Adaptive Prototype Interaction Network for Few-Shot Knowledge Graph Completion.
IEEE transactions on neural networks and learning systems(2023)
摘要
Few-shot knowledge graph completion (FKGC), which aims to infer new triples for a relation using only a few reference triples of the relation, has attracted much attention in recent years. Most existing FKGC methods learn a transferable embedding space, where entity pairs belonging to the same relations are close to each other. In real-world knowledge graphs (KGs), however, some relations may involve multiple semantics, and their entity pairs are not always close due to having different meanings. Hence, the existing FKGC methods may yield suboptimal performance when handling multiple semantic relations in the few-shot scenario. To solve this problem, we propose a new method named adaptive prototype interaction network (APINet) for FKGC. Our model consists of two major components: 1) an interaction attention encoder (InterAE) to capture the underlying relational semantics of entity pairs by modeling the interactive information between head and tail entities and 2) an adaptive prototype net (APNet) to generate relation prototypes adaptive to different query triples by extracting query-relevant reference pairs and reducing the data inconsistency between support and query sets. Experimental results on two public datasets demonstrate that APINet outperforms several state-of-the-art FKGC methods. The ablation study demonstrates the rationality and effectiveness of each component of APINet.
更多查看译文
关键词
Adaptive prototype,few-shot knowledge graph completion (FKGC),multiple semantic relations
AI 理解论文
溯源树
样例
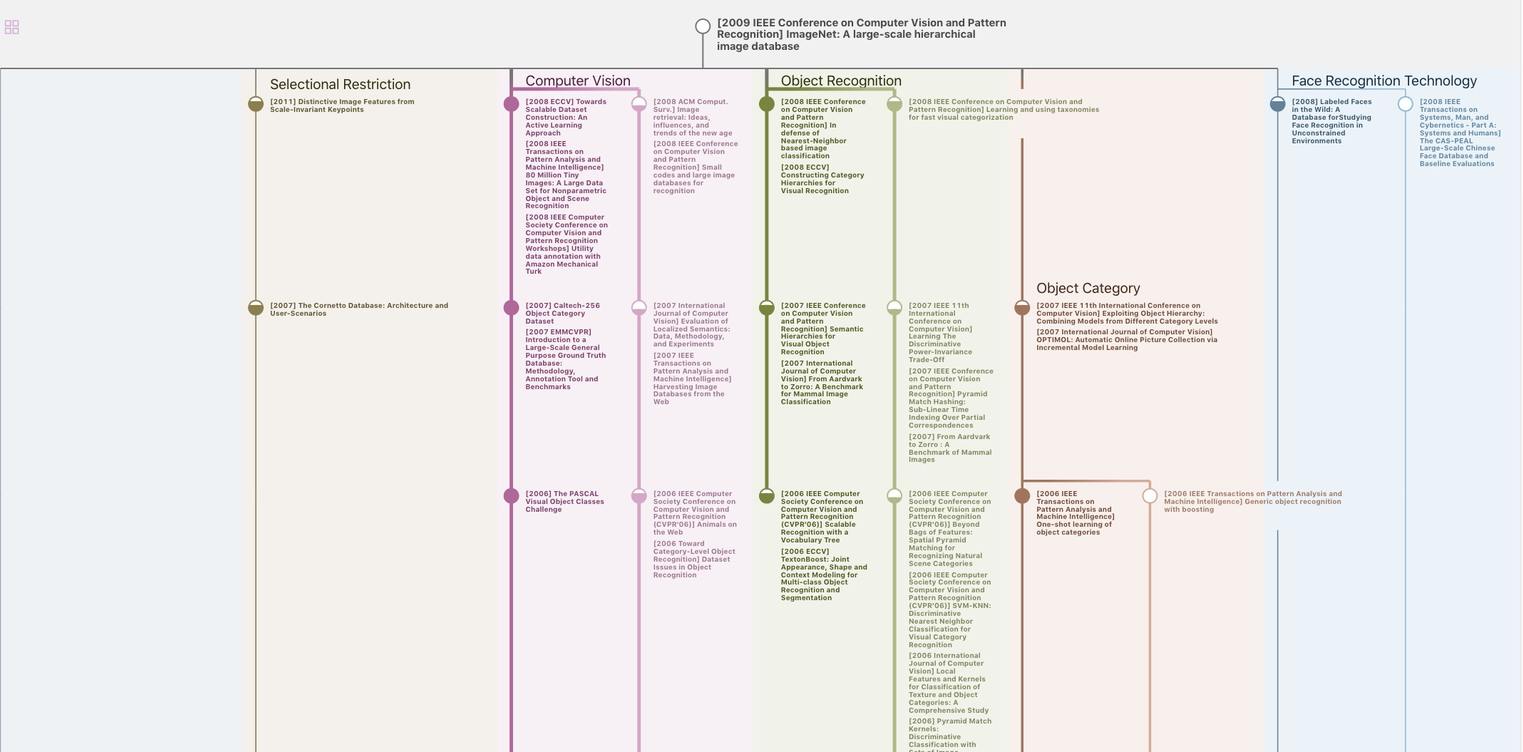
生成溯源树,研究论文发展脉络
Chat Paper
正在生成论文摘要