Exploring the added value of pretherapeutic MR descriptors in predicting breast cancer pathologic complete response to neoadjuvant chemotherapy
European radiology(2023)
摘要
Objectives To evaluate the association between pretreatment MRI descriptors and breast cancer (BC) pathological complete response (pCR) to neoadjuvant chemotherapy (NAC). Materials and methods Patients with BC treated by NAC with a breast MRI between 2016 and 2020 were included in this retrospective observational single-center study. MR studies were described using the standardized BI-RADS and breast edema score on T2-weighted MRI. Univariable and multivariable logistic regression analyses were performed to assess variables association with pCR according to residual cancer burden. Random forest classifiers were trained to predict pCR on a random split including 70% of the database and were validated on the remaining cases. Results Among 129 BC, 59 (46%) achieved pCR after NAC (luminal ( n = 7/37, 19%), triple negative ( n = 30/55, 55%), HER2 + ( n = 22/37, 59%)). Clinical and biological items associated with pCR were BC subtype ( p < 0.001), T stage 0/I/II ( p = 0.008), higher Ki67 ( p = 0.005), and higher tumor-infiltrating lymphocytes levels ( p = 0.016). Univariate analysis showed that the following MRI features, oval or round shape ( p = 0.047), unifocality ( p = 0.026), non-spiculated margins ( p = 0.018), no associated non-mass enhancement ( p = 0.024), and a lower MRI size ( p = 0.031), were significantly associated with pCR. Unifocality and non-spiculated margins remained independently associated with pCR at multivariable analysis. Adding significant MRI features to clinicobiological variables in random forest classifiers significantly increased sensitivity (0.67 versus 0.62), specificity (0.69 versus 0.67), and precision (0.71 versus 0.67) for pCR prediction. Conclusion Non-spiculated margins and unifocality are independently associated with pCR and can increase models performance to predict BC response to NAC. Clinical relevance statement A multimodal approach integrating pretreatment MRI features with clinicobiological predictors, including tumor-infiltrating lymphocytes, could be employed to develop machine learning models for identifying patients at risk of non-response. This may enable consideration of alternative therapeutic strategies to optimize treatment outcomes. Key Points • Unifocality and non-spiculated margins are independently associated with pCR at multivariable logistic regression analysis. • Breast edema score is associated with MR tumor size and TIL expression, not only in TN BC as previously reported, but also in luminal BC. • Adding significant MRI features to clinicobiological variables in machine learning classifiers significantly increased sensitivity, specificity, and precision for pCR prediction.
更多查看译文
关键词
Breast neoplasms,Neoadjuvant therapy,Magnetic resonance imaging,Neoplasm, residual,Treatment outcome
AI 理解论文
溯源树
样例
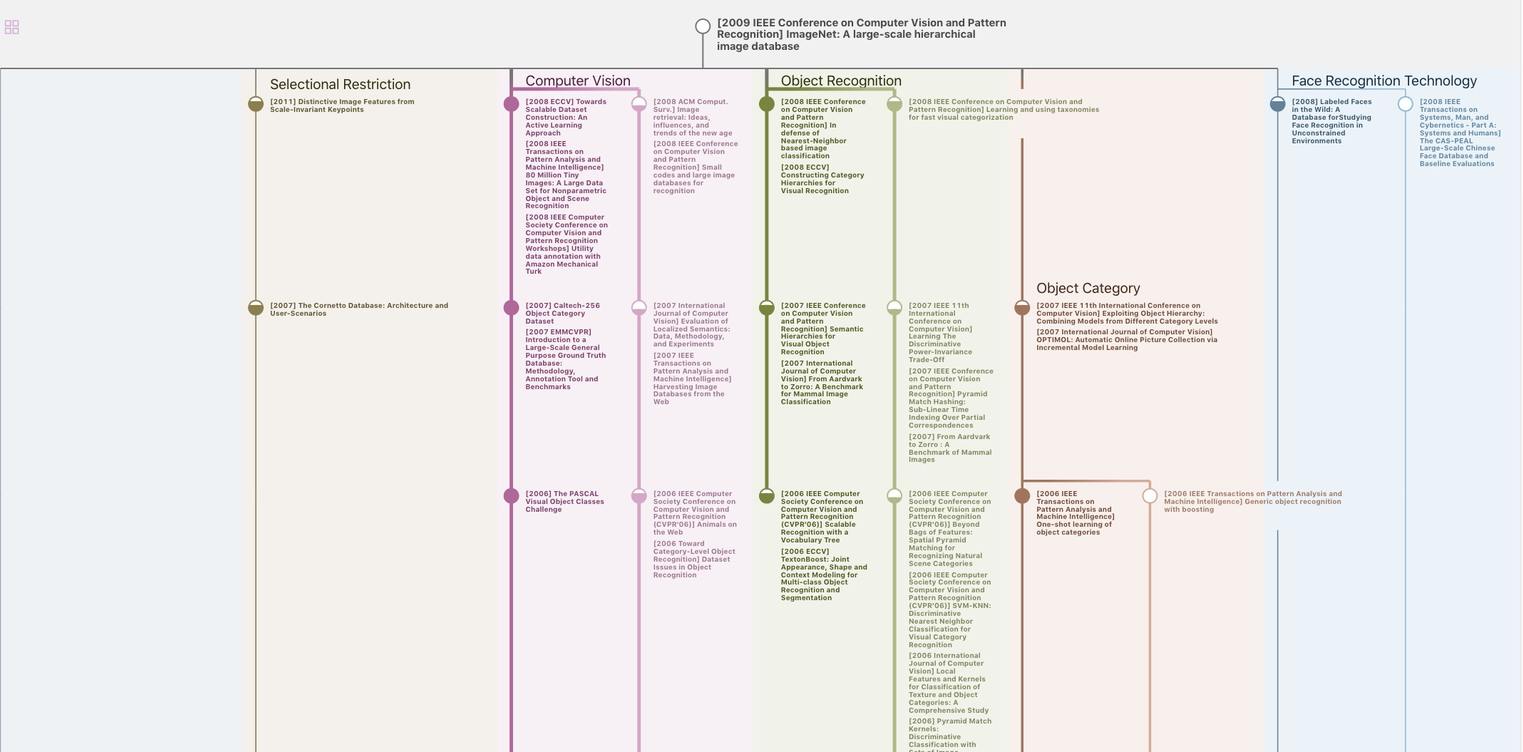
生成溯源树,研究论文发展脉络
Chat Paper
正在生成论文摘要