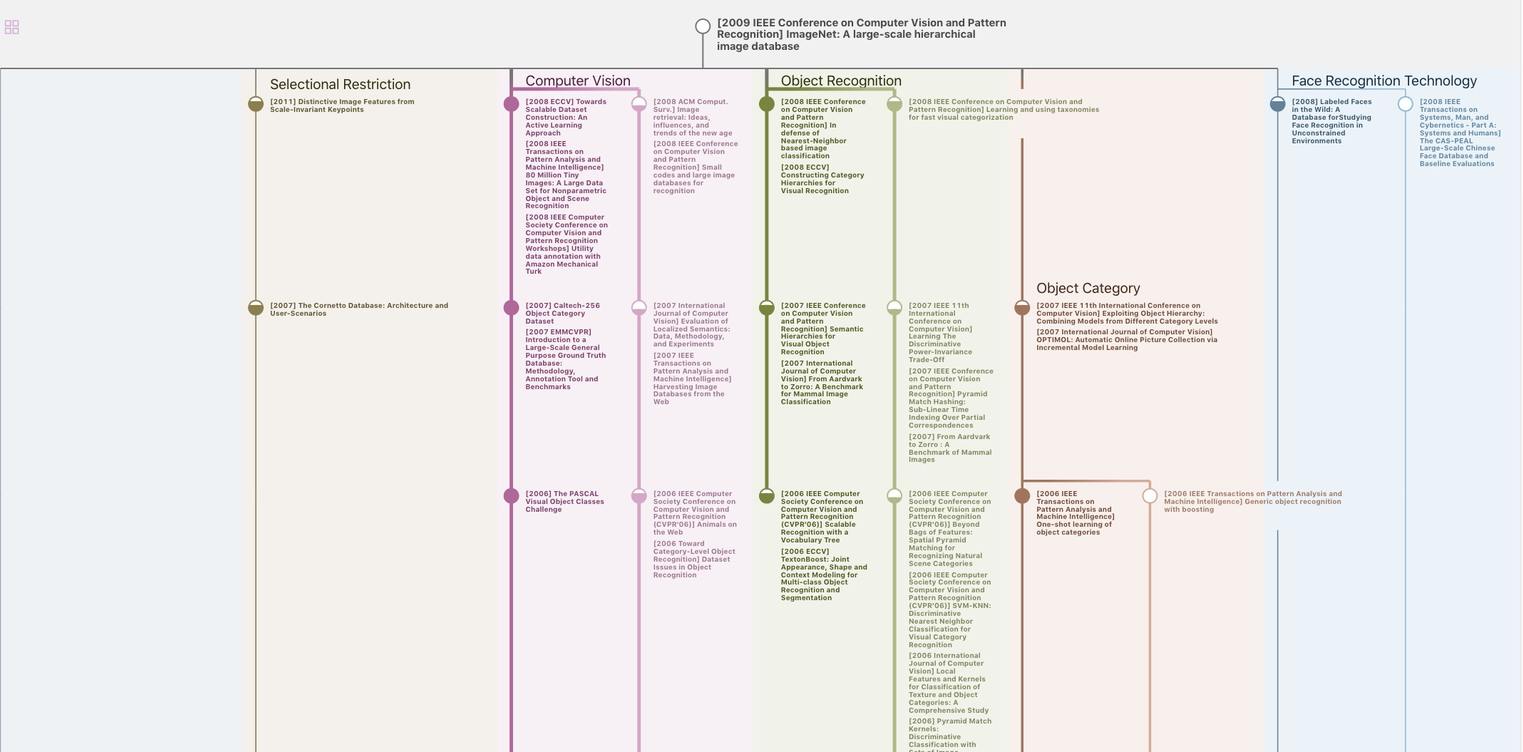
生成溯源树,研究论文发展脉络
Chat Paper
正在生成论文摘要